Exploring Interpretability in Event Extraction: Multitask Learning of a Neural Event Classifier and an Explanation Decoder
Zheng Tang, Gus Hahn-Powell, Mihai Surdeanu
Student Research Workshop SRW Paper
Session 4A: Jul 6
(17:00-18:00 GMT)
Session 14A: Jul 8
(17:00-18:00 GMT)
Abstract:
We propose an interpretable approach for event extraction that mitigates the tension between generalization and interpretability by jointly training for the two goals. Our approach uses an encoder-decoder architecture, which jointly trains a classifier for event extraction, and a rule decoder that generates syntactico-semantic rules that explain the decisions of the event classifier. We evaluate the proposed approach on three biomedical events and show that the decoder generates interpretable rules that serve as accurate explanations for the event classifier's decisions, and, importantly, that the joint training generally improves the performance of the event classifier. Lastly, we show that our approach can be used for semi-supervised learning, and that its performance improves when trained on automatically-labeled data generated by a rule-based system.
You can open the
pre-recorded video
in a separate window.
NOTE: The SlidesLive video may display a random order of the authors.
The correct author list is shown at the top of this webpage.
Similar Papers
Evidence-Aware Inferential Text Generation with Vector Quantised Variational AutoEncoder
Daya Guo, Duyu Tang, Nan Duan, Jian Yin, Daxin Jiang, Ming Zhou,
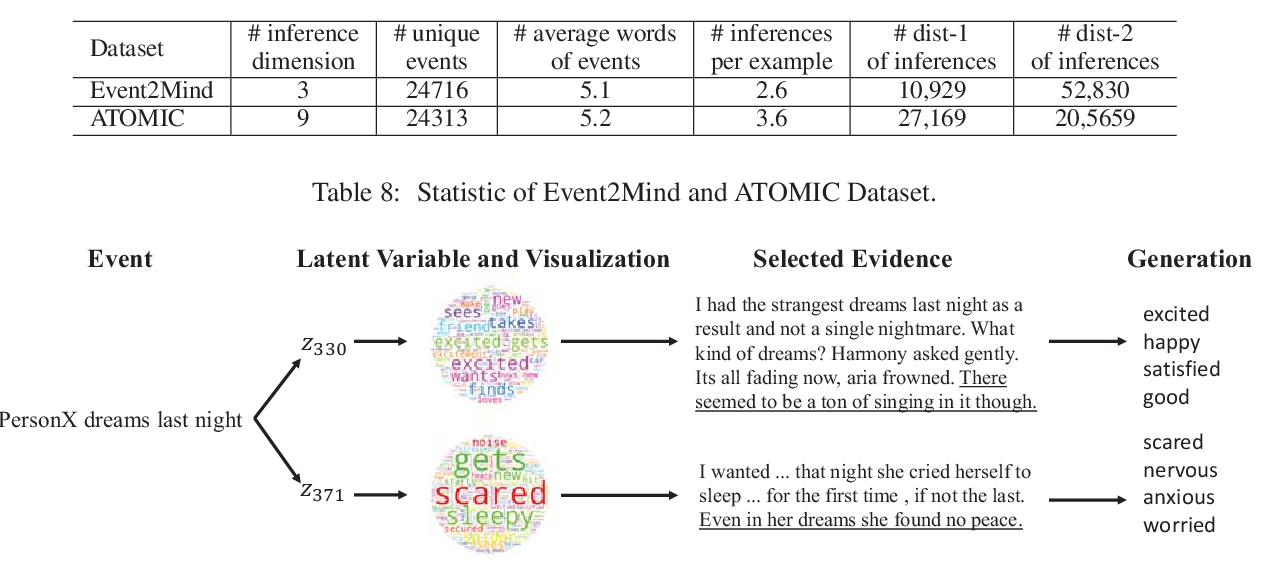
Worse WER, but Better BLEU? Leveraging Word Embedding as Intermediate in Multitask End-to-End Speech Translation
Shun-Po Chuang, Tzu-Wei Sung, Alexander H. Liu, Hung-yi Lee,
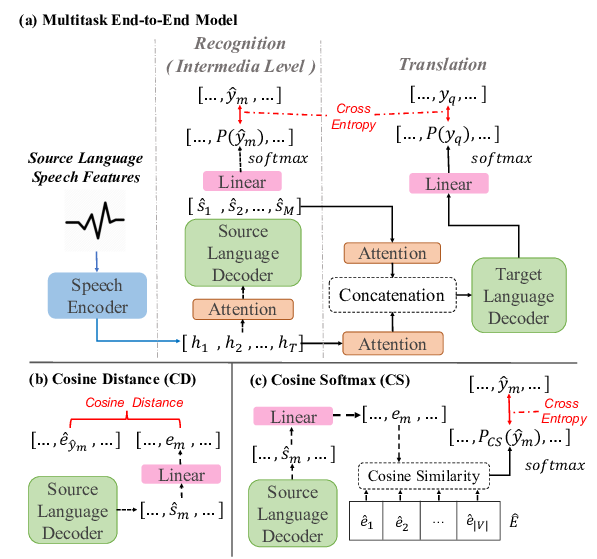
Cross-media Structured Common Space for Multimedia Event Extraction
Manling Li, Alireza Zareian, Qi Zeng, Spencer Whitehead, Di Lu, Heng Ji, Shih-Fu Chang,
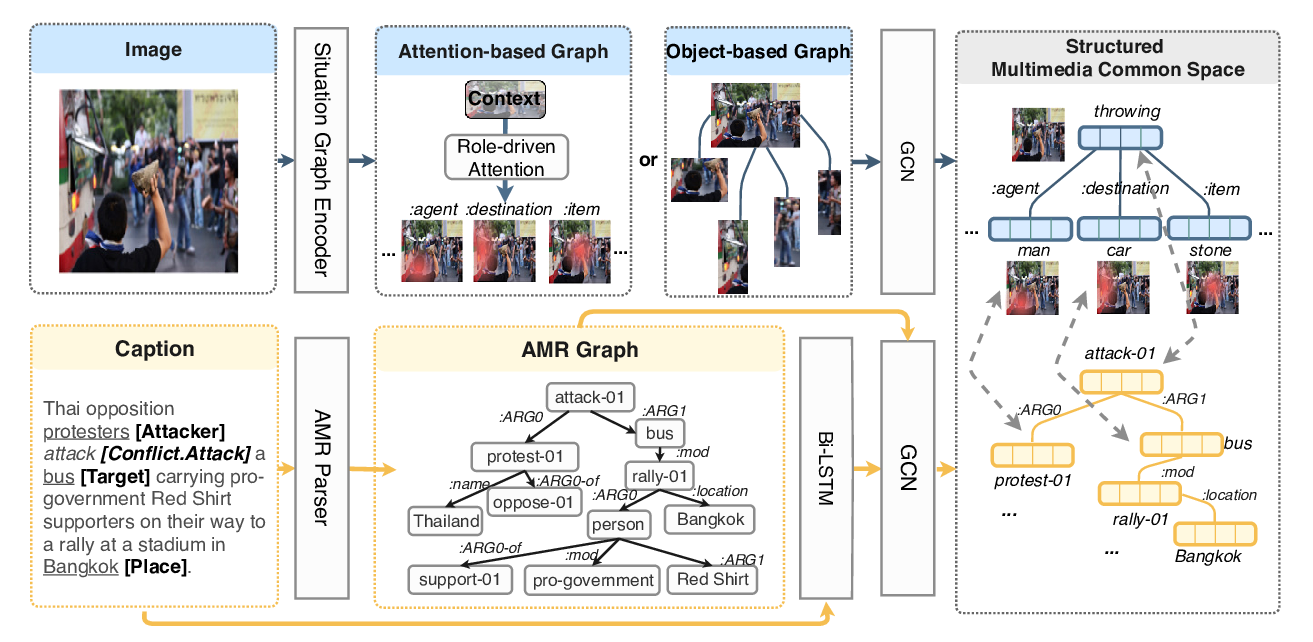
Modeling Label Semantics for Predicting Emotional Reactions
Radhika Gaonkar, Heeyoung Kwon, Mohaddeseh Bastan, Niranjan Balasubramanian, Nathanael Chambers,
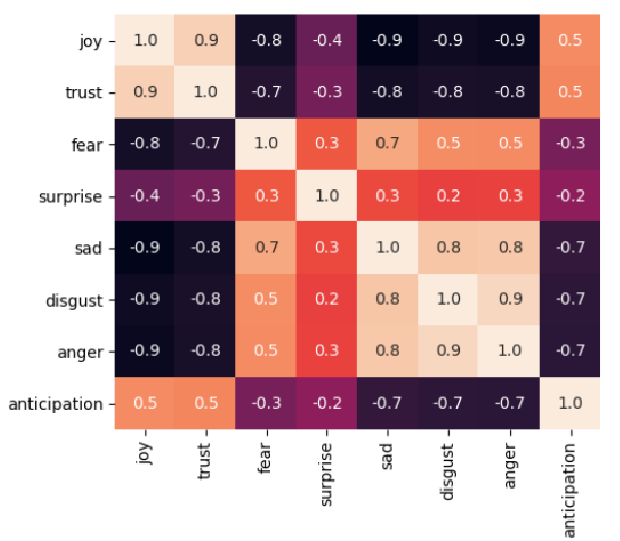