Interpreting Twitter User Geolocation
Ting Zhong, Tianliang Wang, Fan Zhou, Goce Trajcevski, Kunpeng Zhang, Yi Yang
NLP Applications Short Paper
Session 1B: Jul 6
(06:00-07:00 GMT)
Session 2B: Jul 6
(09:00-10:00 GMT)
Abstract:
Identifying user geolocation in online social networks is an essential task in many location-based applications. Existing methods rely on the similarity of text and network structure, however, they suffer from a lack of interpretability on the corresponding results, which is crucial for understanding model behavior. In this work, we adopt influence functions to interpret the behavior of GNN-based models by identifying the importance of training users when predicting the locations of the testing users. This methodology helps with providing meaningful explanations on prediction results. Furthermore, it also initiates an attempt to uncover the so-called "black-box" GNN-based models by investigating the effect of individual nodes.
You can open the
pre-recorded video
in a separate window.
NOTE: The SlidesLive video may display a random order of the authors.
The correct author list is shown at the top of this webpage.
Similar Papers
Generating Hierarchical Explanations on Text Classification via Feature Interaction Detection
Hanjie Chen, Guangtao Zheng, Yangfeng Ji,
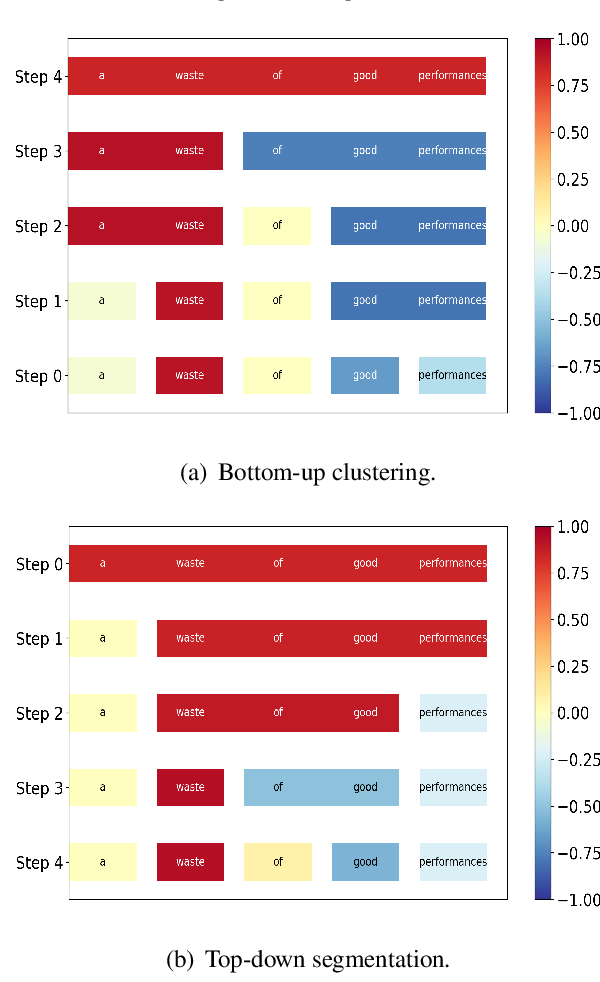
Explaining Black Box Predictions and Unveiling Data Artifacts through Influence Functions
Xiaochuang Han, Byron C. Wallace, Yulia Tsvetkov,
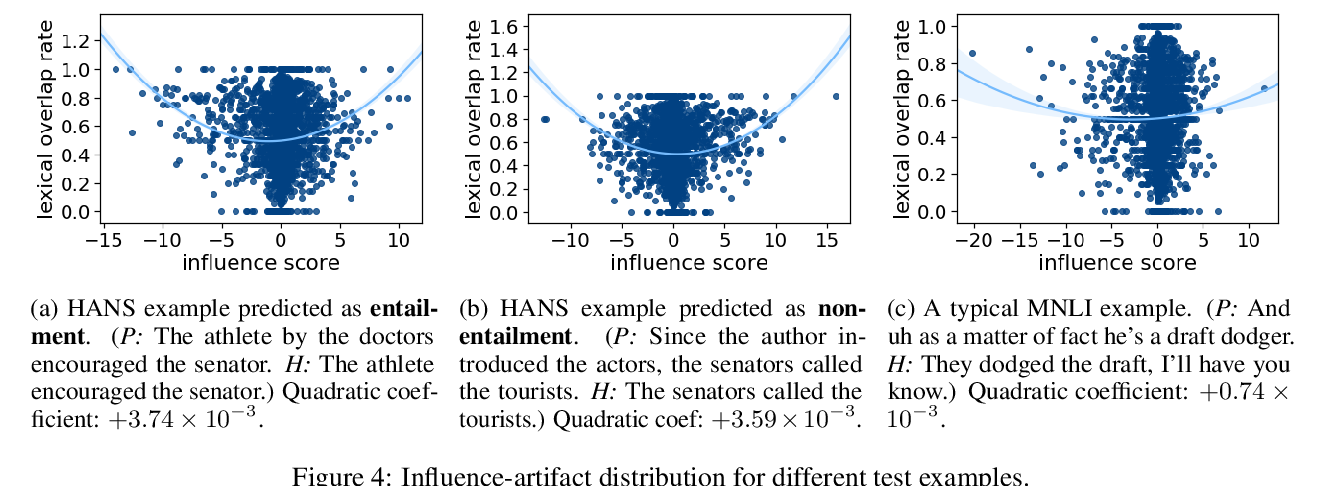
NILE : Natural Language Inference with Faithful Natural Language Explanations
Sawan Kumar, Partha Talukdar,
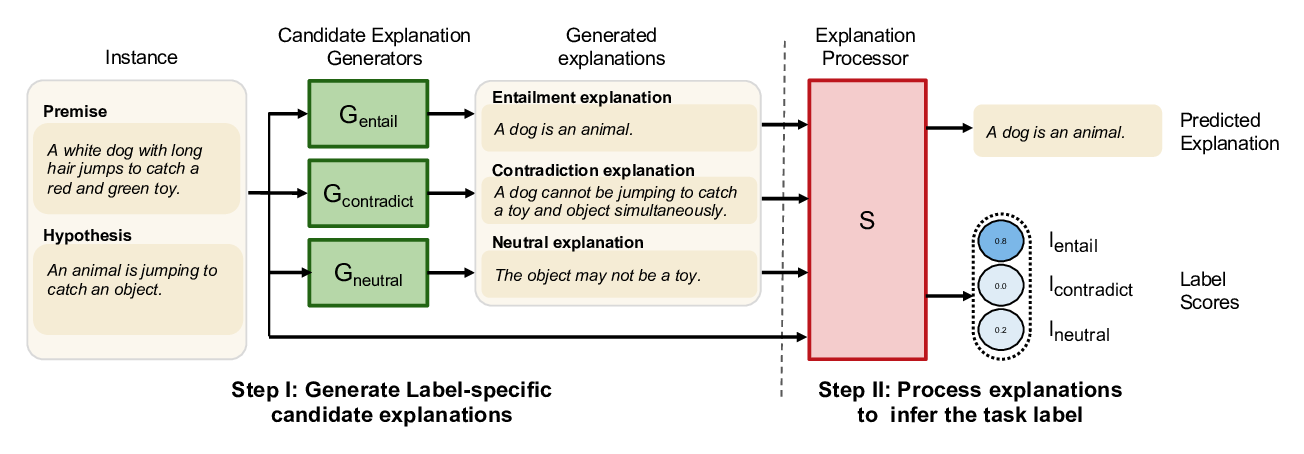
Towards Interpretable Clinical Diagnosis with Bayesian Network Ensembles Stacked on Entity-Aware CNNs
Jun Chen, Xiaoya Dai, Quan Yuan, Chao Lu, Haifeng Huang,
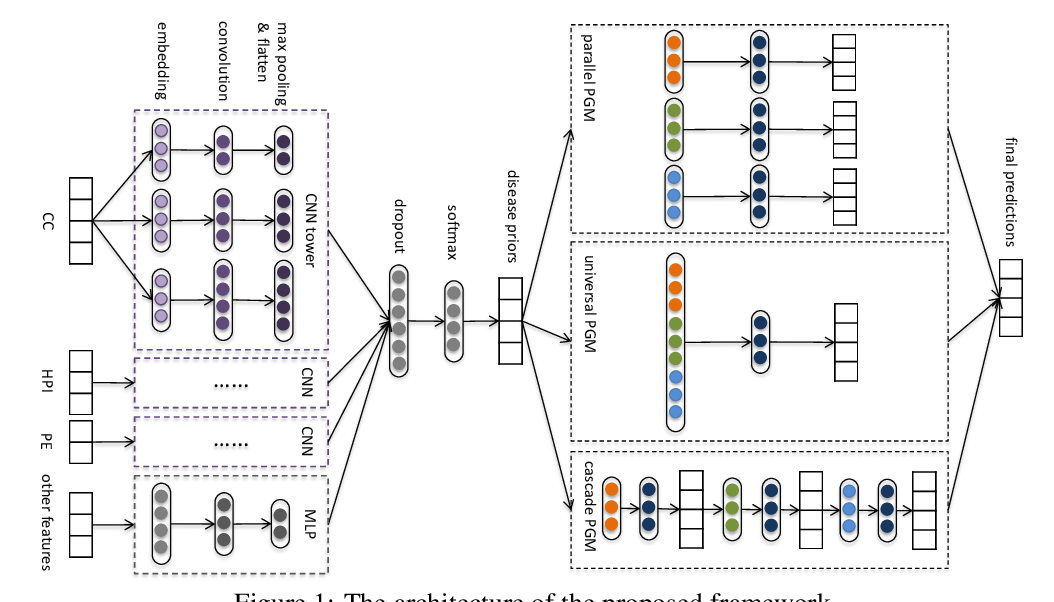