TriggerNER: Learning with Entity Triggers as Explanations for Named Entity Recognition
Bill Yuchen Lin, Dong-Ho Lee, Ming Shen, Ryan Moreno, Xiao Huang, Prashant Shiralkar, Xiang Ren
Information Extraction Short Paper
Session 14B: Jul 8
(18:00-19:00 GMT)
Session 15B: Jul 8
(21:00-22:00 GMT)
Abstract:
Training neural models for named entity recognition (NER) in a new domain often requires additional human annotations (e.g., tens of thousands of labeled instances) that are usually expensive and time-consuming to collect. Thus, a crucial research question is how to obtain supervision in a cost-effective way. In this paper, we introduce "entity triggers," an effective proxy of human explanations for facilitating label-efficient learning of NER models. An entity trigger is defined as a group of words in a sentence that helps to explain why humans would recognize an entity in the sentence. We crowd-sourced 14k entity triggers for two well-studied NER datasets. Our proposed model, Trigger Matching Network, jointly learns trigger representations and soft matching module with self-attention such that can generalize to unseen sentences easily for tagging. Our framework is significantly more cost-effective than the traditional neural NER frameworks. Experiments show that using only 20% of the trigger-annotated sentences results in a comparable performance as using 70% of conventional annotated sentences.
You can open the
pre-recorded video
in a separate window.
NOTE: The SlidesLive video may display a random order of the authors.
The correct author list is shown at the top of this webpage.
Similar Papers
A Unified MRC Framework for Named Entity Recognition
Xiaoya Li, Jingrong Feng, Yuxian Meng, Qinghong Han, Fei Wu, Jiwei Li,
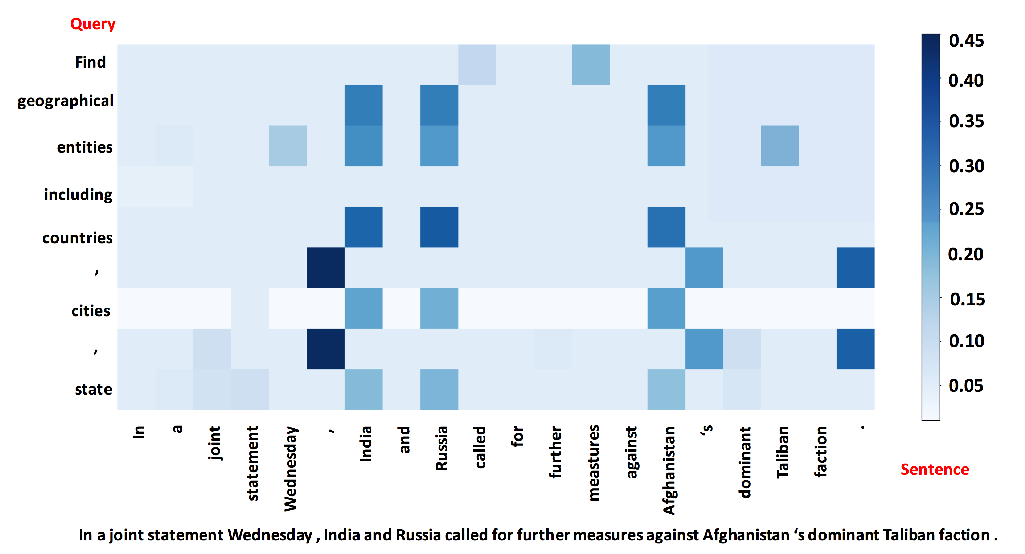
Simplify the Usage of Lexicon in Chinese NER
Ruotian Ma, Minlong Peng, Qi Zhang, Zhongyu Wei, Xuanjing Huang,
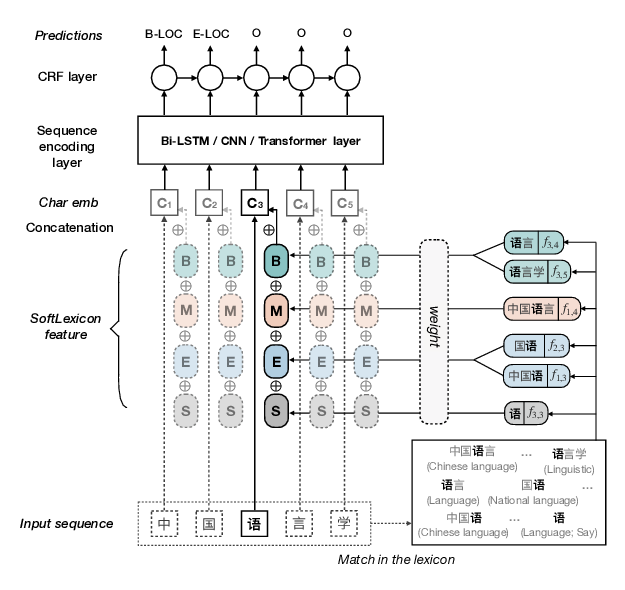
Named Entity Recognition without Labelled Data: A Weak Supervision Approach
Pierre Lison, Jeremy Barnes, Aliaksandr Hubin, Samia Touileb,
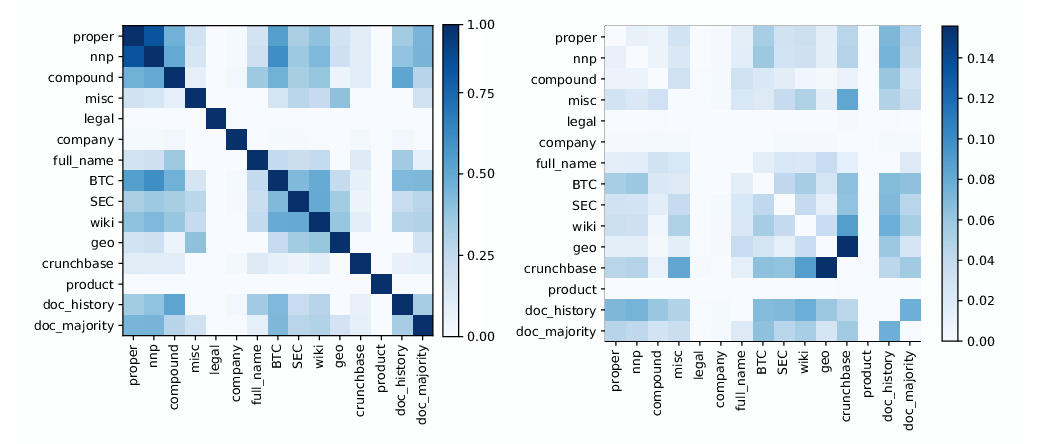
Improving Event Detection via Open-domain Trigger Knowledge
Meihan Tong, Bin Xu, Shuai Wang, Yixin Cao, Lei Hou, Juanzi Li, Jun Xie,
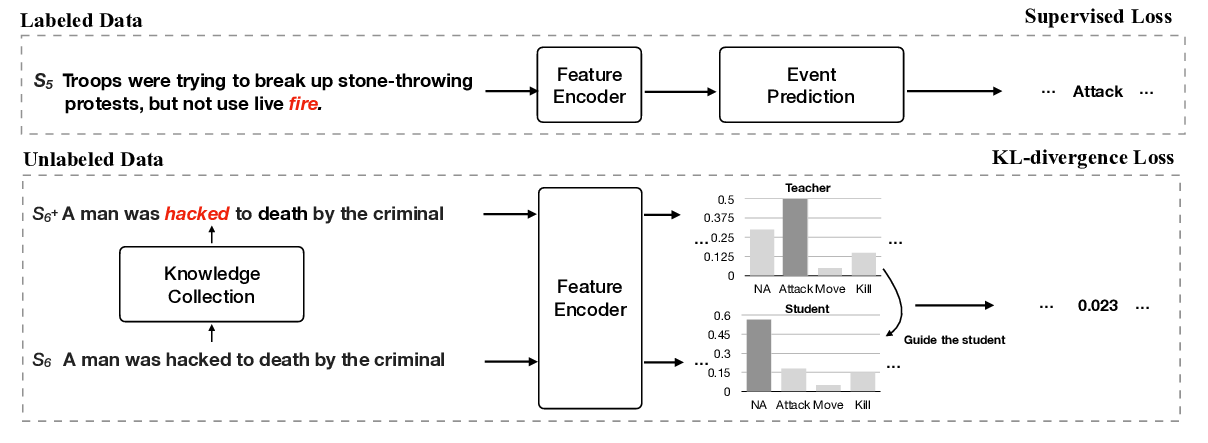