ESPnet-ST: All-in-One Speech Translation Toolkit
Hirofumi Inaguma, Shun Kiyono, Kevin Duh, Shigeki Karita, Nelson Yalta, Tomoki Hayashi, Shinji Watanabe
System Demonstrations Demo Paper
Demo Session 1B-3: Jul 8
(05:45-06:45 GMT)
Demo Session 3A-3: Jul 8
(12:00-13:00 GMT)
Abstract:
We present ESPnet-ST, which is designed for the quick development of speech-to-speech translation systems in a single framework. ESPnet-ST is a new project inside end-to-end speech processing toolkit, ESPnet, which integrates or newly implements automatic speech recognition, machine translation, and text-to-speech functions for speech translation. We provide all-in-one recipes including data pre-processing, feature extraction, training, and decoding pipelines for a wide range of benchmark datasets. Our reproducible results can match or even outperform the current state-of-the-art performances; these pre-trained models are downloadable. The toolkit is publicly available at https://github.com/espnet/espnet.
You can open the
pre-recorded video
in a separate window.
NOTE: The SlidesLive video may display a random order of the authors.
The correct author list is shown at the top of this webpage.
Similar Papers
Learning Spoken Language Representations with Neural Lattice Language Modeling
Chao-Wei Huang, Yun-Nung Chen,
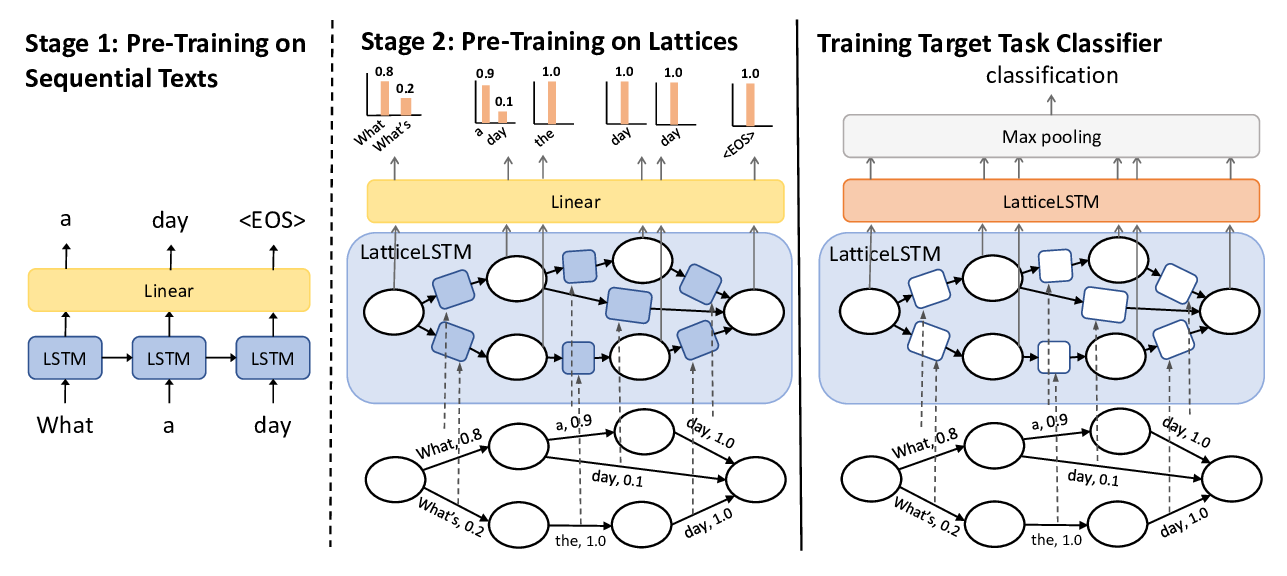
Stanza: A Python Natural Language Processing Toolkit for Many Human Languages
Peng Qi, Yuhao Zhang, Yuhui Zhang, Jason Bolton, Christopher D. Manning,
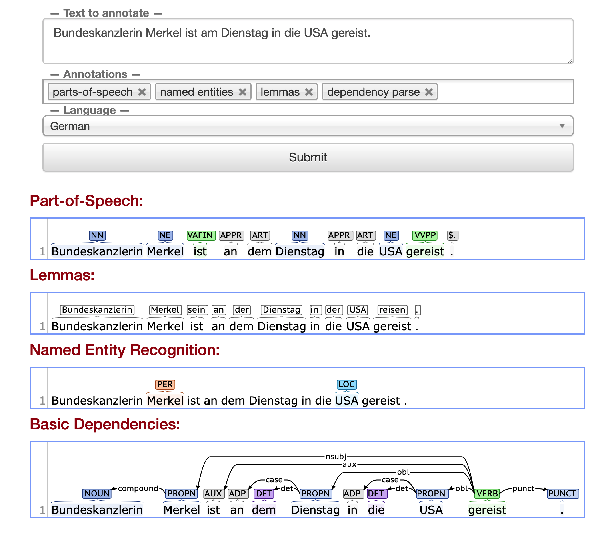
Personalized PageRank with Syntagmatic Information for Multilingual Word Sense Disambiguation
Federico Scozzafava, Marco Maru, Fabrizio Brignone, Giovanni Torrisi, Roberto Navigli,
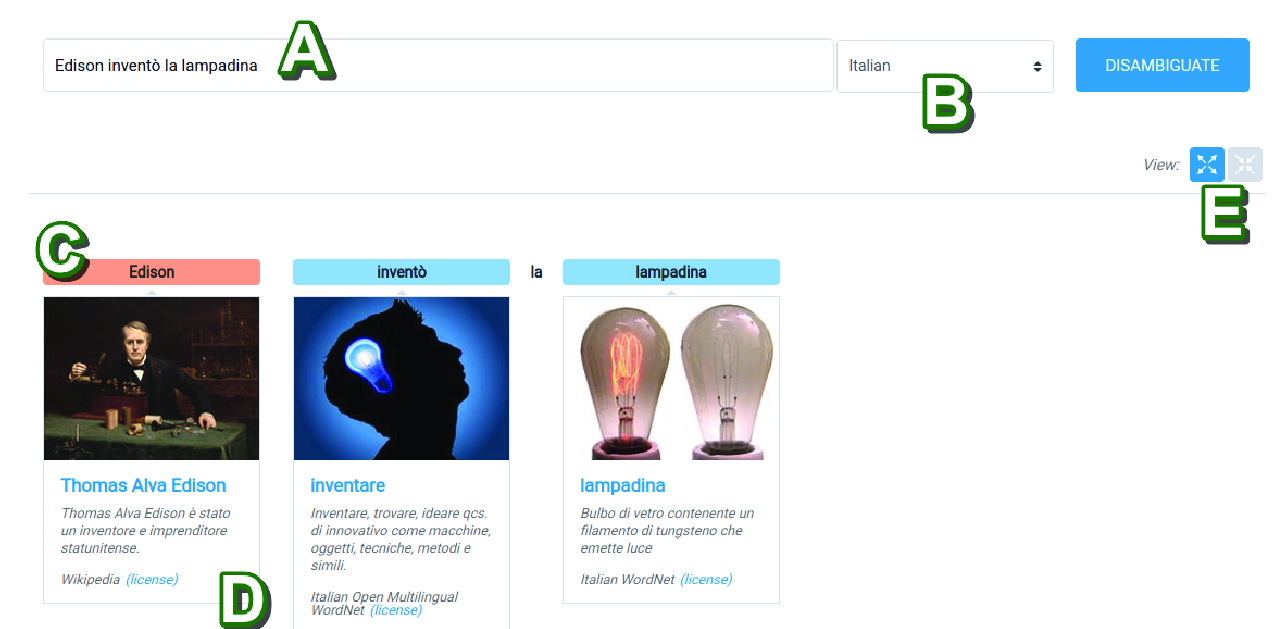
Encoder-Decoder Models Can Benefit from Pre-trained Masked Language Models in Grammatical Error Correction
Masahiro Kaneko, Masato Mita, Shun Kiyono, Jun Suzuki, Kentaro Inui,
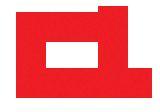