GPT-too: A Language-Model-First Approach for AMR-to-Text Generation
Manuel Mager, Ramón Fernandez Astudillo, Tahira Naseem, Md Arafat Sultan, Young-Suk Lee, Radu Florian, Salim Roukos
Generation Short Paper
Session 3B: Jul 6
(13:00-14:00 GMT)
Session 4B: Jul 6
(18:00-19:00 GMT)
Abstract:
Abstract Meaning Representations (AMRs) are broad-coverage sentence-level semantic graphs. Existing approaches to generating text from AMR have focused on training sequence-to-sequence or graph-to-sequence models on AMR annotated data only. In this paper, we propose an alternative approach that combines a strong pre-trained language model with cycle consistency-based re-scoring. Despite the simplicity of the approach, our experimental results show these models outperform all previous techniques on the English LDC2017T10 dataset, including the recent use of transformer architectures. In addition to the standard evaluation metrics, we provide human evaluation experiments that further substantiate the strength of our approach.
You can open the
pre-recorded video
in a separate window.
NOTE: The SlidesLive video may display a random order of the authors.
The correct author list is shown at the top of this webpage.
Similar Papers
Line Graph Enhanced AMR-to-Text Generation with Mix-Order Graph Attention Networks
Yanbin Zhao, Lu Chen, Zhi Chen, Ruisheng Cao, Su Zhu, Kai Yu,
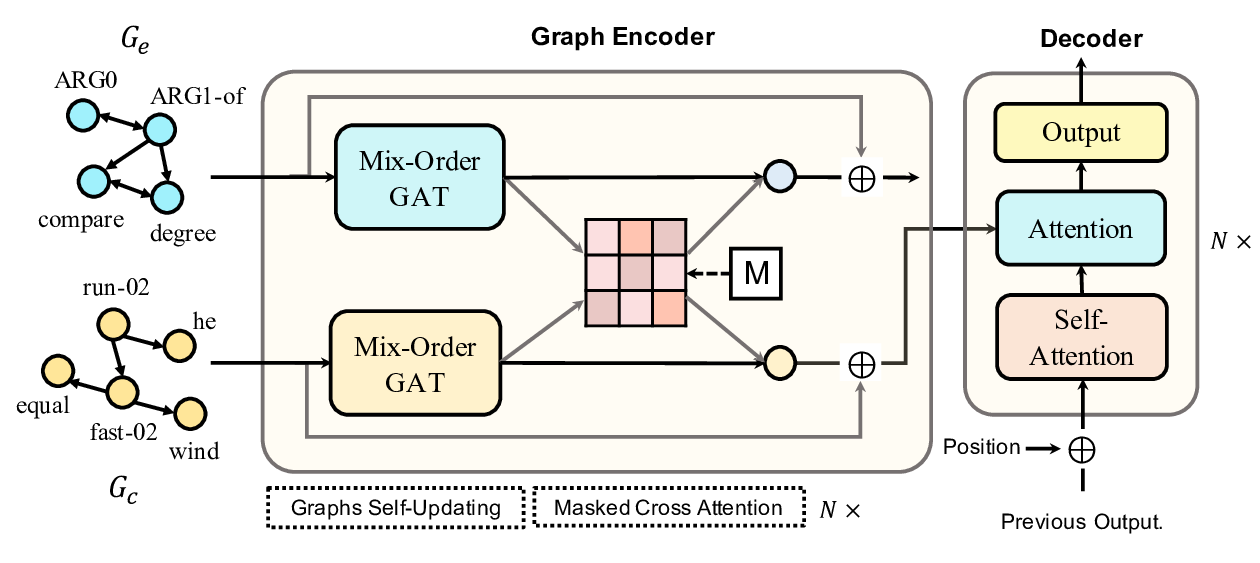