Talk to Papers: Bringing Neural Question Answering to Academic Search
Tiancheng Zhao, Kyusong Lee
System Demonstrations Demo Paper
Demo Session 2A-1: Jul 6
(08:00-09:00 GMT)
Demo Session 4B-1: Jul 6
(17:45-18:45 GMT)
Abstract:
We introduce Talk to Papers, which exploits the recent open-domain question answering (QA) techniques to improve the current experience of academic search. It's designed to enable researchers to use natural language queries to find precise answers and extract insights from a massive amount of academic papers. We present a large improvement over classic search engine baseline on several standard QA datasets and provide the community a collaborative data collection tool to curate the first natural language processing research QA dataset via a community effort.
You can open the
pre-recorded video
in a separate window.
NOTE: The SlidesLive video may display a random order of the authors.
The correct author list is shown at the top of this webpage.
Similar Papers
Template-Based Question Generation from Retrieved Sentences for Improved Unsupervised Question Answering
Alexander Fabbri, Patrick Ng, Zhiguo Wang, Ramesh Nallapati, Bing Xiang,
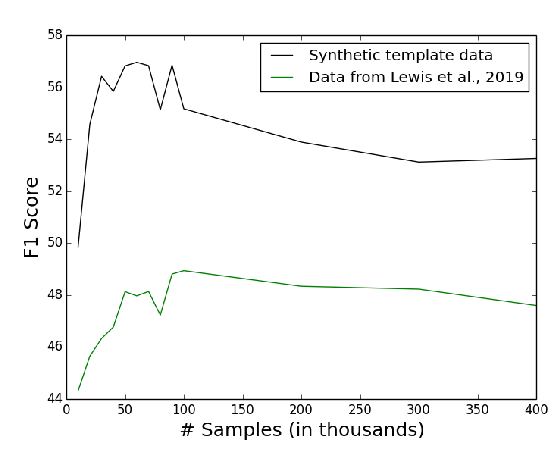
DoQA - Accessing Domain-Specific FAQs via Conversational QA
Jon Ander Campos, Arantxa Otegi, Aitor Soroa, Jan Deriu, Mark Cieliebak, Eneko Agirre,
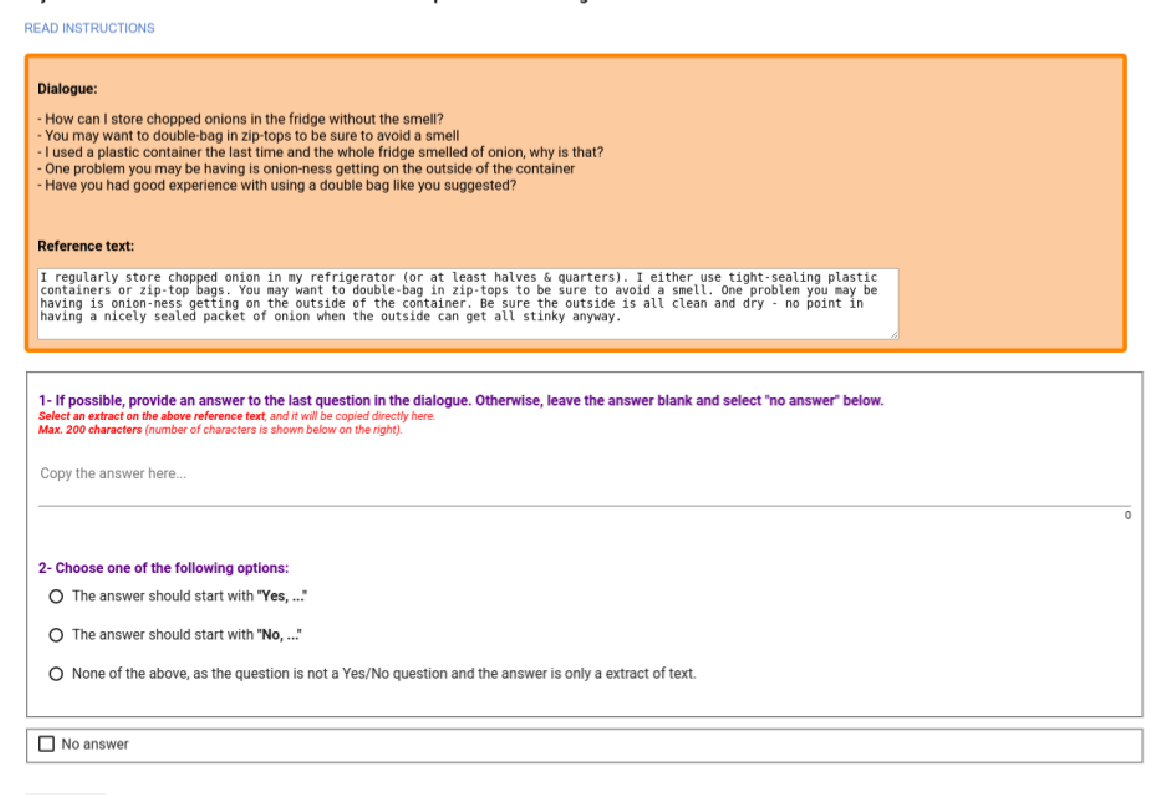
Controlled Crowdsourcing for High-Quality QA-SRL Annotation
Paul Roit, Ayal Klein, Daniela Stepanov, Jonathan Mamou, Julian Michael, Gabriel Stanovsky, Luke Zettlemoyer, Ido Dagan,
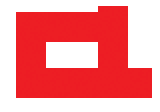