SeqVAT: Virtual Adversarial Training for Semi-Supervised Sequence Labeling
Luoxin Chen, Weitong Ruan, Xinyue Liu, Jianhua Lu
Syntax: Tagging, Chunking and Parsing Long Paper
Session 14B: Jul 8
(18:00-19:00 GMT)
Session 15B: Jul 8
(21:00-22:00 GMT)
Abstract:
Virtual adversarial training (VAT) is a powerful technique to improve model robustness in both supervised and semi-supervised settings. It is effective and can be easily adopted on lots of image classification and text classification tasks. However, its benefits to sequence labeling tasks such as named entity recognition (NER) have not been shown as significant, mostly, because the previous approach can not combine VAT with the conditional random field (CRF). CRF can significantly boost accuracy for sequence models by putting constraints on label transitions, which makes it an essential component in most state-of-the-art sequence labeling model architectures. In this paper, we propose SeqVAT, a method which naturally applies VAT to sequence labeling models with CRF. Empirical studies show that SeqVAT not only significantly improves the sequence labeling performance over baselines under supervised settings, but also outperforms state-of-the-art approaches under semi-supervised settings.
You can open the
pre-recorded video
in a separate window.
NOTE: The SlidesLive video may display a random order of the authors.
The correct author list is shown at the top of this webpage.
