PeTra: A Sparsely Supervised Memory Model for People Tracking
Shubham Toshniwal, Allyson Ettinger, Kevin Gimpel, Karen Livescu
Discourse and Pragmatics Long Paper
Session 9B: Jul 7
(18:00-19:00 GMT)
Session 10B: Jul 7
(21:00-22:00 GMT)
Abstract:
We propose PeTra, a memory-augmented neural network designed to track entities in its memory slots. PeTra is trained using sparse annotation from the GAP pronoun resolution dataset and outperforms a prior memory model on the task while using a simpler architecture. We empirically compare key modeling choices, finding that we can simplify several aspects of the design of the memory module while retaining strong performance. To measure the people tracking capability of memory models, we (a) propose a new diagnostic evaluation based on counting the number of unique entities in text, and (b) conduct a small scale human evaluation to compare evidence of people tracking in the memory logs of PeTra relative to a previous approach. PeTra is highly effective in both evaluations, demonstrating its ability to track people in its memory despite being trained with limited annotation.
You can open the
pre-recorded video
in a separate window.
NOTE: The SlidesLive video may display a random order of the authors.
The correct author list is shown at the top of this webpage.
Similar Papers
MART: Memory-Augmented Recurrent Transformer for Coherent Video Paragraph Captioning
Jie Lei, Liwei Wang, Yelong Shen, Dong Yu, Tamara Berg, Mohit Bansal,
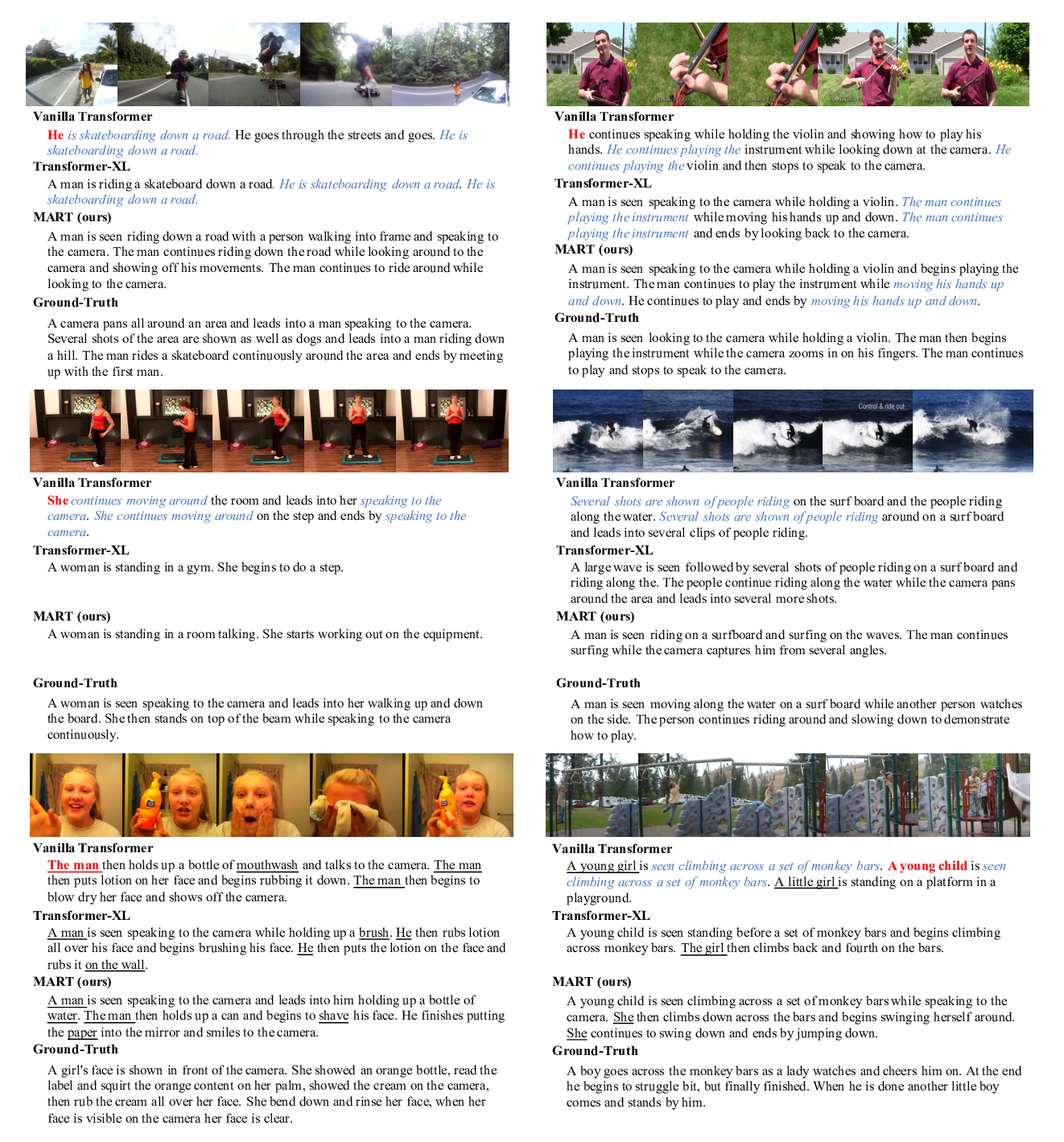
Response-Anticipated Memory for On-Demand Knowledge Integration in Response Generation
Zhiliang Tian, Wei Bi, Dongkyu Lee, Lanqing Xue, Yiping Song, Xiaojiang Liu, Nevin L. Zhang,
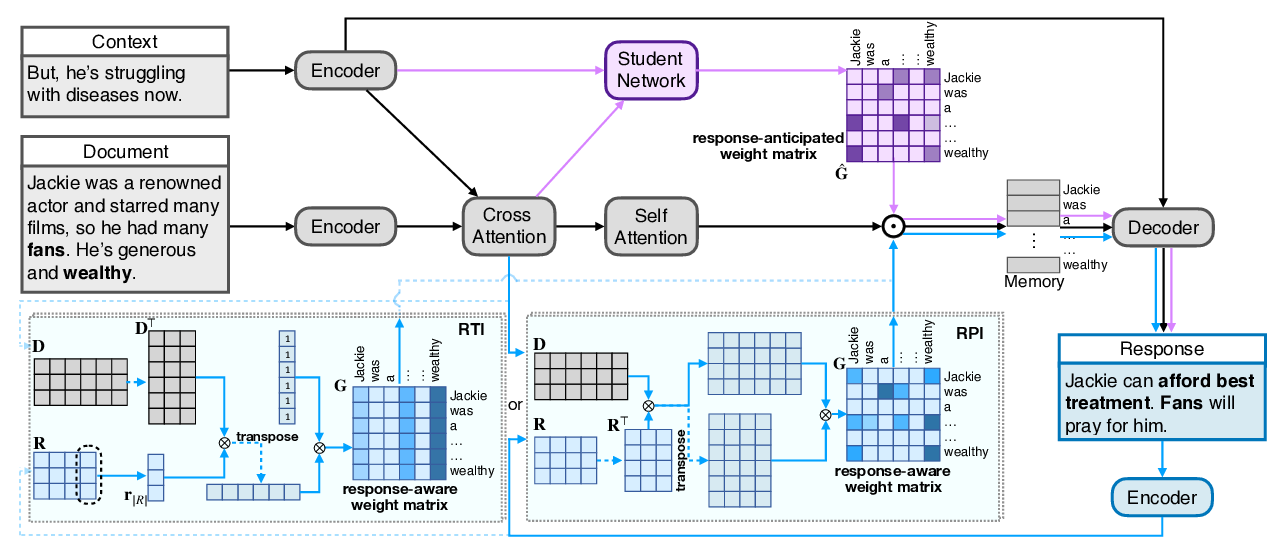
Efficient Dialogue State Tracking by Selectively Overwriting Memory
Sungdong Kim, Sohee Yang, Gyuwan Kim, Sang-Woo Lee,
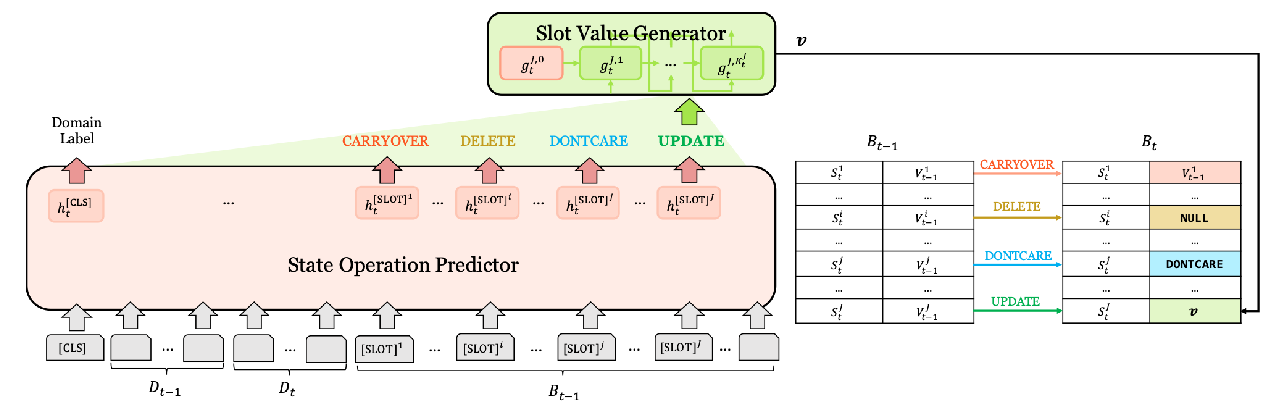