Controlled Crowdsourcing for High-Quality QA-SRL Annotation
Paul Roit, Ayal Klein, Daniela Stepanov, Jonathan Mamou, Julian Michael, Gabriel Stanovsky, Luke Zettlemoyer, Ido Dagan
Semantics: Sentence Level Short Paper
Session 12A: Jul 8
(08:00-09:00 GMT)
Session 15B: Jul 8
(21:00-22:00 GMT)
Abstract:
Question-answer driven Semantic Role Labeling (QA-SRL) was proposed as an attractive open and natural flavour of SRL, potentially attainable from laymen. Recently, a large-scale crowdsourced QA-SRL corpus and a trained parser were released. Trying to replicate the QA-SRL annotation for new texts, we found that the resulting annotations were lacking in quality, particularly in coverage, making them insufficient for further research and evaluation. In this paper, we present an improved crowdsourcing protocol for complex semantic annotation, involving worker selection and training, and a data consolidation phase. Applying this protocol to QA-SRL yielded high-quality annotation with drastically higher coverage, producing a new gold evaluation dataset. We believe that our annotation protocol and gold standard will facilitate future replicable research of natural semantic annotations.
You can open the
pre-recorded video
in a separate window.
NOTE: The SlidesLive video may display a random order of the authors.
The correct author list is shown at the top of this webpage.
Similar Papers
Template-Based Question Generation from Retrieved Sentences for Improved Unsupervised Question Answering
Alexander Fabbri, Patrick Ng, Zhiguo Wang, Ramesh Nallapati, Bing Xiang,
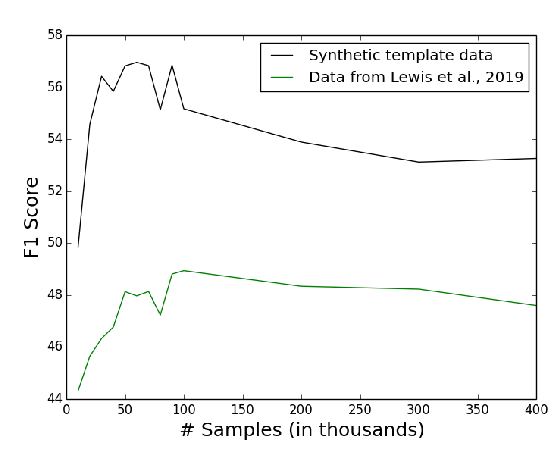
On the Importance of Diversity in Question Generation for QA
Md Arafat Sultan, Shubham Chandel, Ramón Fernandez Astudillo, Vittorio Castelli,
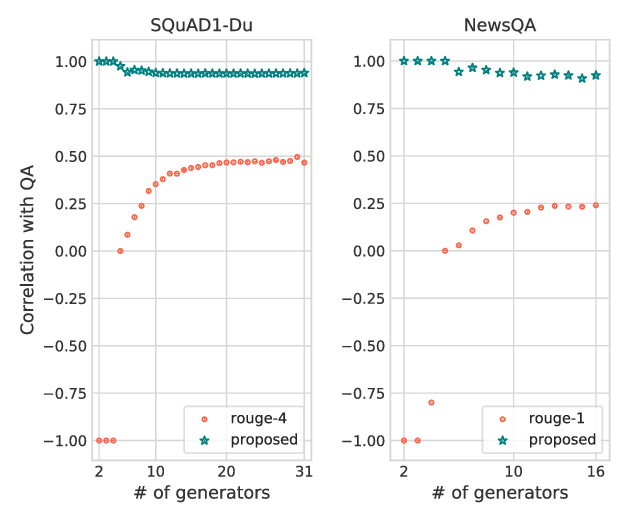
Asking and Answering Questions to Evaluate the Factual Consistency of Summaries
Alex Wang, Kyunghyun Cho, Mike Lewis,
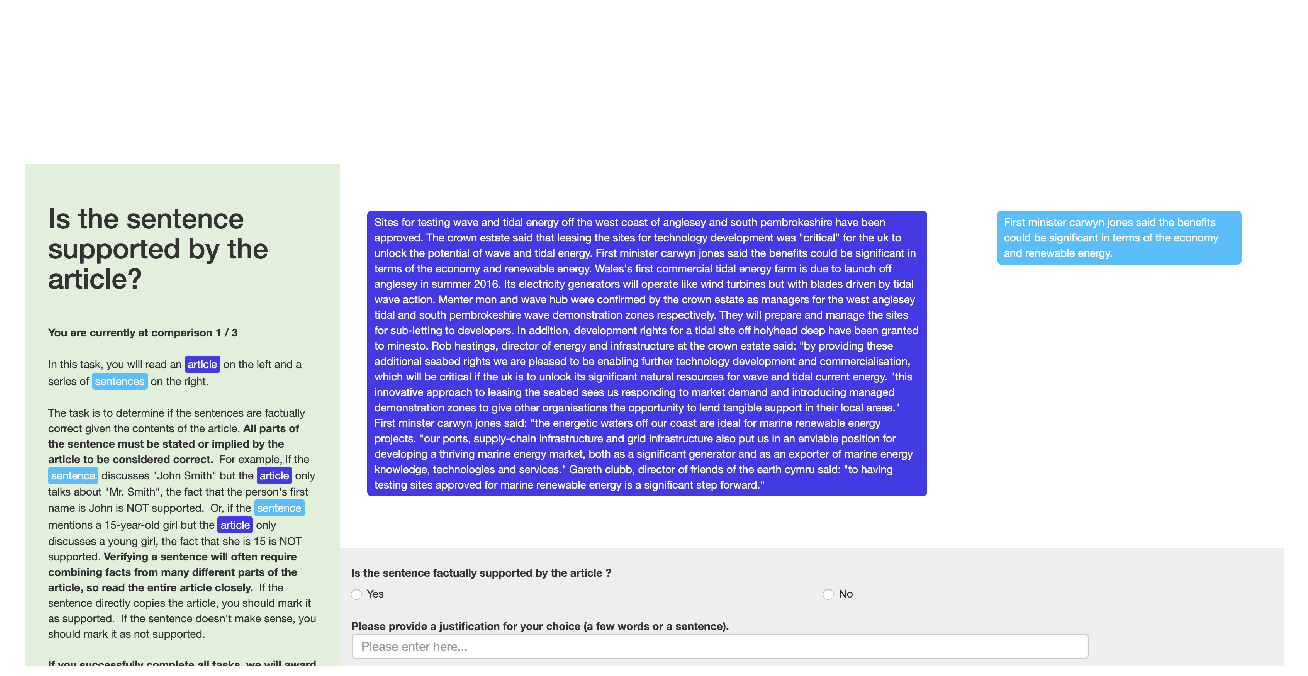