Exclusive Hierarchical Decoding for Deep Keyphrase Generation
Wang Chen, Hou Pong Chan, Piji Li, Irwin King
Information Retrieval and Text Mining Long Paper
Session 2A: Jul 6
(08:00-09:00 GMT)
Session 3A: Jul 6
(12:00-13:00 GMT)
Abstract:
Keyphrase generation (KG) aims to summarize the main ideas of a document into a set of keyphrases. A new setting is recently introduced into this problem, in which, given a document, the model needs to predict a set of keyphrases and simultaneously determine the appropriate number of keyphrases to produce. Previous work in this setting employs a sequential decoding process to generate keyphrases. However, such a decoding method ignores the intrinsic hierarchical compositionality existing in the keyphrase set of a document. Moreover, previous work tends to generate duplicated keyphrases, which wastes time and computing resources. To overcome these limitations, we propose an exclusive hierarchical decoding framework that includes a hierarchical decoding process and either a soft or a hard exclusion mechanism. The hierarchical decoding process is to explicitly model the hierarchical compositionality of a keyphrase set. Both the soft and the hard exclusion mechanisms keep track of previously-predicted keyphrases within a window size to enhance the diversity of the generated keyphrases. Extensive experiments on multiple KG benchmark datasets demonstrate the effectiveness of our method to generate less duplicated and more accurate keyphrases.
You can open the
pre-recorded video
in a separate window.
NOTE: The SlidesLive video may display a random order of the authors.
The correct author list is shown at the top of this webpage.
Similar Papers
One Size Does Not Fit All: Generating and Evaluating Variable Number of Keyphrases
Xingdi Yuan, Tong Wang, Rui Meng, Khushboo Thaker, Peter Brusilovsky, Daqing He, Adam Trischler,
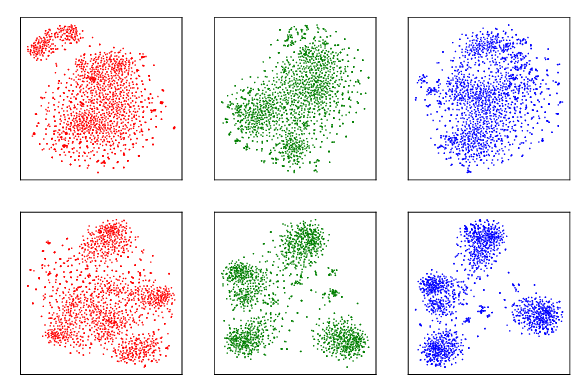
CluHTM - Semantic Hierarchical Topic Modeling based on CluWords
Felipe Viegas, Washington Cunha, Christian Gomes, Antônio Pereira, Leonardo Rocha, Marcos Goncalves,
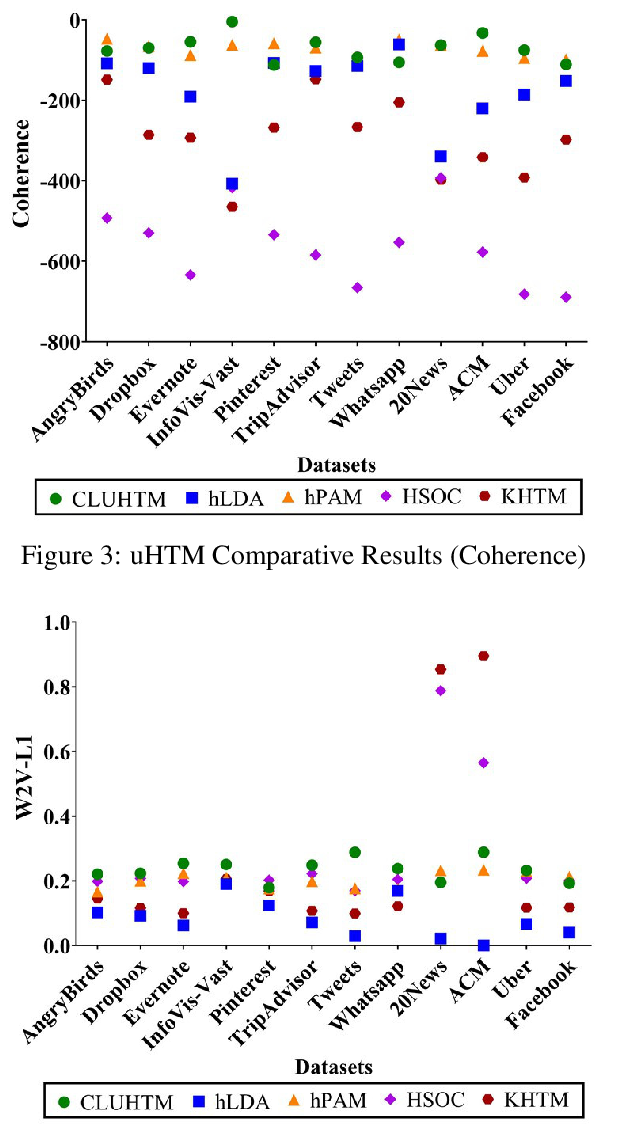
Low-Dimensional Hyperbolic Knowledge Graph Embeddings
Ines Chami, Adva Wolf, Da-Cheng Juan, Frederic Sala, Sujith Ravi, Christopher Ré,
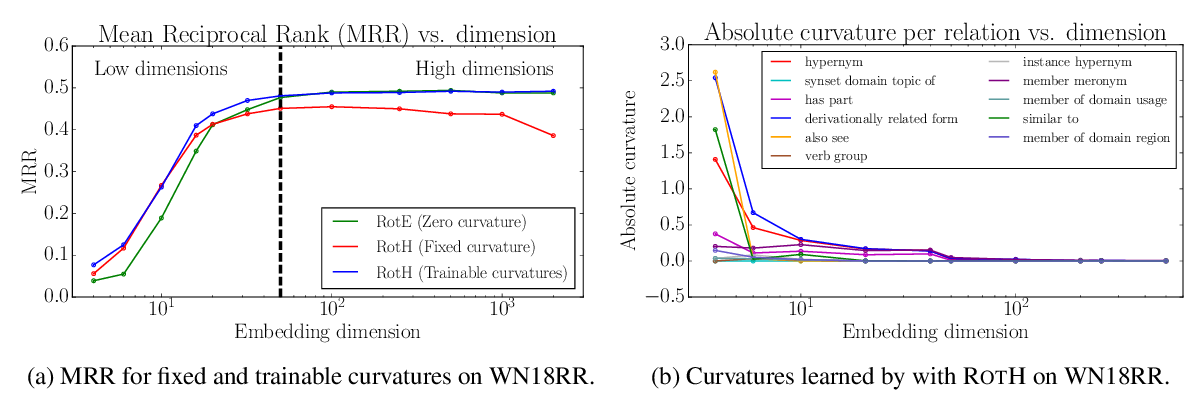