Inherent Disagreements in Human Textual Inferences
Ellie Pavlick, Tom Kwiatkowski
Semantics: Textual Inference and Other Areas of Semantics TACL Paper
Session 4A: Jul 6
(17:00-18:00 GMT)
Session 5A: Jul 6
(20:00-21:00 GMT)
Abstract:
We analyze human’s disagreements about the validity of natural language inferences. We show that, very often, disagreements are not dismissible as annotation “noise”, but rather persist as we collect more ratings and as we vary the amount of context provided to raters. We further show that the type of uncertainty captured by current state-of-the-art models for natural language inference is not reflective of the type of uncertainty present in human disagreements. We discuss implications of our results in relation to the recognizing textual entailment (RTE)/natural language inference (NLI) task. We argue for a refined evaluation objective which requires models to explicitly capture the full distribution of plausible human judgments.
You can open the
pre-recorded video
in a separate window.
NOTE: The SlidesLive video may display a random order of the authors.
The correct author list is shown at the top of this webpage.
Similar Papers
Are Natural Language Inference Models IMPPRESsive? Learning IMPlicature and PRESupposition
Paloma Jeretic, Alex Warstadt, Suvrat Bhooshan, Adina Williams,
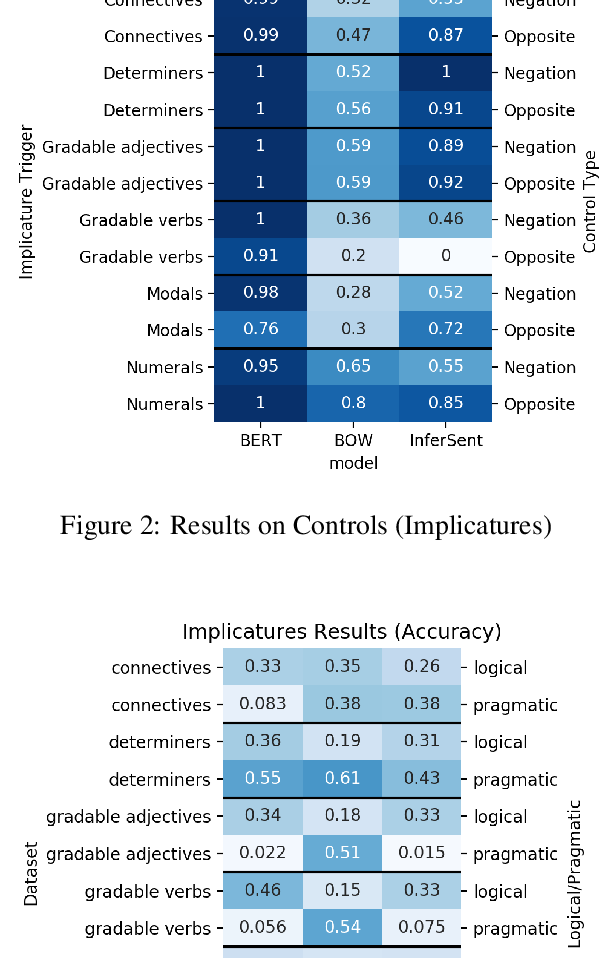
How Furiously Can Colourless Green Ideas Sleep? Sentence Acceptability in Context
Jey Han Lau, Carlos Santos Armendariz, Matthew Purver, Chang Shu, Shalom Lappin,
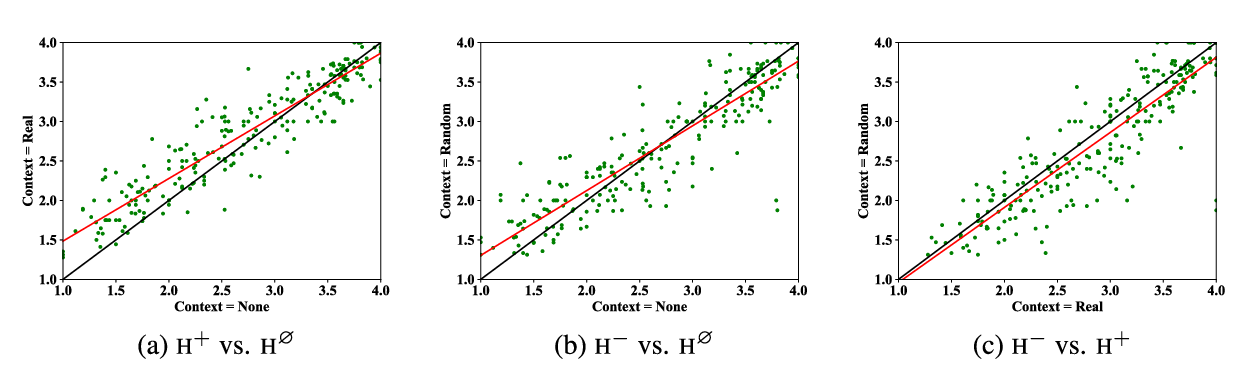
Learning an Unreferenced Metric for Online Dialogue Evaluation
Koustuv Sinha, Prasanna Parthasarathi, Jasmine Wang, Ryan Lowe, William L. Hamilton, Joelle Pineau,
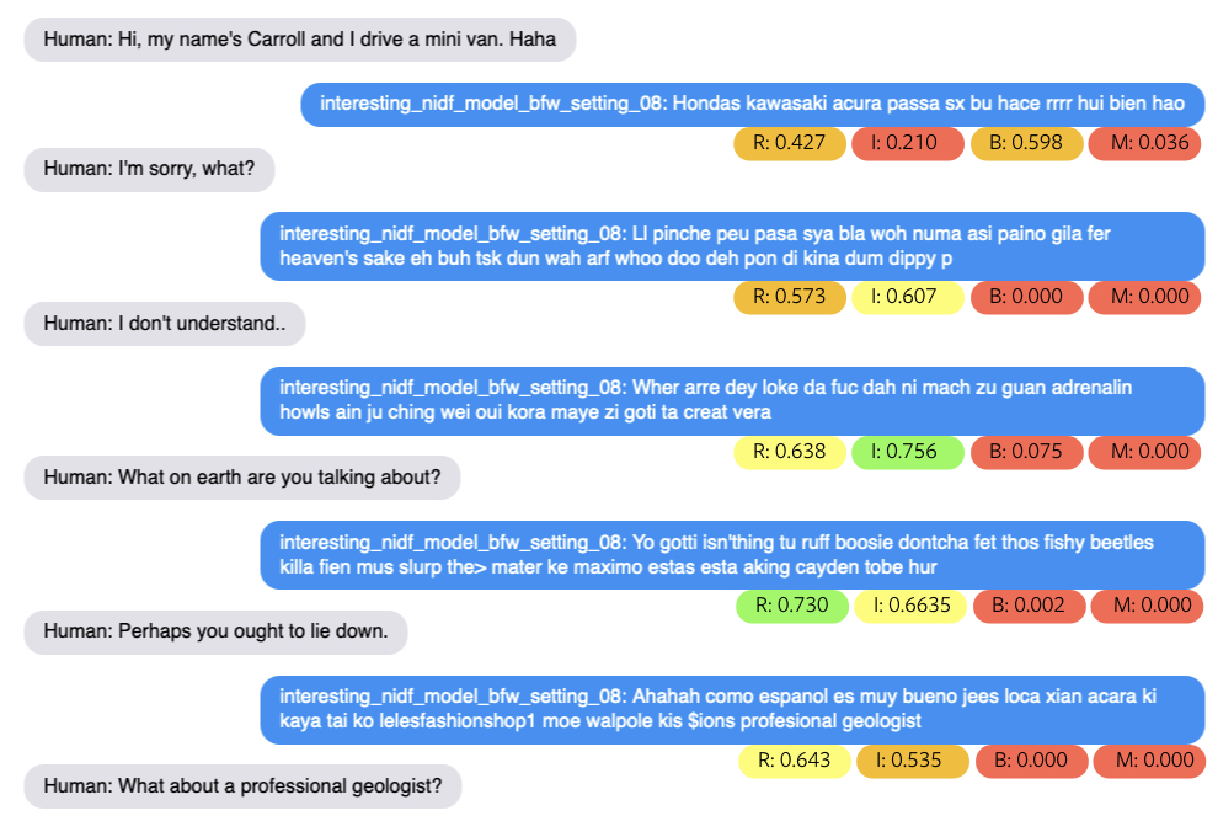
On Faithfulness and Factuality in Abstractive Summarization
Joshua Maynez, Shashi Narayan, Bernd Bohnet, Ryan McDonald,
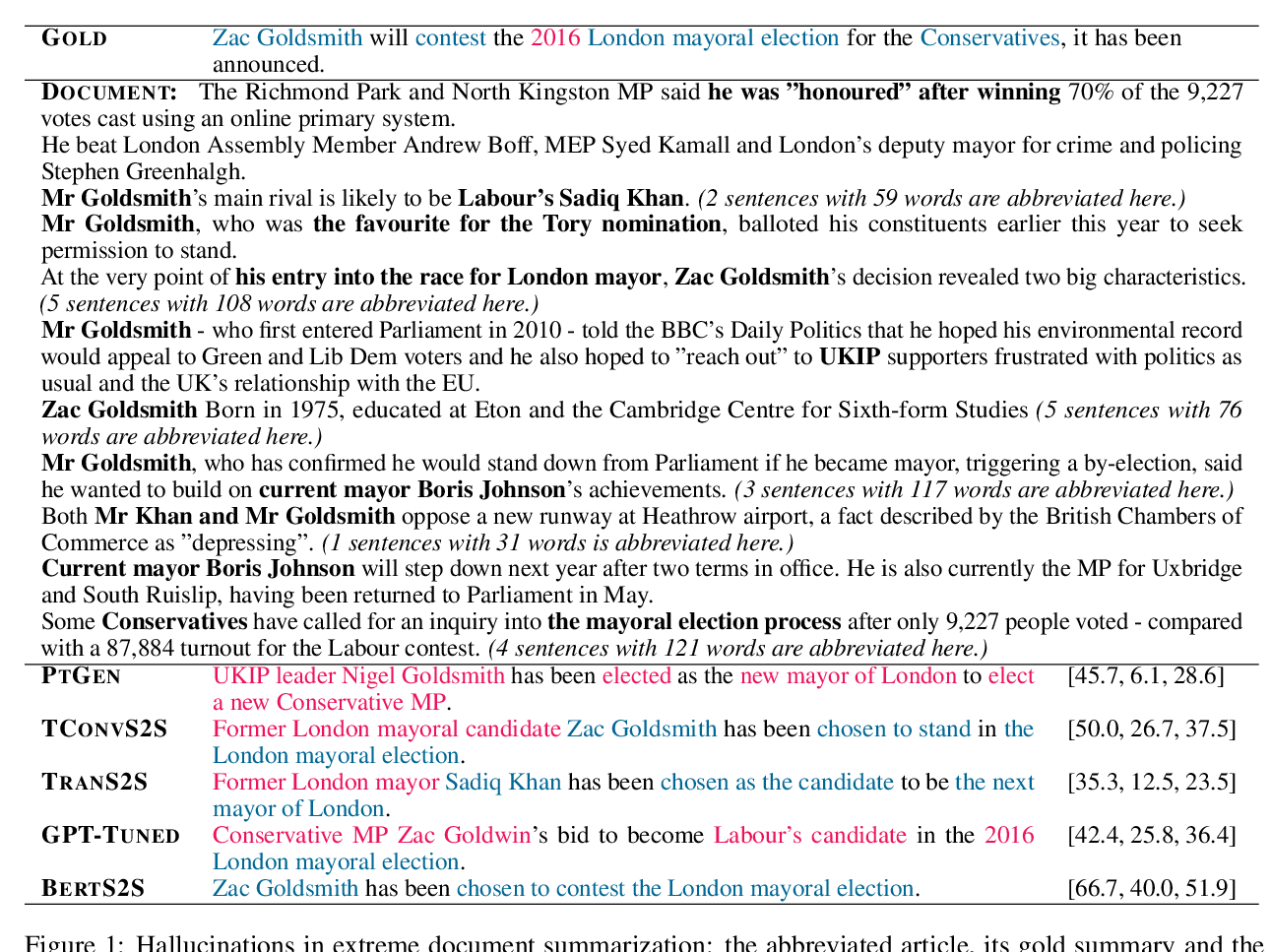