Hierarchy-Aware Global Model for Hierarchical Text Classification
Jie Zhou, Chunping Ma, Dingkun Long, Guangwei Xu, Ning Ding, Haoyu Zhang, Pengjun Xie, Gongshen Liu
Information Retrieval and Text Mining Long Paper
Session 2A: Jul 6
(08:00-09:00 GMT)
Session 3A: Jul 6
(12:00-13:00 GMT)
Abstract:
Hierarchical text classification is an essential yet challenging subtask of multi-label text classification with a taxonomic hierarchy. Existing methods have difficulties in modeling the hierarchical label structure in a global view. Furthermore, they cannot make full use of the mutual interactions between the text feature space and the label space. In this paper, we formulate the hierarchy as a directed graph and introduce hierarchy-aware structure encoders for modeling label dependencies. Based on the hierarchy encoder, we propose a novel end-to-end hierarchy-aware global model (HiAGM) with two variants. A multi-label attention variant (HiAGM-LA) learns hierarchy-aware label embeddings through the hierarchy encoder and conducts inductive fusion of label-aware text features. A text feature propagation model (HiAGM-TP) is proposed as the deductive variant that directly feeds text features into hierarchy encoders. Compared with previous works, both HiAGM-LA and HiAGM-TP achieve significant and consistent improvements on three benchmark datasets.
You can open the
pre-recorded video
in a separate window.
NOTE: The SlidesLive video may display a random order of the authors.
The correct author list is shown at the top of this webpage.
Similar Papers
Learning to Tag OOV Tokens by Integrating Contextual Representation and Background Knowledge
Keqing He, Yuanmeng Yan, Weiran XU,
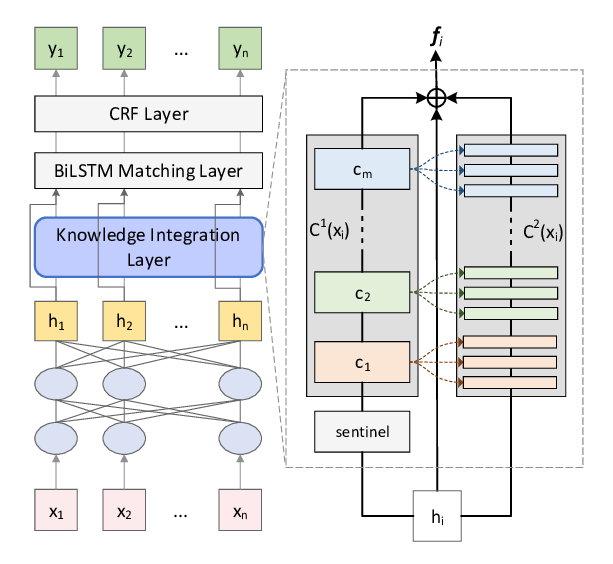
TAG : Type Auxiliary Guiding for Code Comment Generation
Ruichu Cai, Zhihao Liang, Boyan Xu, zijian li, Yuexing Hao, Yao Chen,
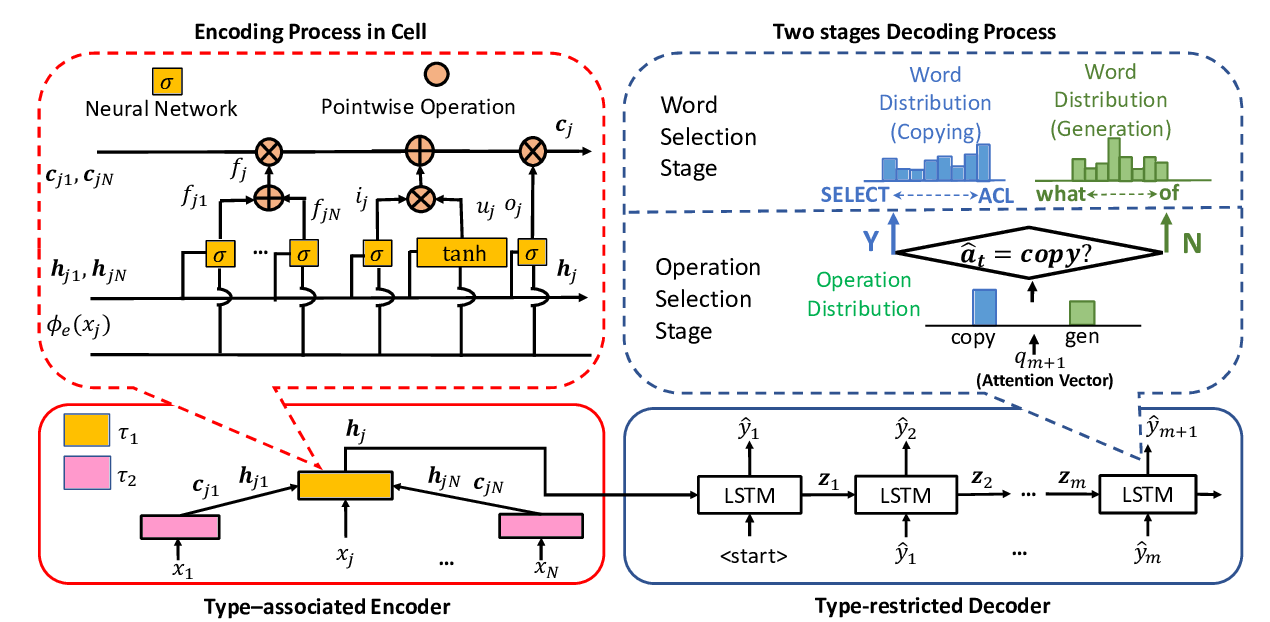
Hyperbolic Capsule Networks for Multi-Label Classification
Boli Chen, Xin Huang, Lin Xiao, Liping Jing,
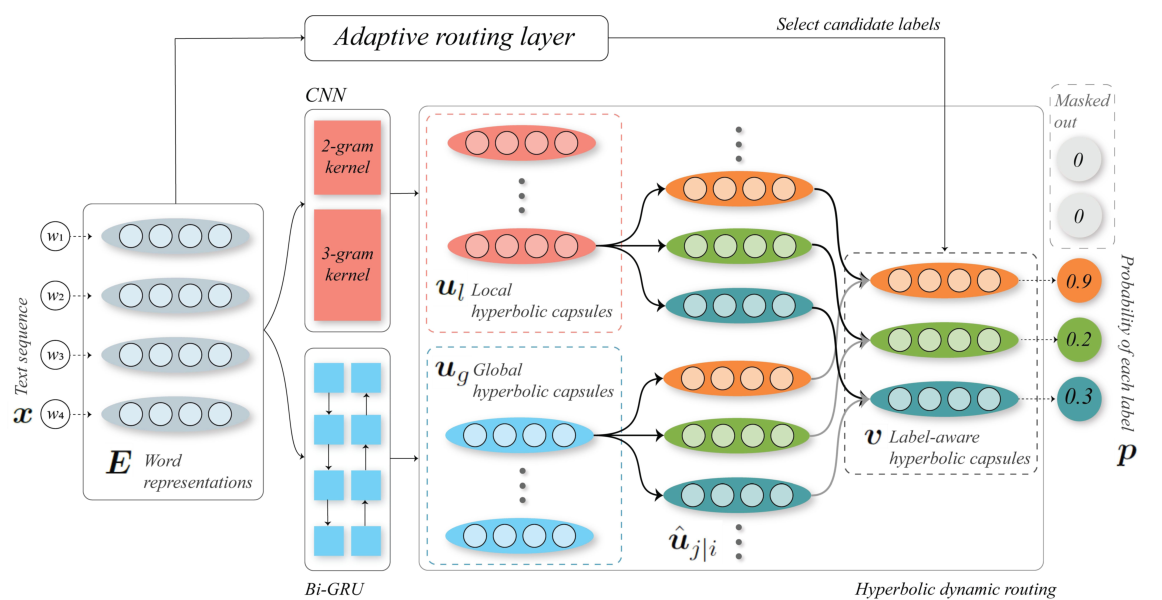
Few-shot Slot Tagging with Collapsed Dependency Transfer and Label-enhanced Task-adaptive Projection Network
Yutai Hou, Wanxiang Che, Yongkui Lai, Zhihan Zhou, Yijia Liu, Han Liu, Ting Liu,
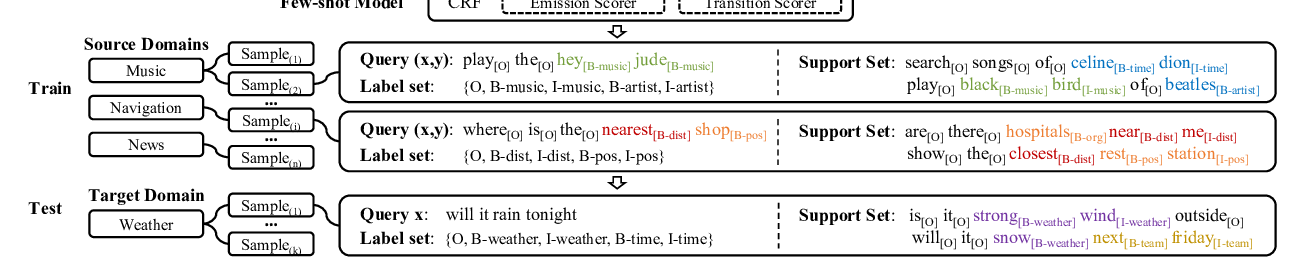