Dynamic Memory Induction Networks for Few-Shot Text Classification
Ruiying Geng, Binhua Li, Yongbin Li, Jian Sun, Xiaodan Zhu
Information Retrieval and Text Mining Short Paper
Session 2A: Jul 6
(08:00-09:00 GMT)
Session 3A: Jul 6
(12:00-13:00 GMT)
Abstract:
This paper proposes Dynamic Memory Induction Networks (DMIN) for few-short text classification. The model develops a dynamic routing mechanism over static memory, enabling it to better adapt to unseen classes, a critical capability for few-short classification. The model also expands the induction process with supervised learning weights and query information to enhance the generalization ability of meta-learning. The proposed model brings forward the state-of-the-art performance significantly by 2~4% improvement on the miniRCV1 and ODIC datasets. Detailed analysis is further performed to show how the proposed network achieves the new performance.
You can open the
pre-recorded video
in a separate window.
NOTE: The SlidesLive video may display a random order of the authors.
The correct author list is shown at the top of this webpage.
Similar Papers
Few-shot Slot Tagging with Collapsed Dependency Transfer and Label-enhanced Task-adaptive Projection Network
Yutai Hou, Wanxiang Che, Yongkui Lai, Zhihan Zhou, Yijia Liu, Han Liu, Ting Liu,
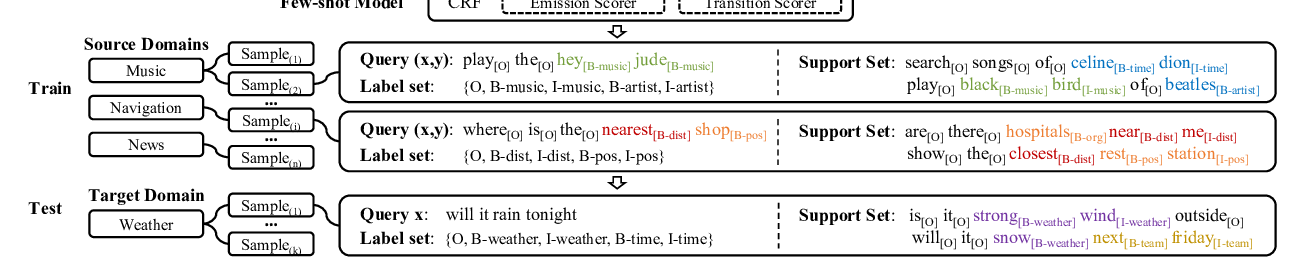
PeTra: A Sparsely Supervised Memory Model for People Tracking
Shubham Toshniwal, Allyson Ettinger, Kevin Gimpel, Karen Livescu,
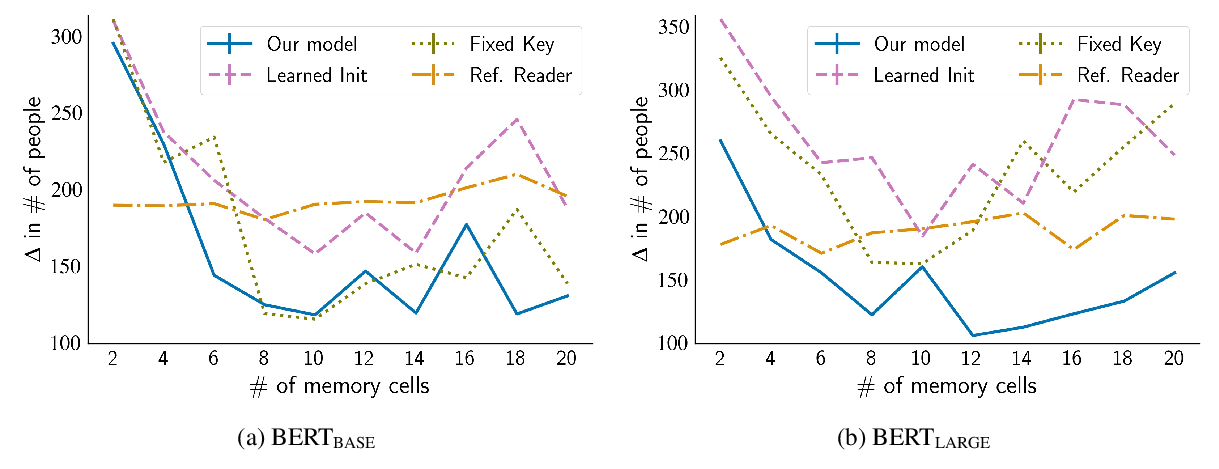
Learning to Tag OOV Tokens by Integrating Contextual Representation and Background Knowledge
Keqing He, Yuanmeng Yan, Weiran XU,
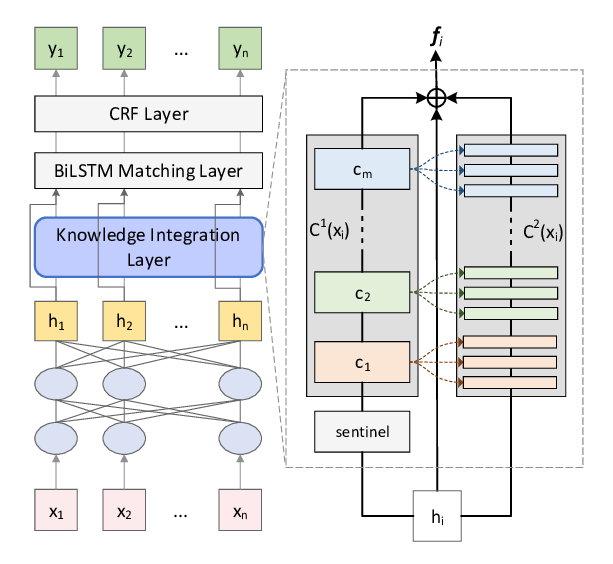
Unknown Intent Detection Using Gaussian Mixture Model with an Application to Zero-shot Intent Classification
Guangfeng Yan, Lu Fan, Qimai Li, Han Liu, Xiaotong Zhang, Xiao-Ming Wu, Albert Y.S. Lam,
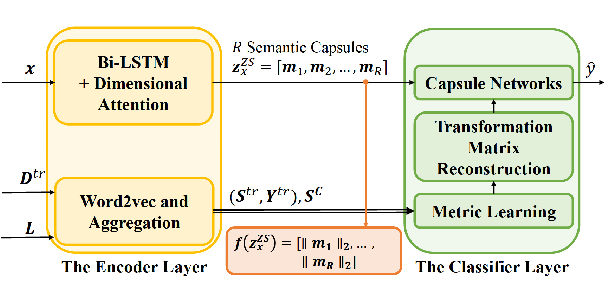