Abstract:
We propose a new end-to-end model that treats AMR parsing as a series of dual decisions on the input sequence and the incrementally constructed graph. At each time step, our model performs multiple rounds of attention, reasoning, and composition that aim to answer two critical questions: (1) which part of the input sequence to abstract; and (2) where in the output graph to construct the new concept. We show that the answers to these two questions are mutually causalities. We design a model based on iterative inference that helps achieve better answers in both perspectives, leading to greatly improved parsing accuracy. Our experimental results significantly outperform all previously reported Smatch scores by large margins. Remarkably, without the help of any large-scale pre-trained language model (e.g., BERT), our model already surpasses previous state-of-the-art using BERT. With the help of BERT, we can push the state-of-the-art results to 80.2% on LDC2017T10 (AMR 2.0) and 75.4% on LDC2014T12 (AMR 1.0).
You can open the
pre-recorded video
in a separate window.
NOTE: The SlidesLive video may display a random order of the authors.
The correct author list is shown at the top of this webpage.
Similar Papers
GPT-too: A Language-Model-First Approach for AMR-to-Text Generation
Manuel Mager, Ramón Fernandez Astudillo, Tahira Naseem, Md Arafat Sultan, Young-Suk Lee, Radu Florian, Salim Roukos,
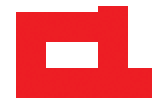
Line Graph Enhanced AMR-to-Text Generation with Mix-Order Graph Attention Networks
Yanbin Zhao, Lu Chen, Zhi Chen, Ruisheng Cao, Su Zhu, Kai Yu,
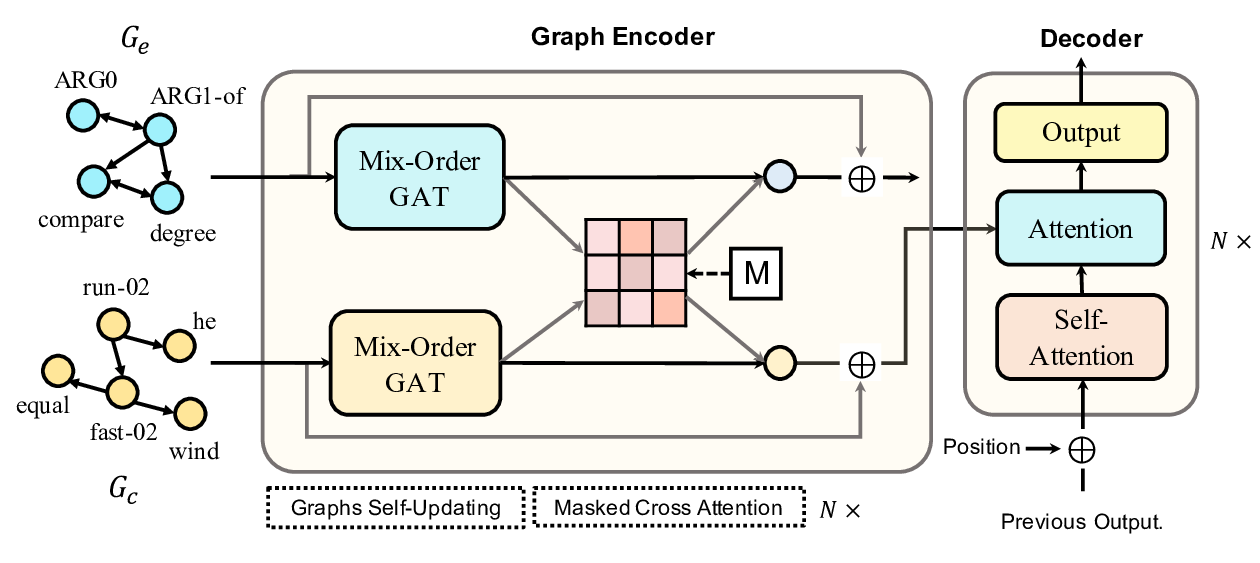