A Systematic Study of Inner-Attention-Based Sentence Representations in Multilingual Neural Machine Translation
Raúl Vázquez, Alessandro Raganato, Mathias Creutz, Jörg Tiedemann
Machine Translation CL Paper
Session 7A: Jul 7
(08:00-09:00 GMT)
Session 8B: Jul 7
(13:00-14:00 GMT)
Abstract:
Neural machine translation has considerably improved the quality of automatic translations by learning good representations of input sentences. In this article, we explore a multilingual translation model capable of producing fixed-size sentence representations by incorporating an intermediate crosslingual shared layer, which we refer to as attention bridge. This layer exploits the semantics from each language and develops into a language-agnostic meaning representation that can be efficiently used for transfer learning.We systematically study the impact of the size of the attention bridge and the effect of including additional languages in the model. In contrast to related previous work, we demonstrate that there is no conflict between translation performance and the use of sentence representations in downstream tasks. In particular, we show that larger intermediate layers not only improve translation quality, especially for long sentences, but also push the accuracy of trainable classification tasks. Nevertheless, shorter representations lead to increased compression that is beneficial in non-trainable similarity tasks. Similarly, we show that trainable downstream tasks benefit from multilingual models, whereas additional language signals do not improve performance in non-trainable benchmarks. This is an important insight that helps to properly design models for specific applications. Finally, we also include an in-depth analysis of the proposed attention bridge and its ability of encoding linguistic properties. We carefully analyze the information that is captured by individual attention heads and identify interesting patterns that explain the performance of specific settings in linguistic probing tasks.
You can open the
pre-recorded video
in a separate window.
NOTE: The SlidesLive video may display a random order of the authors.
The correct author list is shown at the top of this webpage.
Similar Papers
Roles and Utilization of Attention Heads in Transformer-based Neural Language Models
Jae-young Jo, Sung-Hyon Myaeng,
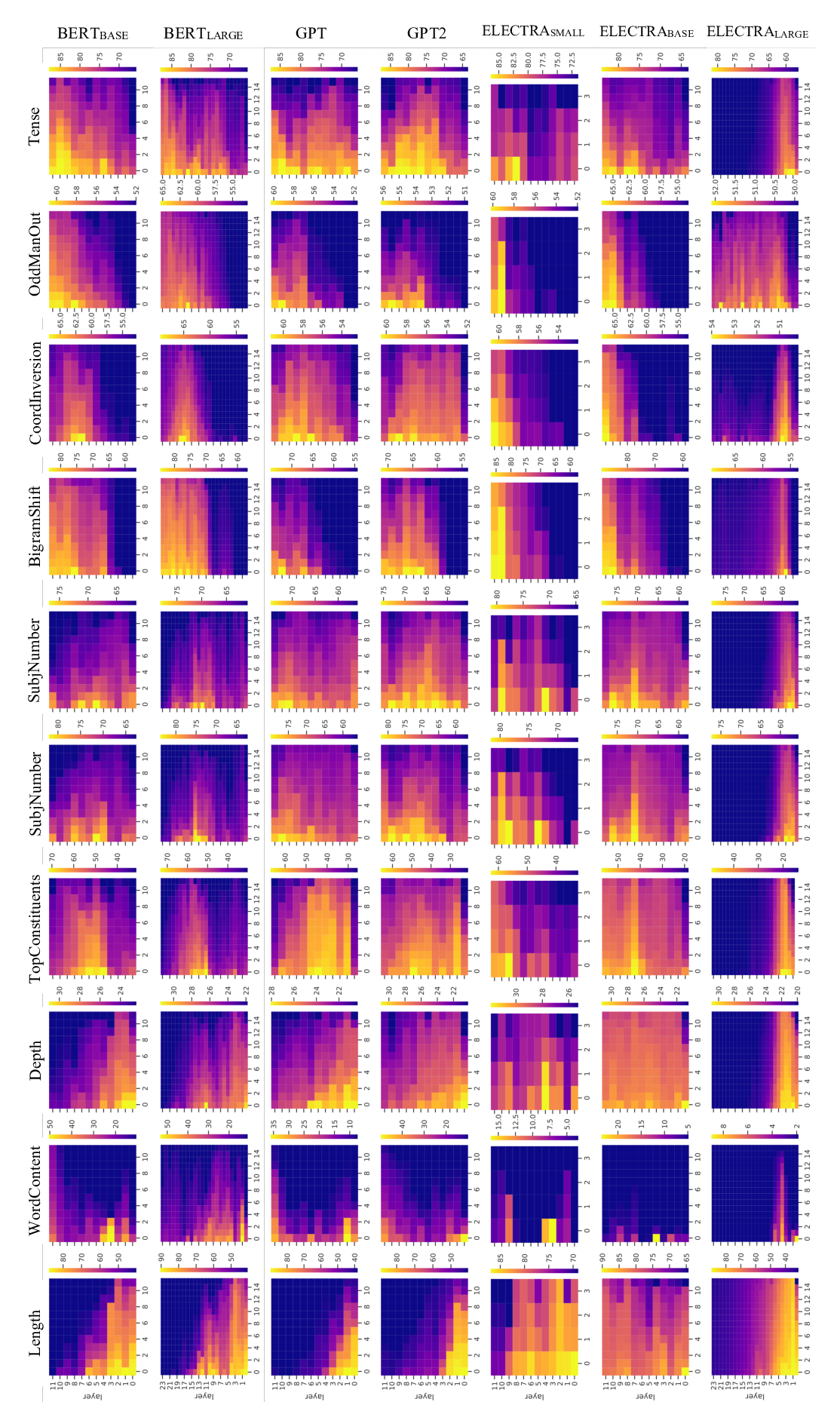
Similarity Analysis of Contextual Word Representation Models
John Wu, Yonatan Belinkov, Hassan Sajjad, Nadir Durrani, Fahim Dalvi, James Glass,
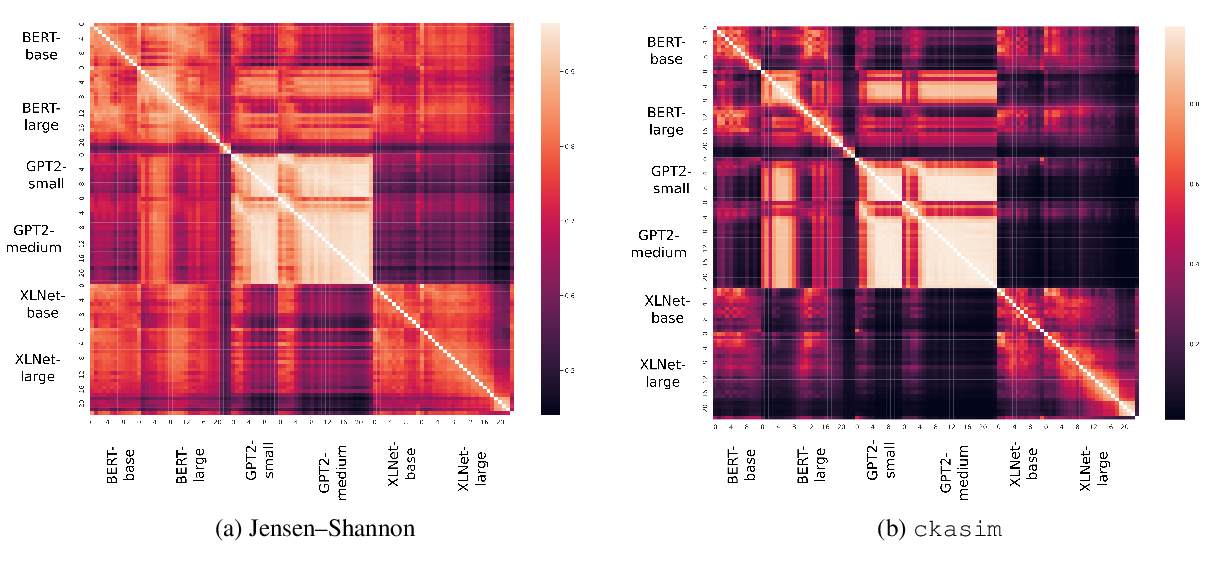