CluHTM - Semantic Hierarchical Topic Modeling based on CluWords
Felipe Viegas, Washington Cunha, Christian Gomes, Antônio Pereira, Leonardo Rocha, Marcos Goncalves
Information Retrieval and Text Mining Long Paper
Session 14A: Jul 8
(17:00-18:00 GMT)
Session 15A: Jul 8
(20:00-21:00 GMT)
Abstract:
Hierarchical Topic modeling (HTM) exploits latent topics and relationships among them as a powerful tool for data analysis and exploration. Despite advantages over traditional topic modeling, HTM poses its own challenges, such as (1) topic incoherence, (2) unreasonable (hierarchical) structure, and (3) issues related to the definition of the ``ideal'' number of topics and depth of the hierarchy. In this paper, we advance the state-of-the-art on HTM by means of the design and evaluation of CluHTM, a novel non-probabilistic hierarchical matrix factorization aimed at solving the specific issues of HTM. CluHTM's novel contributions include: (i) the exploration of richer text representation that encapsulates both, global (dataset level) and local semantic information -- when combined, these pieces of information help to solve the topic incoherence problem as well as issues related to the unreasonable structure; (ii) the exploitation of a stability analysis metric for defining the number of topics and the ``shape'' the hierarchical structure. In our evaluation, considering twelve datasets and seven state-of-the-art baselines, CluHTM outperformed the baselines in the vast majority of the cases, with gains of around 500% over the strongest state-of-the-art baselines. We also provide qualitative and quantitative statistical analyses of why our solution works so well.
You can open the
pre-recorded video
in a separate window.
NOTE: The SlidesLive video may display a random order of the authors.
The correct author list is shown at the top of this webpage.
Similar Papers
Neural Topic Modeling with Bidirectional Adversarial Training
Rui Wang, Xuemeng Hu, Deyu Zhou, Yulan He, Yuxuan Xiong, Chenchen Ye, Haiyang Xu,
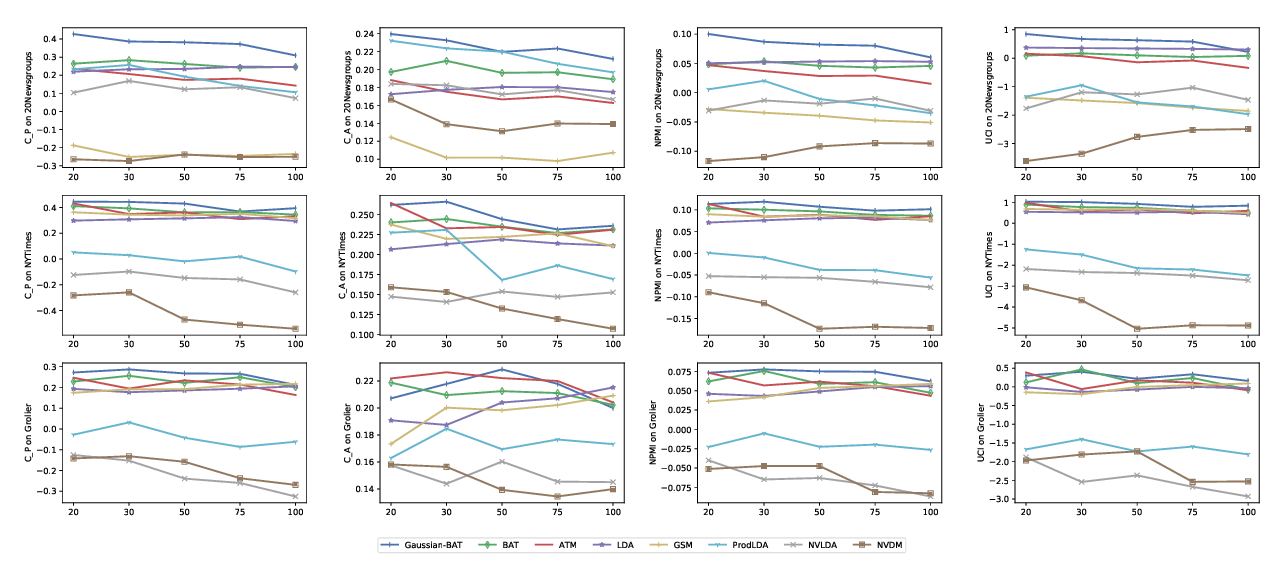
Exclusive Hierarchical Decoding for Deep Keyphrase Generation
Wang Chen, Hou Pong Chan, Piji Li, Irwin King,
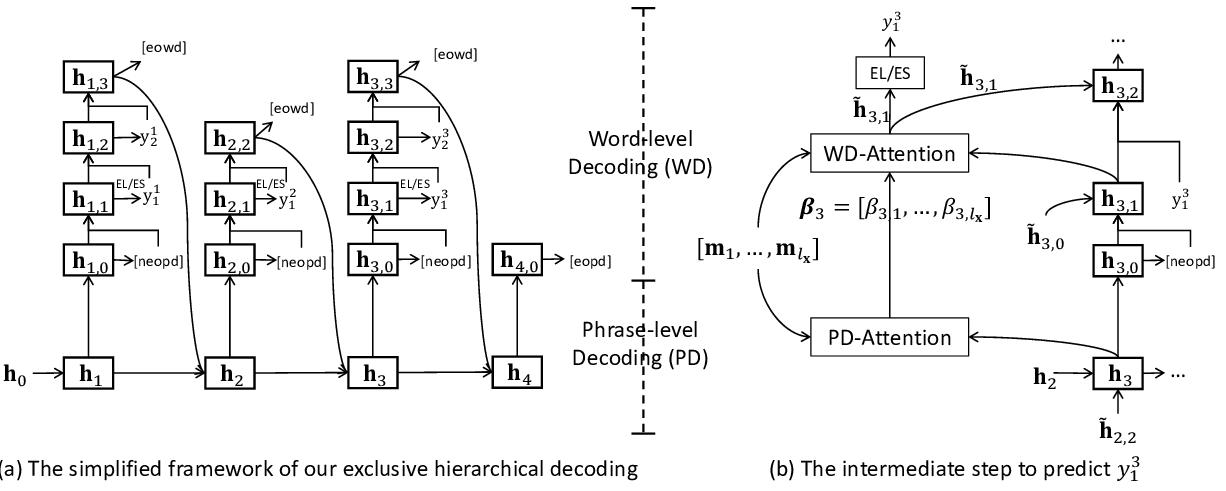
Explicit Semantic Decomposition for Definition Generation
Jiahuan Li, Yu Bao, Shujian Huang, Xinyu Dai, Jiajun Chen,
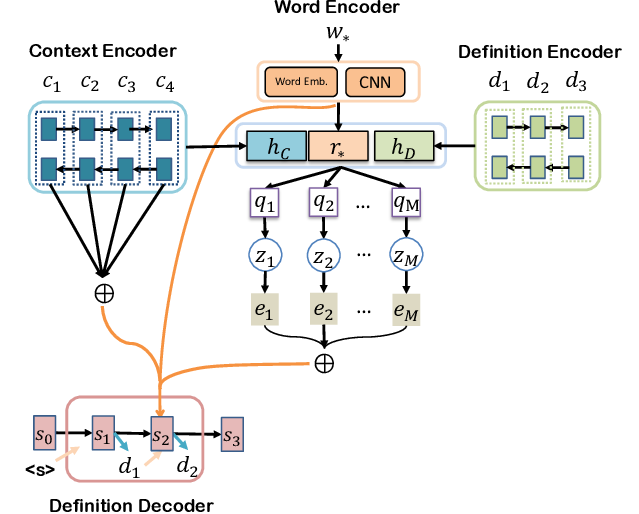
Hierarchy-Aware Global Model for Hierarchical Text Classification
Jie Zhou, Chunping Ma, Dingkun Long, Guangwei Xu, Ning Ding, Haoyu Zhang, Pengjun Xie, Gongshen Liu,
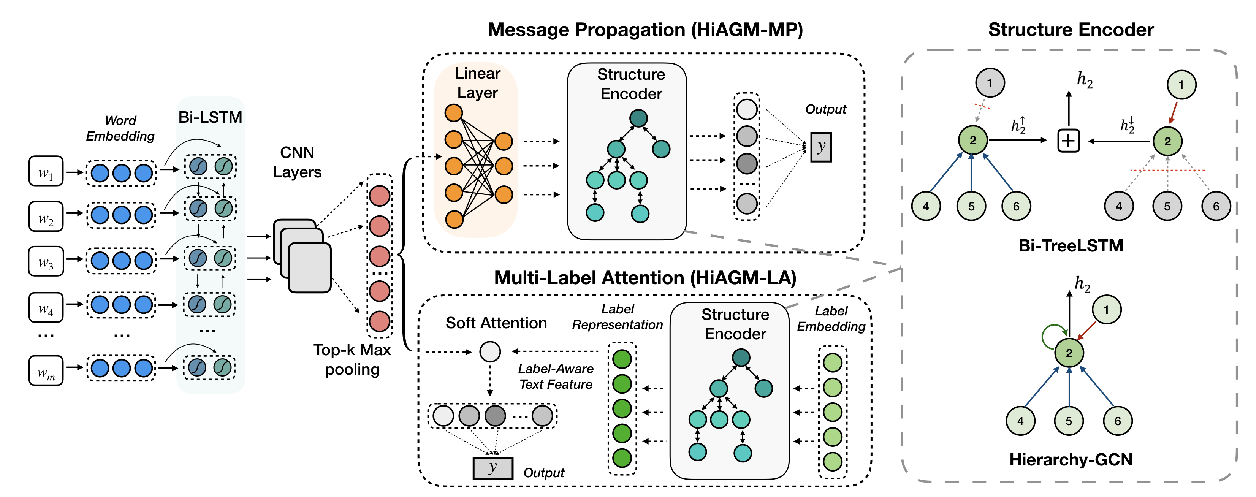