Similar Papers
NAT: Noise-Aware Training for Robust Neural Sequence Labeling
Marcin Namysl, Sven Behnke, Joachim Köhler,
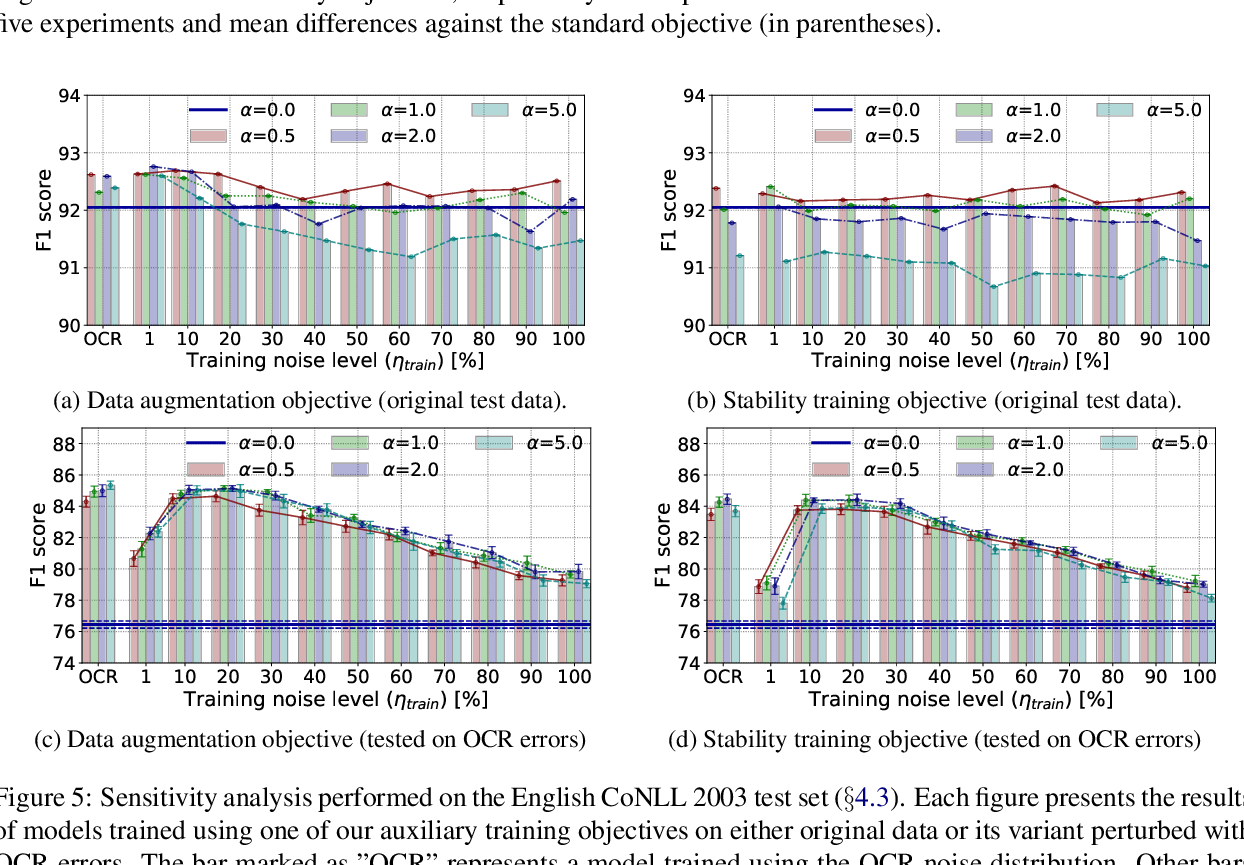
A Unified MRC Framework for Named Entity Recognition
Xiaoya Li, Jingrong Feng, Yuxian Meng, Qinghong Han, Fei Wu, Jiwei Li,
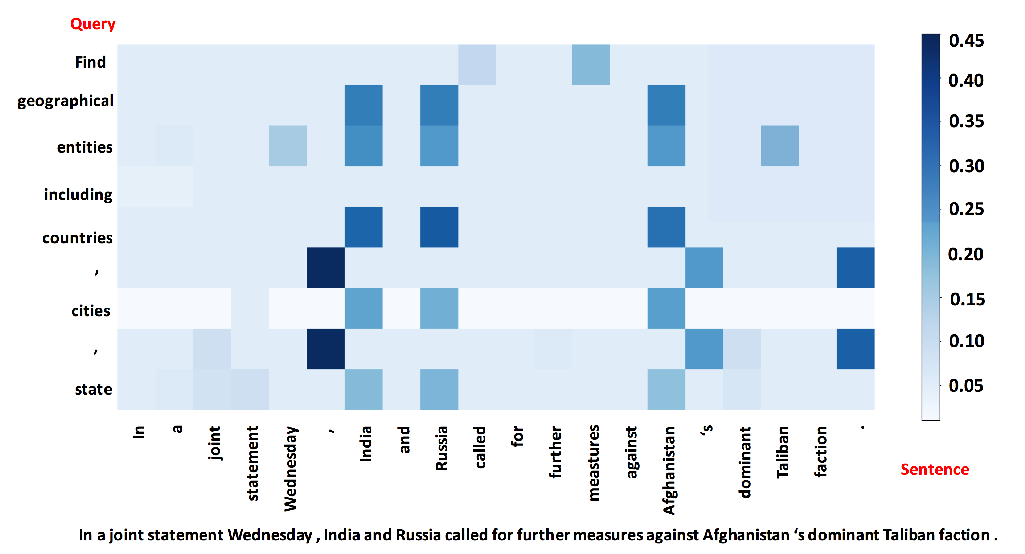
Embeddings of Label Components for Sequence Labeling: A Case Study of Fine-grained Named Entity Recognition
Takuma Kato, Kaori Abe, Hiroki Ouchi, Shumpei Miyawaki, Jun Suzuki, Kentaro Inui,
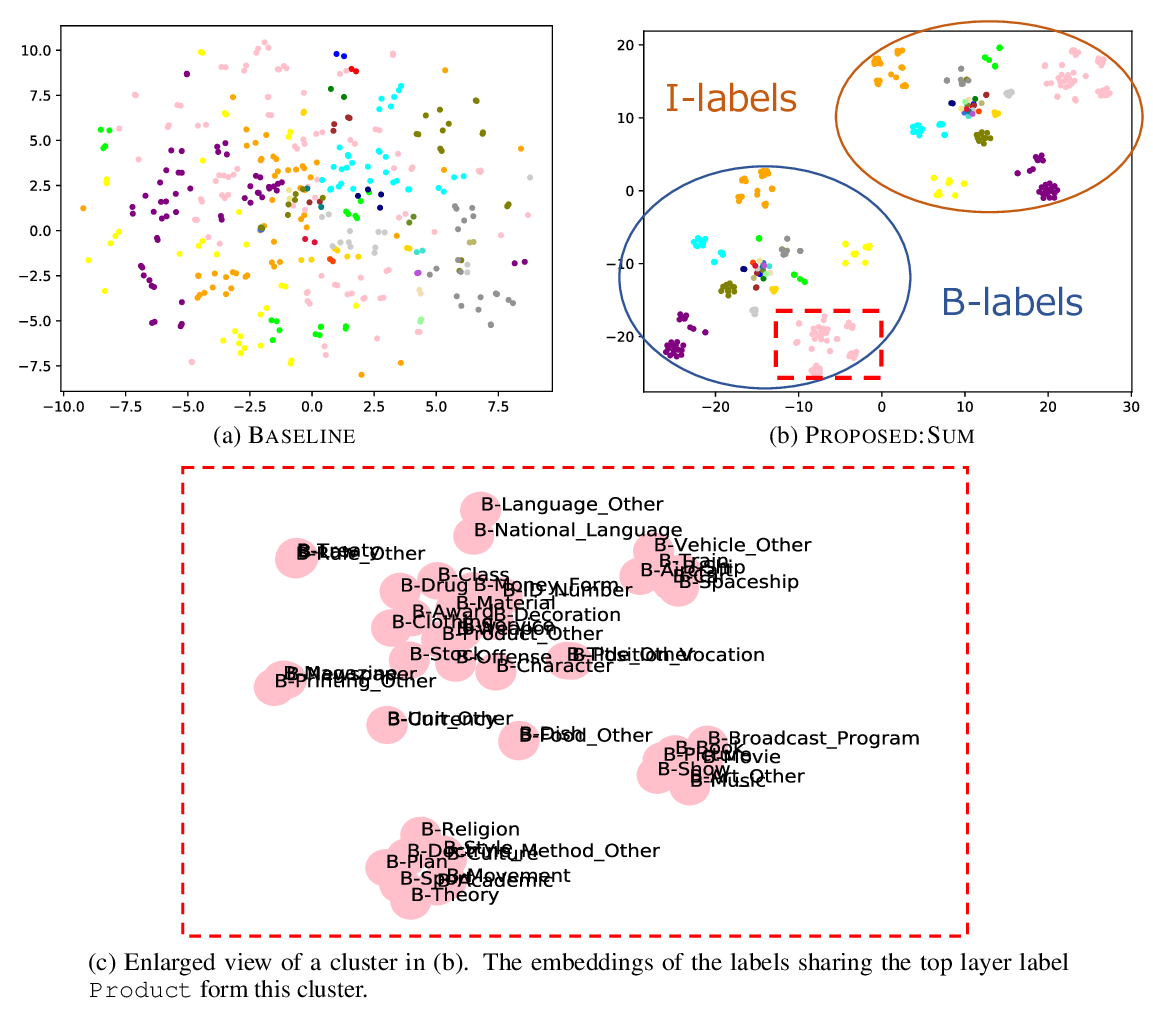
Cross-Lingual Semantic Role Labeling with High-Quality Translated Training Corpus
Hao Fei, Meishan Zhang, Donghong Ji,
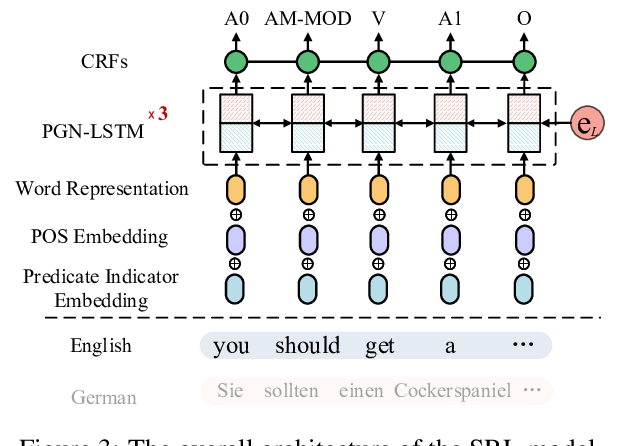