Worse WER, but Better BLEU? Leveraging Word Embedding as Intermediate in Multitask End-to-End Speech Translation
Shun-Po Chuang, Tzu-Wei Sung, Alexander H. Liu, Hung-yi Lee
Machine Translation Short Paper
Session 11A: Jul 8
(05:00-06:00 GMT)
Session 12A: Jul 8
(08:00-09:00 GMT)
Abstract:
Speech translation (ST) aims to learn transformations from speech in the source language to the text in the target language. Previous works show that multitask learning improves the ST performance, in which the recognition decoder generates the text of the source language, and the translation decoder obtains the final translations based on the output of the recognition decoder. Because whether the output of the recognition decoder has the correct semantics is more critical than its accuracy, we propose to improve the multitask ST model by utilizing word embedding as the intermediate.
You can open the
pre-recorded video
in a separate window.
NOTE: The SlidesLive video may display a random order of the authors.
The correct author list is shown at the top of this webpage.
Similar Papers
Jointly Masked Sequence-to-Sequence Model for Non-Autoregressive Neural Machine Translation
Junliang Guo, Linli Xu, Enhong Chen,
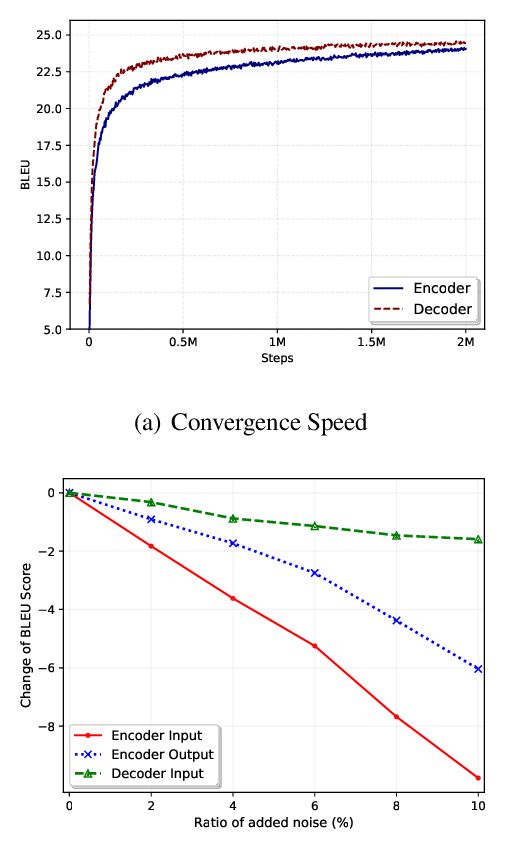
On the Encoder-Decoder Incompatibility in Variational Text Modeling and Beyond
Chen Wu, Prince Zizhuang Wang, William Yang Wang,
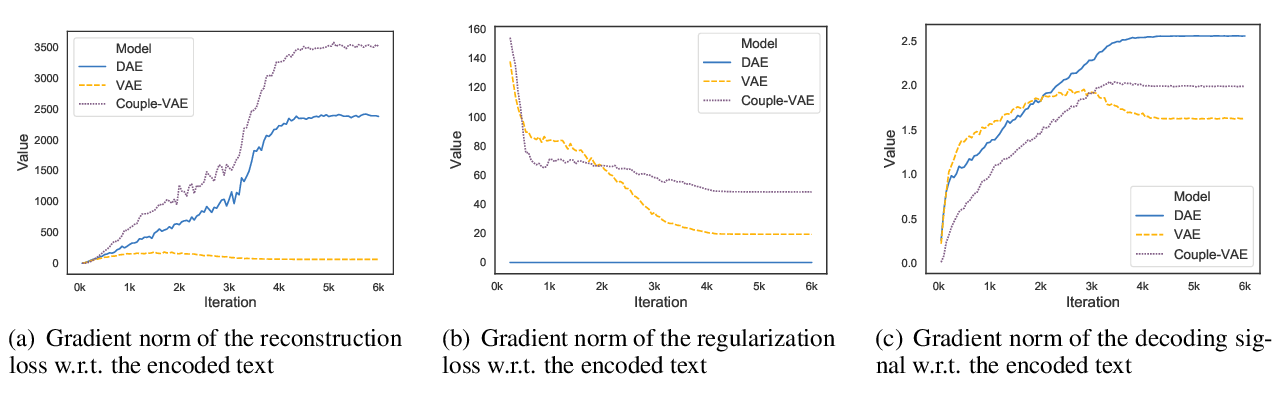
Improving Neural Machine Translation with Soft Template Prediction
Jian Yang, Shuming Ma, Dongdong Zhang, Zhoujun Li, Ming Zhou,
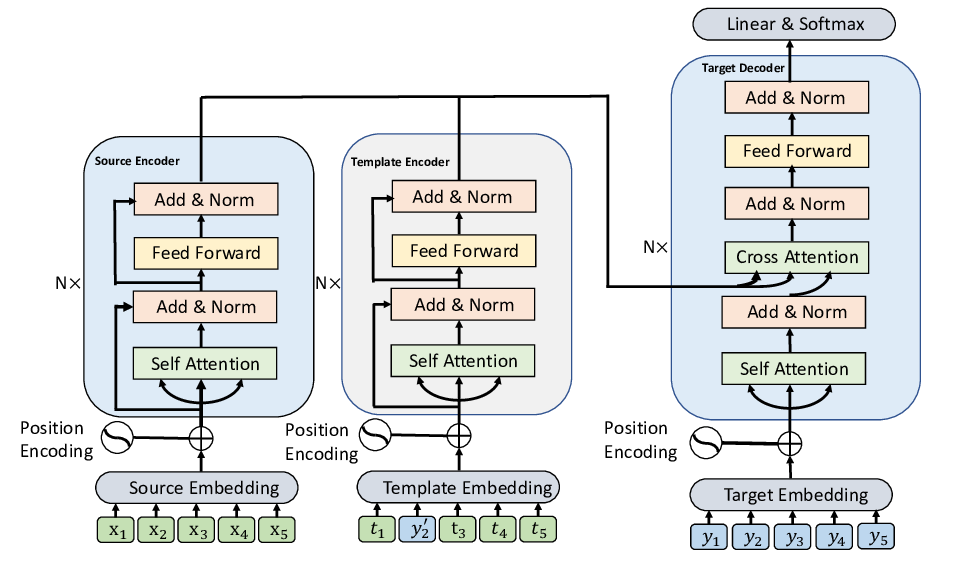
Exploring Interpretability in Event Extraction: Multitask Learning of a Neural Event Classifier and an Explanation Decoder
Zheng Tang, Gus Hahn-Powell, Mihai Surdeanu,
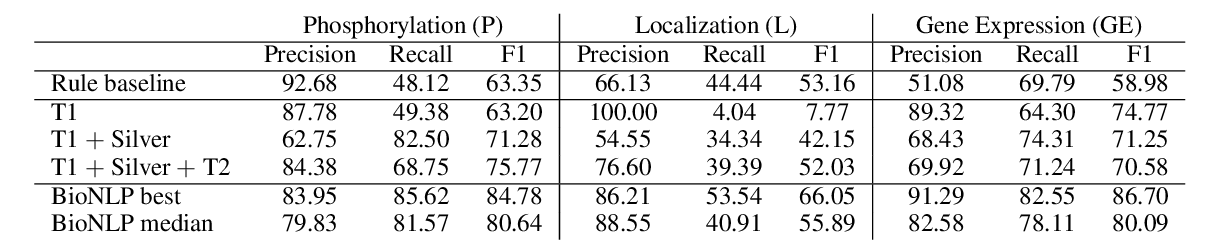