Combining Subword Representations into Word-level Representations in the Transformer Architecture
Noe Casas, Marta R. Costa-jussà, José A. R. Fonollosa
Student Research Workshop SRW Paper
Session 2A: Jul 6
(08:00-09:00 GMT)
Session 14A: Jul 8
(17:00-18:00 GMT)
Abstract:
In Neural Machine Translation, using word-level tokens leads to degradation in translation quality. The dominant approaches use subword-level tokens, but this increases the length of the sequences and makes it difficult to profit from word-level information such as POS tags or semantic dependencies.We propose a modification to the Transformer model to combine subword-level representations into word-level ones in the first layers of the encoder, reducing the effective length of the sequences in the following layers and providing a natural point to incorporate extra word-level information.Our experiments show that this approach maintains the translation quality with respect to the normal Transformer model when no extra word-level information is injected and that it is superior to the currently dominant method for incorporating word-level source language information to models based on subword-level vocabularies.
You can open the
pre-recorded video
in a separate window.
NOTE: The SlidesLive video may display a random order of the authors.
The correct author list is shown at the top of this webpage.
Similar Papers
Character-Level Translation with Self-attention
Yingqiang Gao, Nikola I. Nikolov, Yuhuang Hu, Richard H.R. Hahnloser,
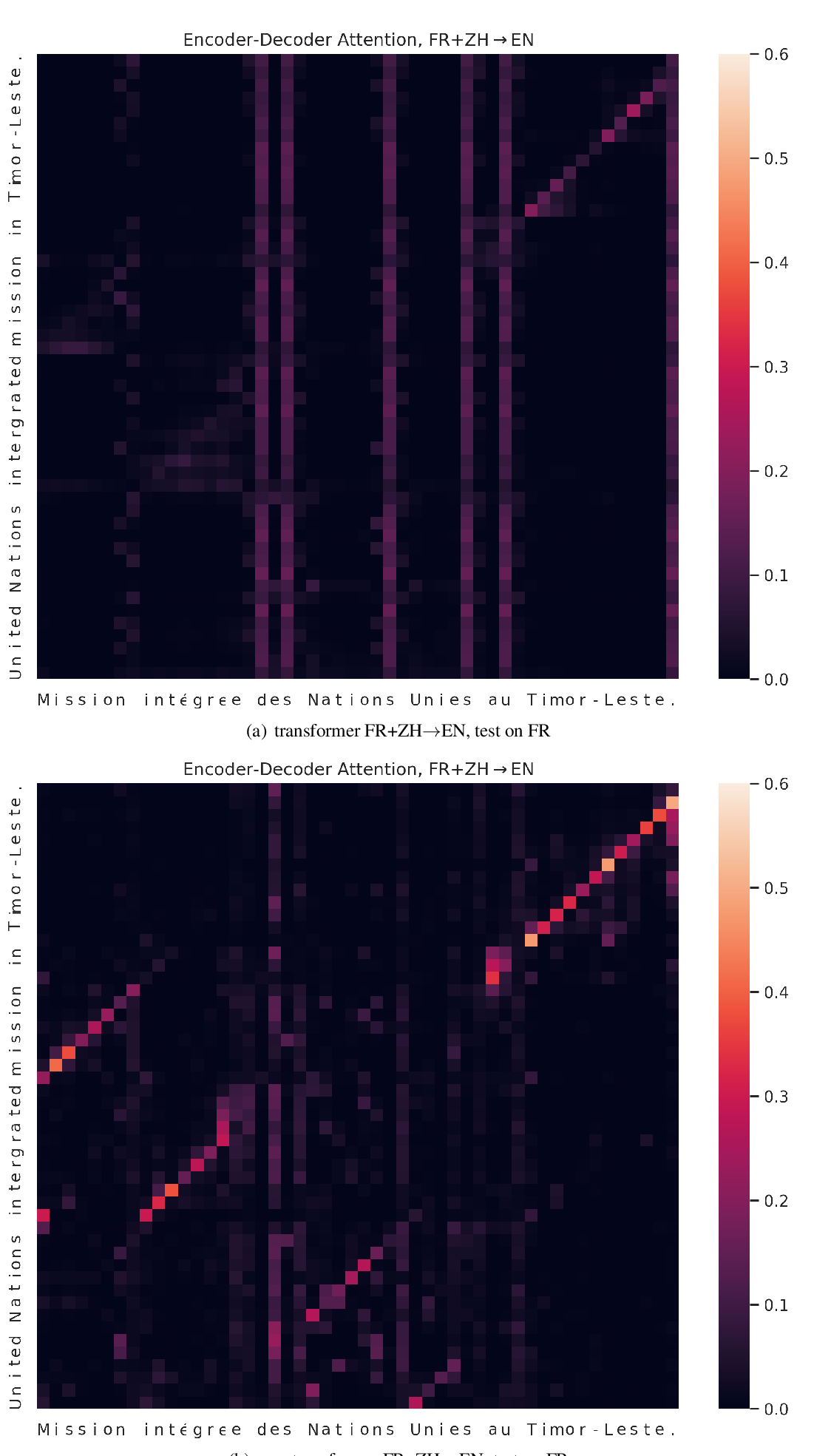
SPECTER: Document-level Representation Learning using Citation-informed Transformers
Arman Cohan, Sergey Feldman, Iz Beltagy, Doug Downey, Daniel Weld,
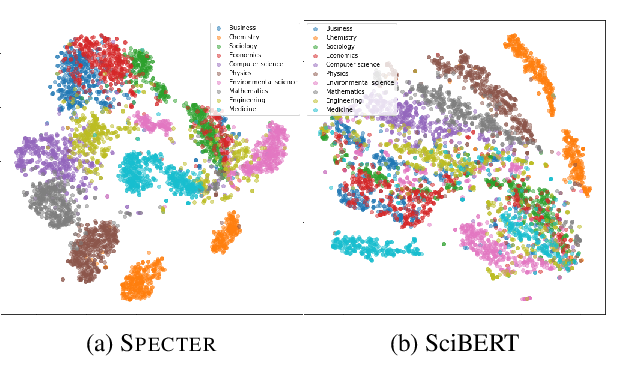
Using Context in Neural Machine Translation Training Objectives
Danielle Saunders, Felix Stahlberg, Bill Byrne,
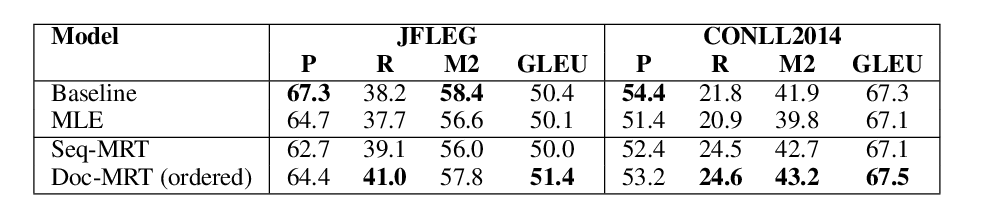