Generating Hierarchical Explanations on Text Classification via Feature Interaction Detection
Hanjie Chen, Guangtao Zheng, Yangfeng Ji
Interpretability and Analysis of Models for NLP Long Paper
Session 9B: Jul 7
(18:00-19:00 GMT)
Session 10B: Jul 7
(21:00-22:00 GMT)
Abstract:
Generating explanations for neural networks has become crucial for their applications in real-world with respect to reliability and trustworthiness. In natural language processing, existing methods usually provide important features which are words or phrases selected from an input text as an explanation, but ignore the interactions between them. It poses challenges for humans to interpret an explanation and connect it to model prediction. In this work, we build hierarchical explanations by detecting feature interactions. Such explanations visualize how words and phrases are combined at different levels of the hierarchy, which can help users understand the decision-making of black-box models. The proposed method is evaluated with three neural text classifiers (LSTM, CNN, and BERT) on two benchmark datasets, via both automatic and human evaluations. Experiments show the effectiveness of the proposed method in providing explanations that are both faithful to models and interpretable to humans.
You can open the
pre-recorded video
in a separate window.
NOTE: The SlidesLive video may display a random order of the authors.
The correct author list is shown at the top of this webpage.
Similar Papers
NILE : Natural Language Inference with Faithful Natural Language Explanations
Sawan Kumar, Partha Talukdar,
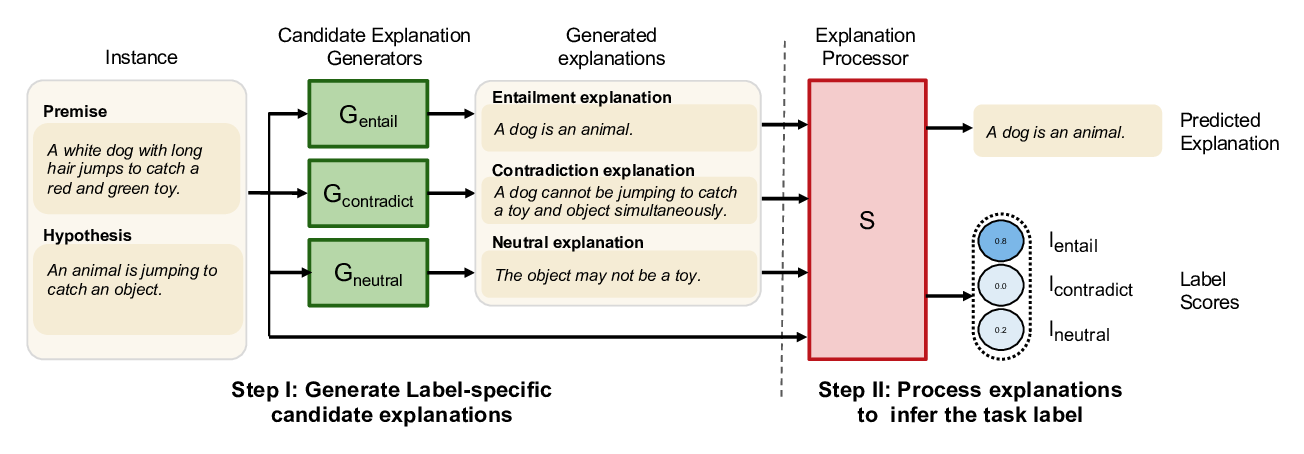
Evaluating Explainable AI: Which Algorithmic Explanations Help Users Predict Model Behavior?
