Evaluating Robustness to Input Perturbations for Neural Machine Translation
Xing Niu, Prashant Mathur, Georgiana Dinu, Yaser Al-Onaizan
Machine Translation Short Paper
Session 14B: Jul 8
(18:00-19:00 GMT)
Session 15A: Jul 8
(20:00-21:00 GMT)
Abstract:
Neural Machine Translation (NMT) models are sensitive to small perturbations in the input. Robustness to such perturbations is typically measured using translation quality metrics such as BLEU on the noisy input. This paper proposes additional metrics which measure the relative degradation and changes in translation when small perturbations are added to the input. We focus on a class of models employing subword regularization to address robustness and perform extensive evaluations of these models using the robustness measures proposed. Results show that our proposed metrics reveal a clear trend of improved robustness to perturbations when subword regularization methods are used.
You can open the
pre-recorded video
in a separate window.
NOTE: The SlidesLive video may display a random order of the authors.
The correct author list is shown at the top of this webpage.
Similar Papers
Word-level Textual Adversarial Attacking as Combinatorial Optimization
Yuan Zang, Fanchao Qi, Chenghao Yang, Zhiyuan Liu, Meng Zhang, Qun Liu, Maosong Sun,
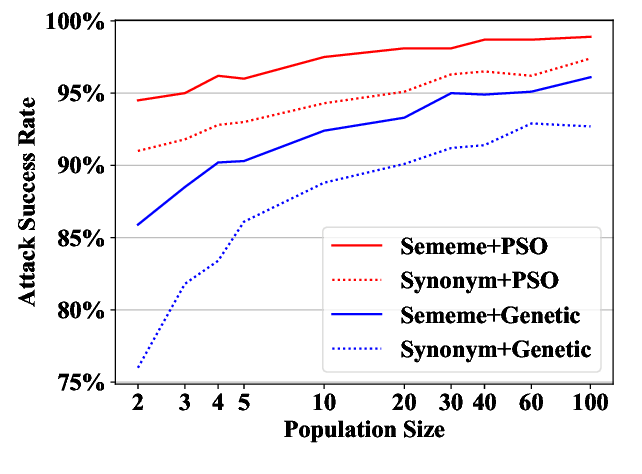
Robust Encodings: A Framework for Combating Adversarial Typos
Erik Jones, Robin Jia, Aditi Raghunathan, Percy Liang,
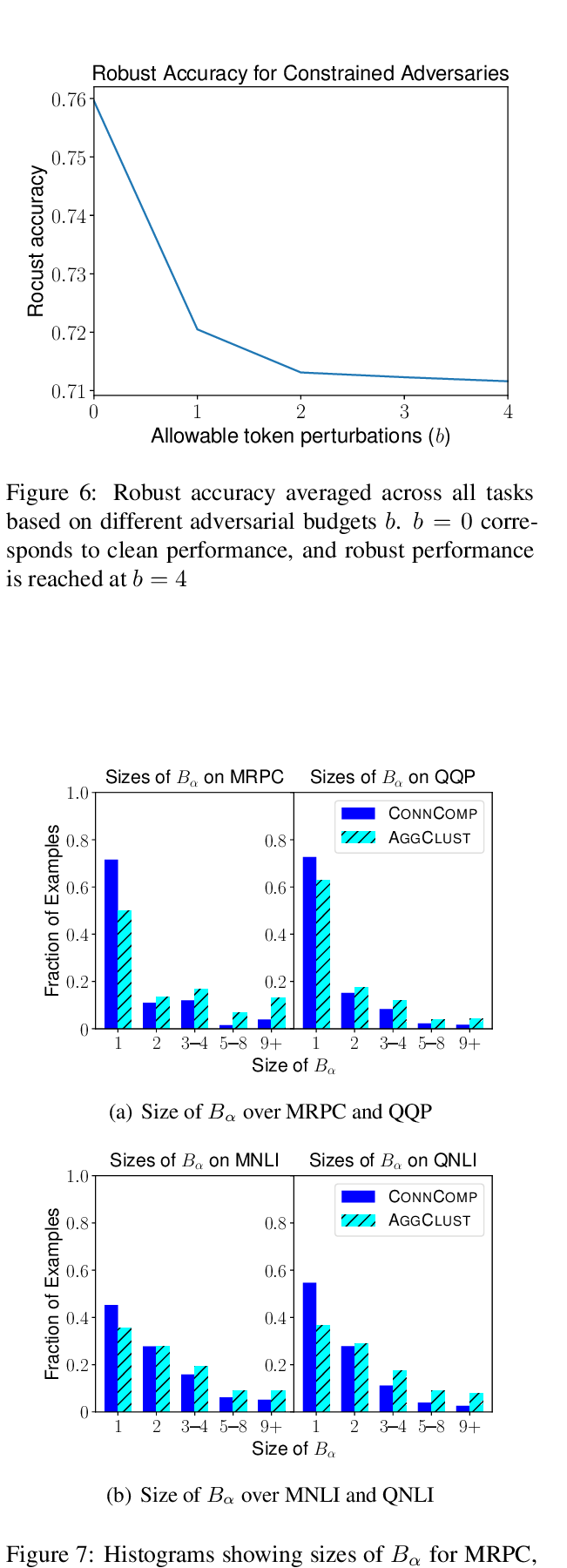
SAFER: A Structure-free Approach for Certified Robustness to Adversarial Word Substitutions
Mao Ye, Chengyue Gong, Qiang Liu,
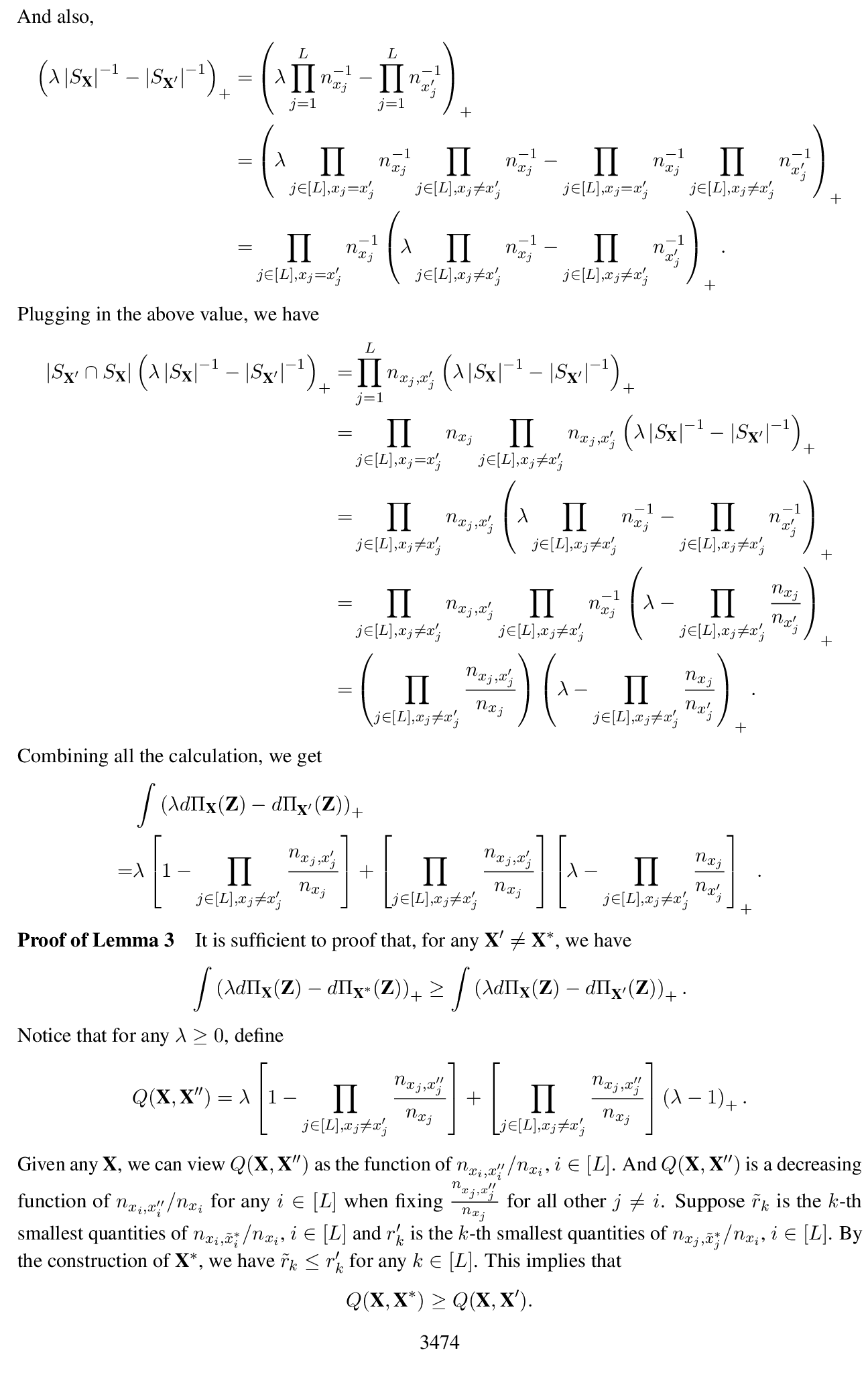
NAT: Noise-Aware Training for Robust Neural Sequence Labeling
Marcin Namysl, Sven Behnke, Joachim Köhler,
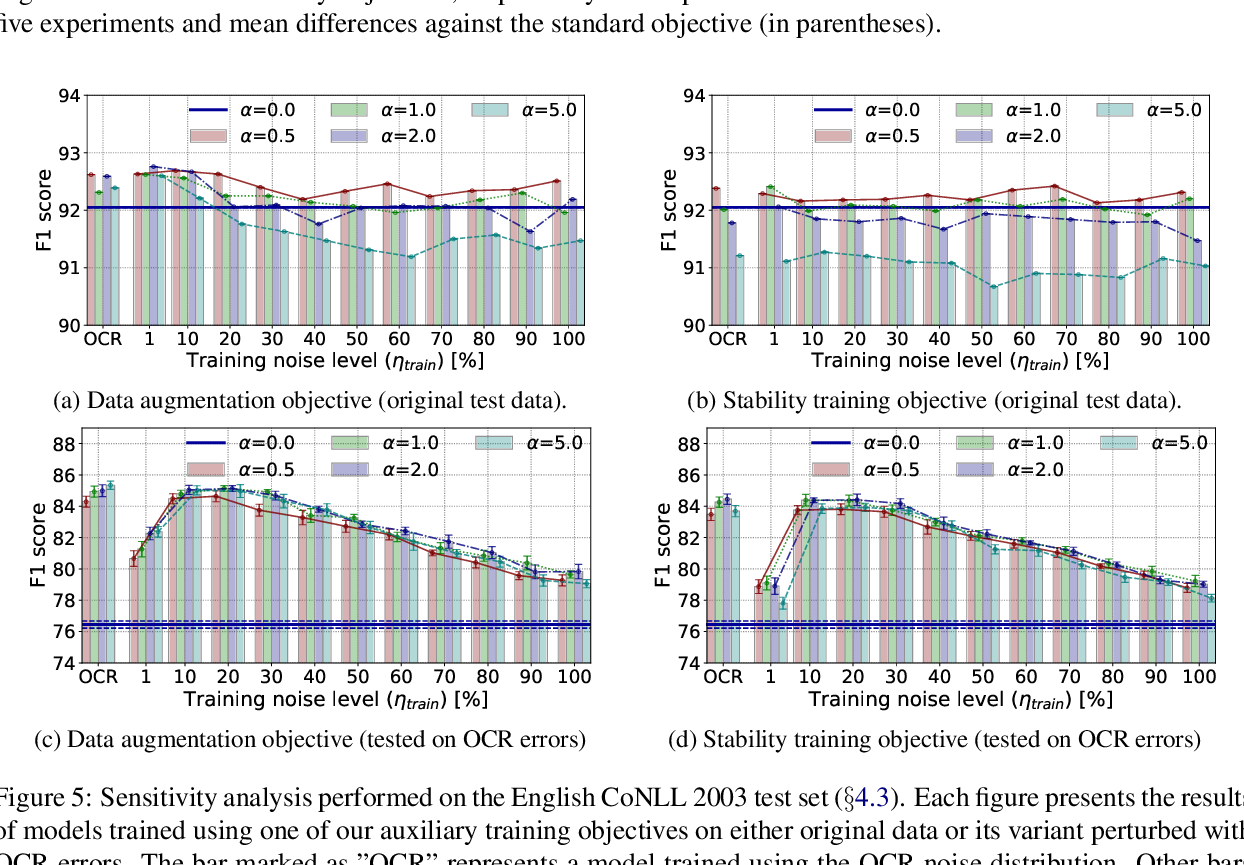