Tabula nearly Rasa: Probing the linguistic knowledge of character-level neural language models trained on unsegmented text
Michael Hahn, Marco Baroni
Interpretability and Analysis of Models for NLP TACL Paper
Session 6B: Jul 7
(06:00-07:00 GMT)
Session 8B: Jul 7
(13:00-14:00 GMT)
Abstract:
Recurrent neural networks (RNNs) reached striking performance in many natural language processing tasks. This has renewed interest in whether these generic sequence processing devices are inducing genuine linguistic knowledge. Nearly all current analytical studies, however, initialize the RNNs with a vocabulary of known words, and feed them tokenized input during training. We present a multi-lingual study of the linguistic knowledge encoded in RNNs trained as character-level language models, on input data with word boundaries removed. These networks face a tougher and more cognitively realistic task, having to discover and store any useful linguistic unit from scratch, based on input statistics. The results show that our "near tabula rasa" RNNs are mostly able to solve morphological, syntactic and semantic tasks that intuitively presuppose word-level knowledge, and indeed they learned to track "soft" word boundaries. Our study opens the door to speculations about the necessity of an explicit word lexicon in language learning and usage.
You can open the
pre-recorded video
in a separate window.
NOTE: The SlidesLive video may display a random order of the authors.
The correct author list is shown at the top of this webpage.
Similar Papers
A Formal Hierarchy of RNN Architectures
William Merrill, Gail Weiss, Yoav Goldberg, Roy Schwartz, Noah A. Smith, Eran Yahav,
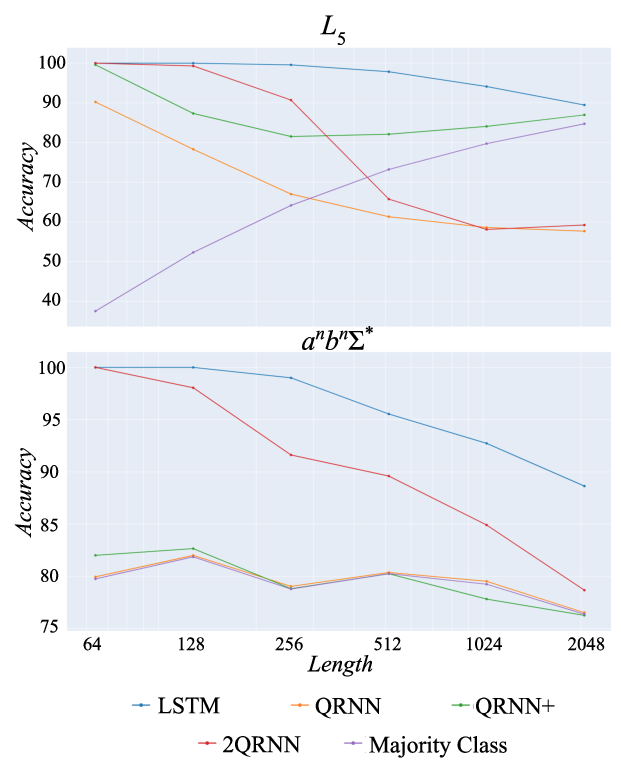
On the Linguistic Representational Power of Neural Machine Translation Models
Yonatan Belinkov, Nadir Durrani, Fahim Dalvi, Hassan Sajjad, James Glass,
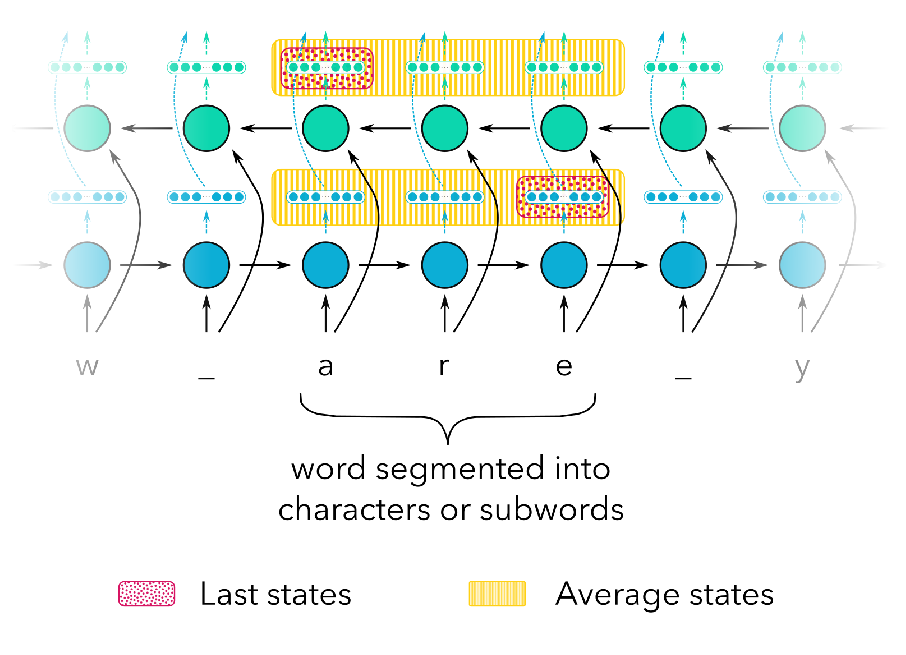
Combining Subword Representations into Word-level Representations in the Transformer Architecture
Noe Casas, Marta R. Costa-jussà, José A. R. Fonollosa,
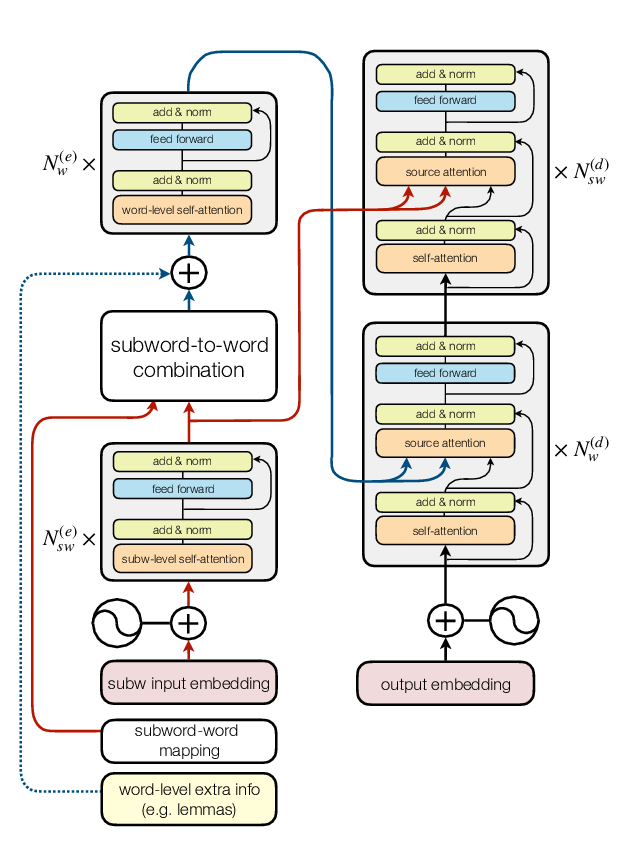
How much complexity does an RNN architecture need to learn syntax-sensitive dependencies?
Gantavya Bhatt, Hritik Bansal, Rishubh Singh, Sumeet Agarwal,
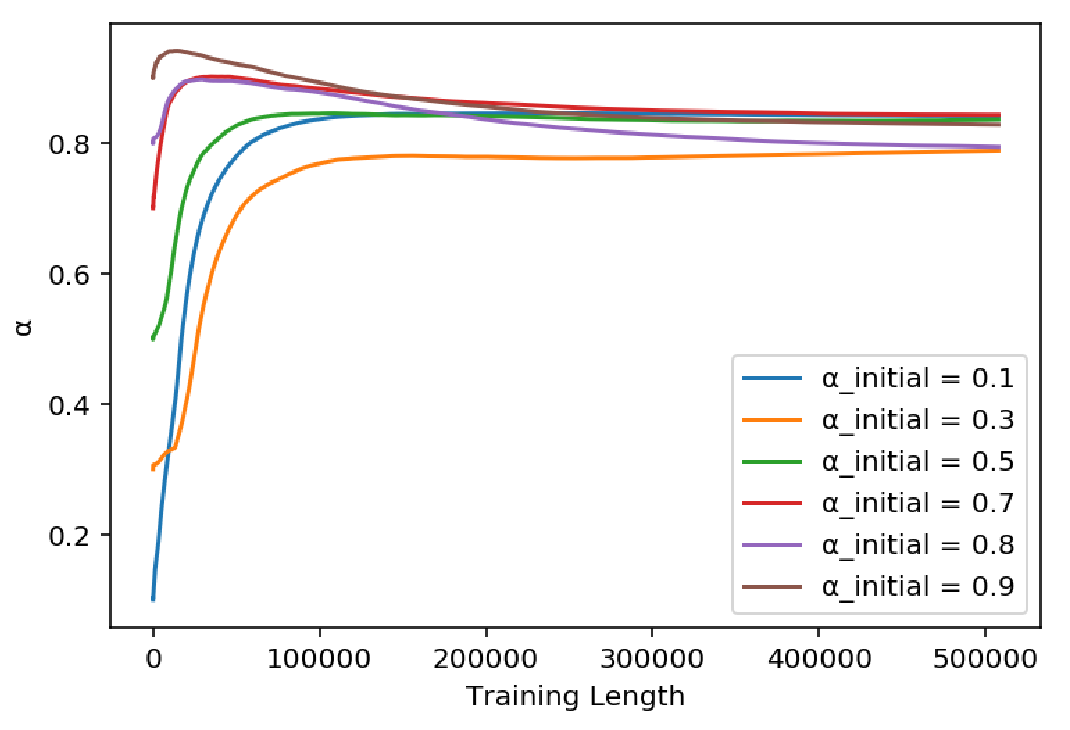