Peter Hase, Mohit Bansal,
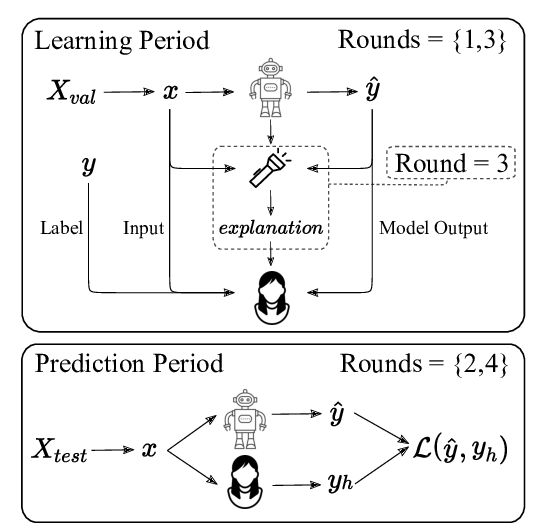
Make Up Your Mind! Adversarial Generation of Inconsistent Natural Language Explanations
Oana-Maria Camburu, Brendan Shillingford, Pasquale Minervini, Thomas Lukasiewicz, Phil Blunsom,
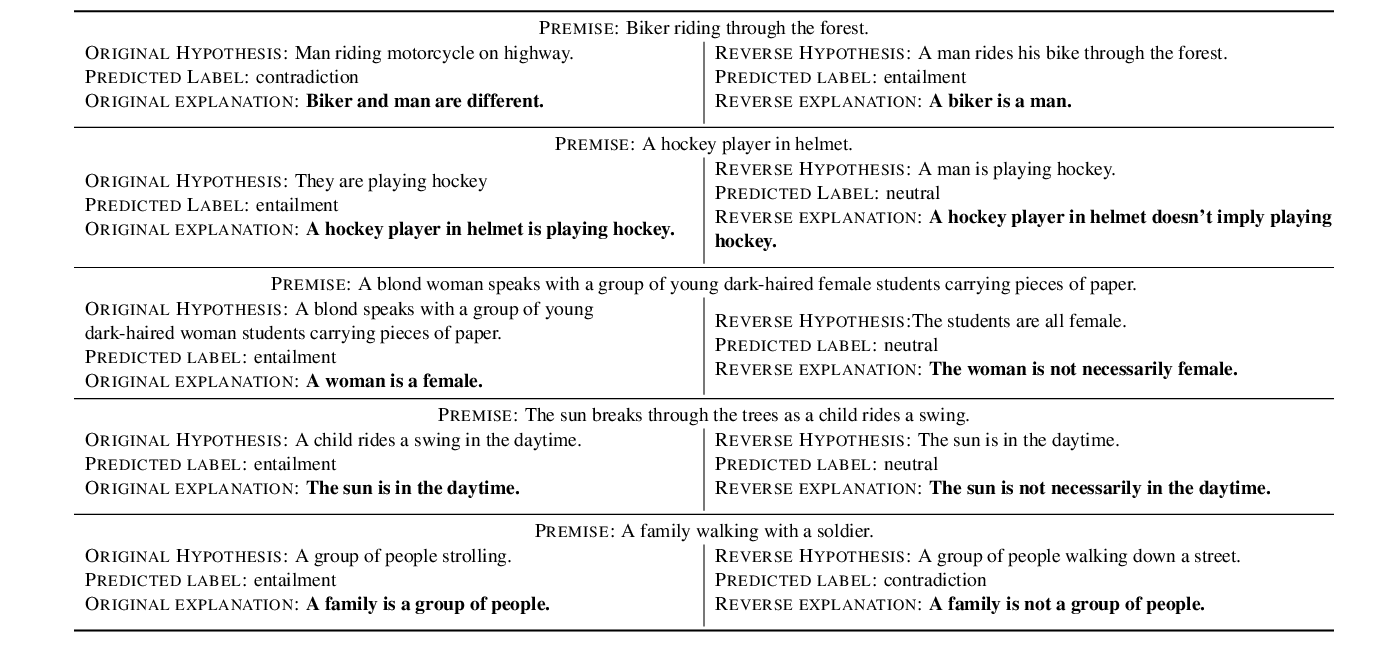
Interpreting Twitter User Geolocation
Ting Zhong, Tianliang Wang, Fan Zhou, Goce Trajcevski, Kunpeng Zhang, Yi Yang,
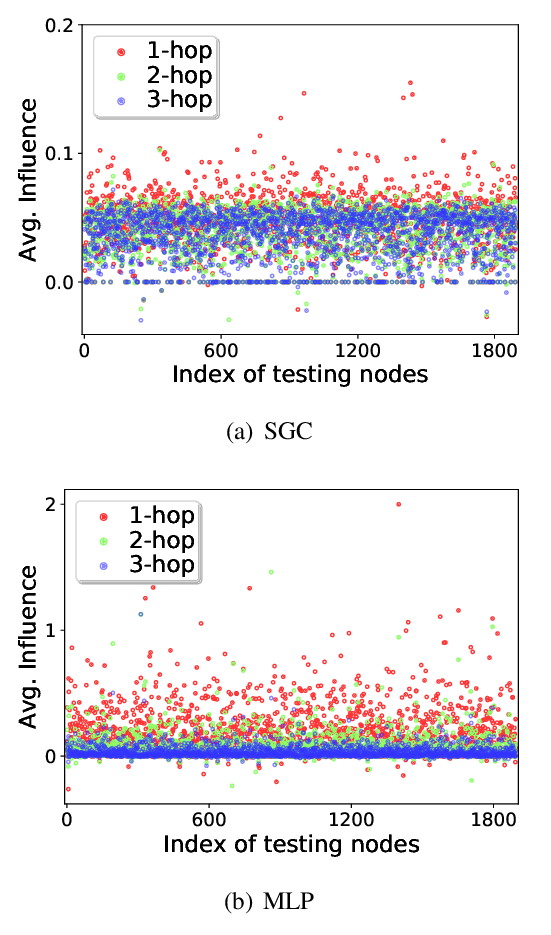