Similarity Analysis of Contextual Word Representation Models
John Wu, Yonatan Belinkov, Hassan Sajjad, Nadir Durrani, Fahim Dalvi, James Glass
Interpretability and Analysis of Models for NLP Long Paper
Session 8B: Jul 7
(13:00-14:00 GMT)
Session 9A: Jul 7
(17:00-18:00 GMT)
Abstract:
This paper investigates contextual word representation models from the lens of similarity analysis. Given a collection of trained models, we measure the similarity of their internal representations and attention. Critically, these models come from vastly different architectures. We use existing and novel similarity measures that aim to gauge the level of localization of information in the deep models, and facilitate the investigation of which design factors affect model similarity, without requiring any external linguistic annotation. The analysis reveals that models within the same family are more similar to one another, as may be expected. Surprisingly, different architectures have rather similar representations, but different individual neurons. We also observed differences in information localization in lower and higher layers and found that higher layers are more affected by fine-tuning on downstream tasks.
You can open the
pre-recorded video
in a separate window.
NOTE: The SlidesLive video may display a random order of the authors.
The correct author list is shown at the top of this webpage.
Similar Papers
A Systematic Study of Inner-Attention-Based Sentence Representations in Multilingual Neural Machine Translation
Raúl Vázquez, Alessandro Raganato, Mathias Creutz, Jörg Tiedemann,
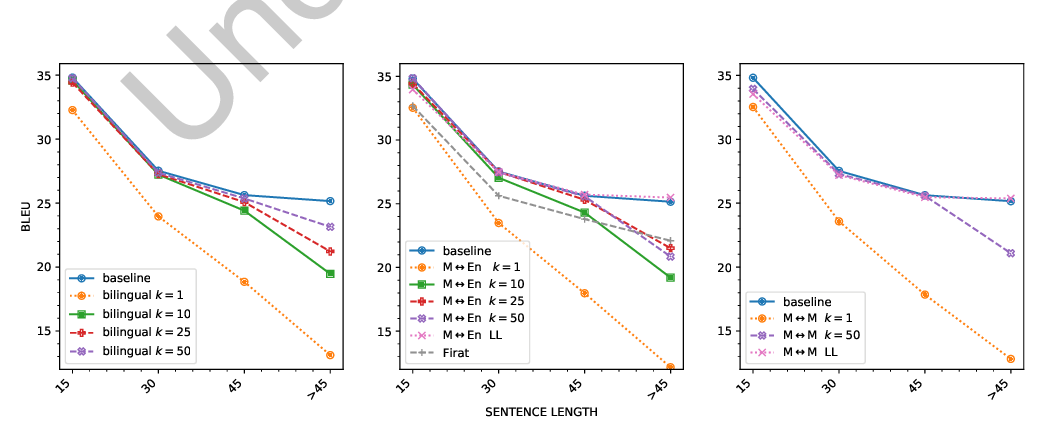
On the Linguistic Representational Power of Neural Machine Translation Models
Yonatan Belinkov, Nadir Durrani, Fahim Dalvi, Hassan Sajjad, James Glass,
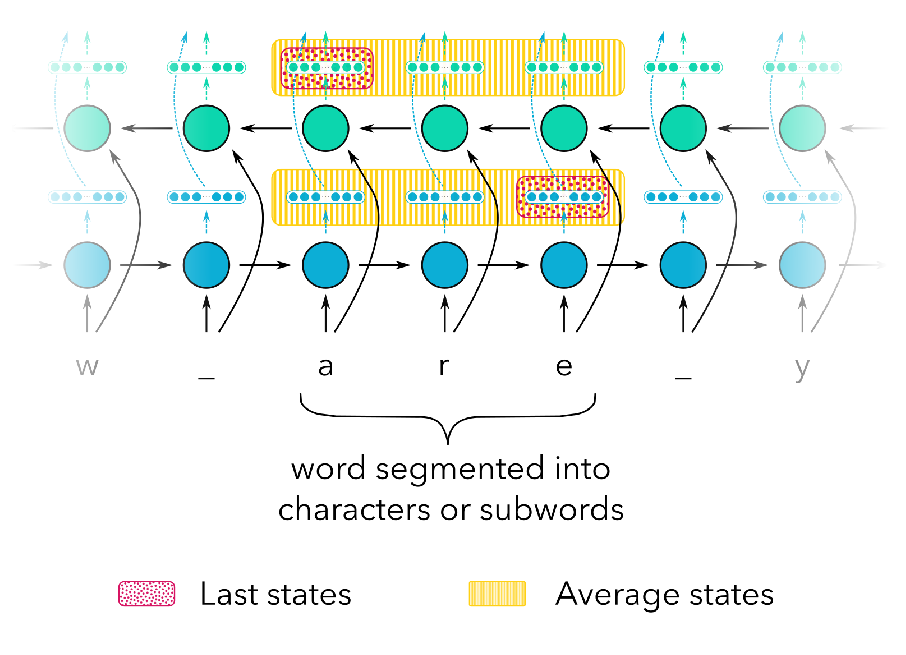
exBERT: A Visual Analysis Tool to Explore Learned Representations in Transformer Models
Benjamin Hoover, Hendrik Strobelt, Sebastian Gehrmann,
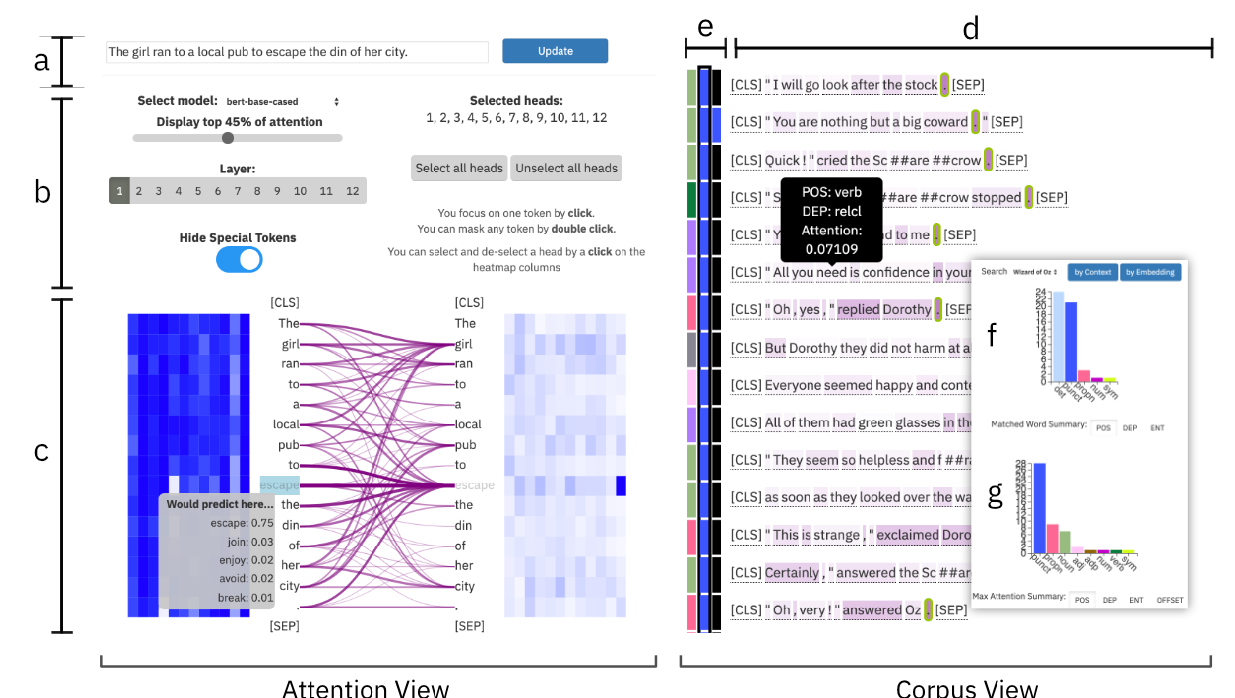
Interpreting Pretrained Contextualized Representations via Reductions to Static Embeddings
Rishi Bommasani, Kelly Davis, Claire Cardie,
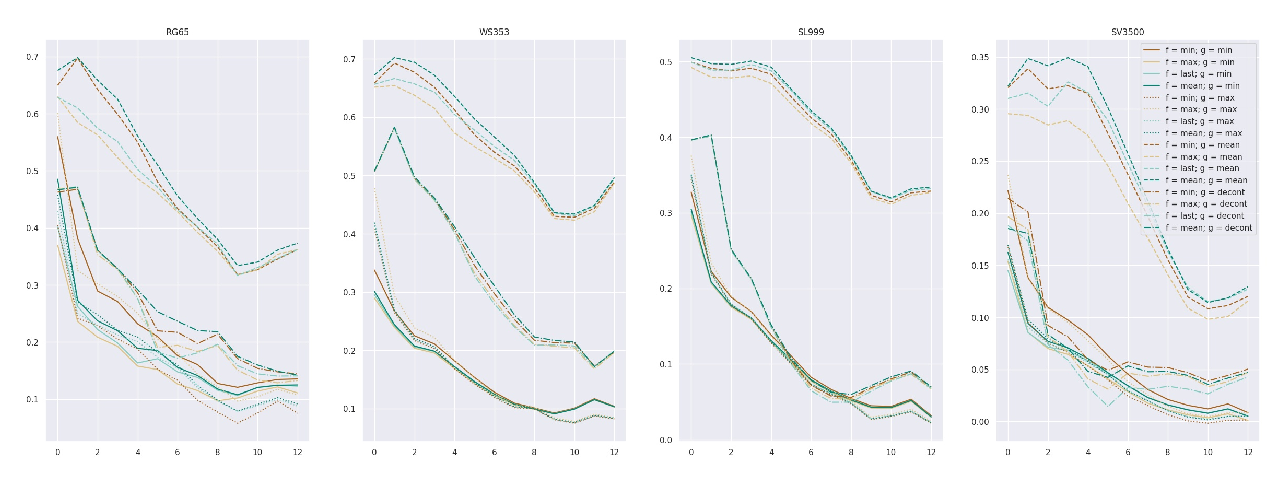