What Question Answering can Learn from Trivia Nerds
Jordan Boyd-Graber, Benjamin Börschinger
Theme Long Paper
Session 12B: Jul 8
(09:00-10:00 GMT)
Session 13B: Jul 8
(13:00-14:00 GMT)
Abstract:
In addition to the traditional task of machines answering questions, question answering (QA) research creates interesting, challenging questions that help systems how to answer questions and reveal the best systems. We argue that creating a QA dataset—and the ubiquitous leaderboard that goes with it—closely resembles running a trivia tournament: you write questions, have agents (either humans or machines) answer the questions, and declare a winner. However, the research community has ignored the hard-learned lessons from decades of the trivia community creating vibrant, fair, and effective question answering competitions. After detailing problems with existing QA datasets, we outline the key lessons—removing ambiguity, discriminating skill, and adjudicating disputes---that can transfer to QA research and how they might be implemented.
You can open the
pre-recorded video
in a separate window.
NOTE: The SlidesLive video may display a random order of the authors.
The correct author list is shown at the top of this webpage.
Similar Papers
Template-Based Question Generation from Retrieved Sentences for Improved Unsupervised Question Answering
Alexander Fabbri, Patrick Ng, Zhiguo Wang, Ramesh Nallapati, Bing Xiang,
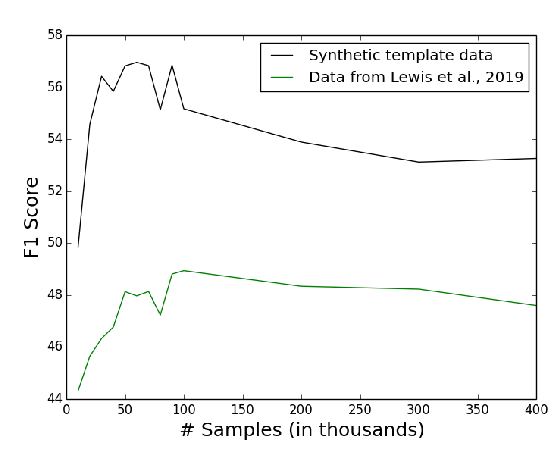
Generating Diverse and Consistent QA pairs from Contexts with Information-Maximizing Hierarchical Conditional VAEs
Dong Bok Lee, Seanie Lee, Woo Tae Jeong, Donghwan Kim, Sung Ju Hwang,
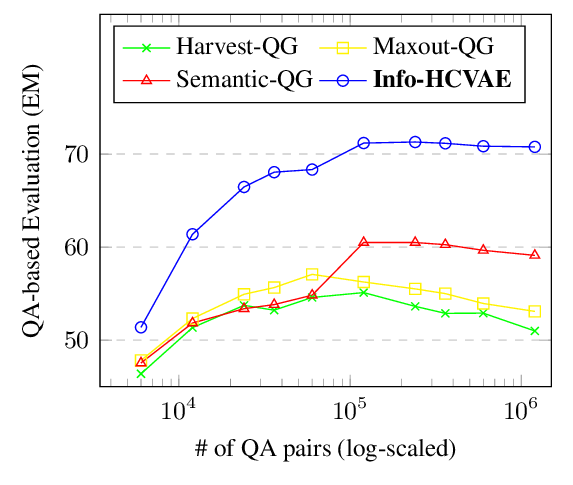
Harvesting and Refining Question-Answer Pairs for Unsupervised QA
Zhongli Li, Wenhui Wang, Li Dong, Furu Wei, Ke Xu,
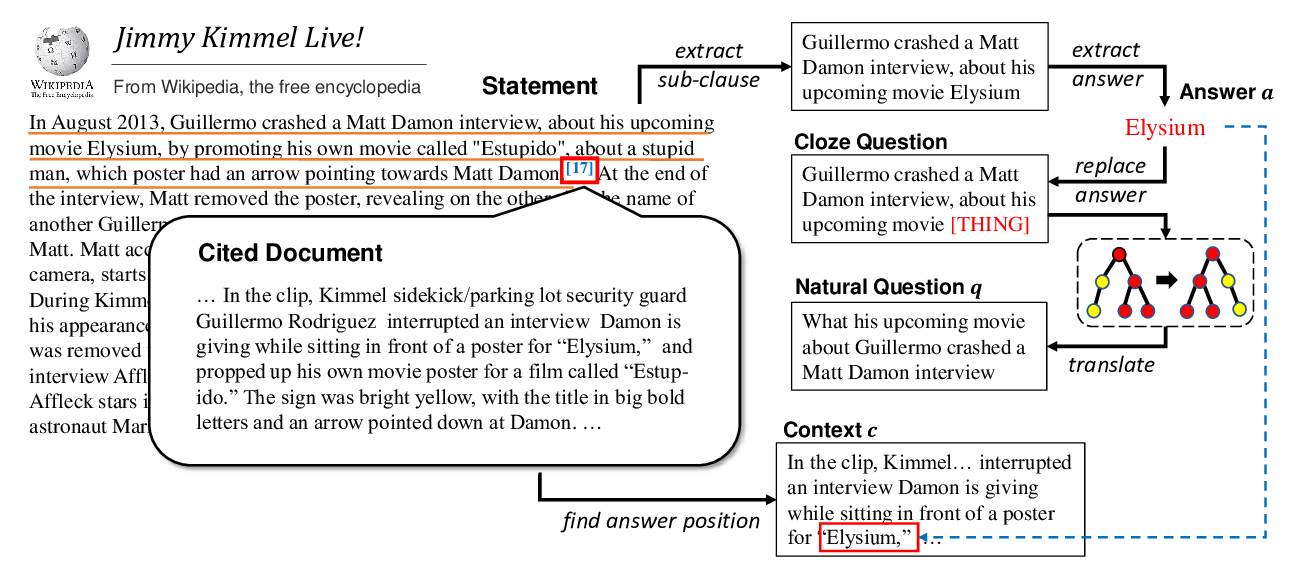
DoQA - Accessing Domain-Specific FAQs via Conversational QA
Jon Ander Campos, Arantxa Otegi, Aitor Soroa, Jan Deriu, Mark Cieliebak, Eneko Agirre,
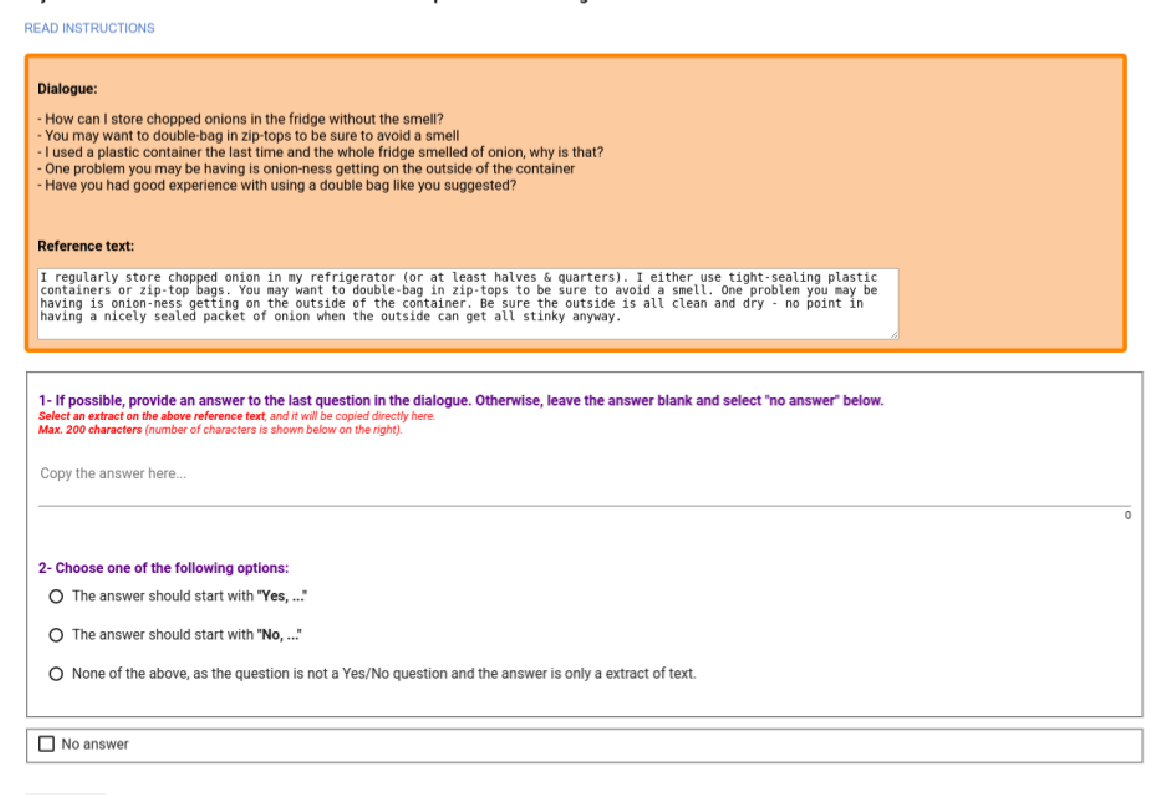