AMR-To-Text Generation with Graph Transformer
Tianming Wang, Xiaojun Wan, Hanqi Jin
Semantics: Sentence Level TACL Paper
Session 11B: Jul 8
(06:00-07:00 GMT)
Session 12A: Jul 8
(08:00-09:00 GMT)
Abstract:
Abstract meaning representation (AMR)-to-text generation is the challenging task of generating natural language texts from AMR graphs, where nodes represent concepts and edges denote relations. The current state-of-the-art methods use graph-to-sequence models; however, they still cannot significantly outperform the previous sequence-to-sequence models or statistical approaches. In this paper, we propose a novel graph-to-sequence model (Graph Transformer) to address the above-mentioned task. The model directly encodes the AMR graphs and learns the node representations. A pairwise interaction function is used for computing the semantic relations between the concepts. Moreover, attention mechanisms are employed for aggregating the information from the incoming and outgoing neighbors, which help the model to capture the semantic information effectively. Our model outperforms the state-of-the-art neural approach by 1.5 BLEU points on LDC2015E86 and 4.8 BLEU points on LDC2017T10 and achieves new state-of-the-art performances.
You can open the
pre-recorded video
in a separate window.
NOTE: The SlidesLive video may display a random order of the authors.
The correct author list is shown at the top of this webpage.
Similar Papers
Line Graph Enhanced AMR-to-Text Generation with Mix-Order Graph Attention Networks
Yanbin Zhao, Lu Chen, Zhi Chen, Ruisheng Cao, Su Zhu, Kai Yu,
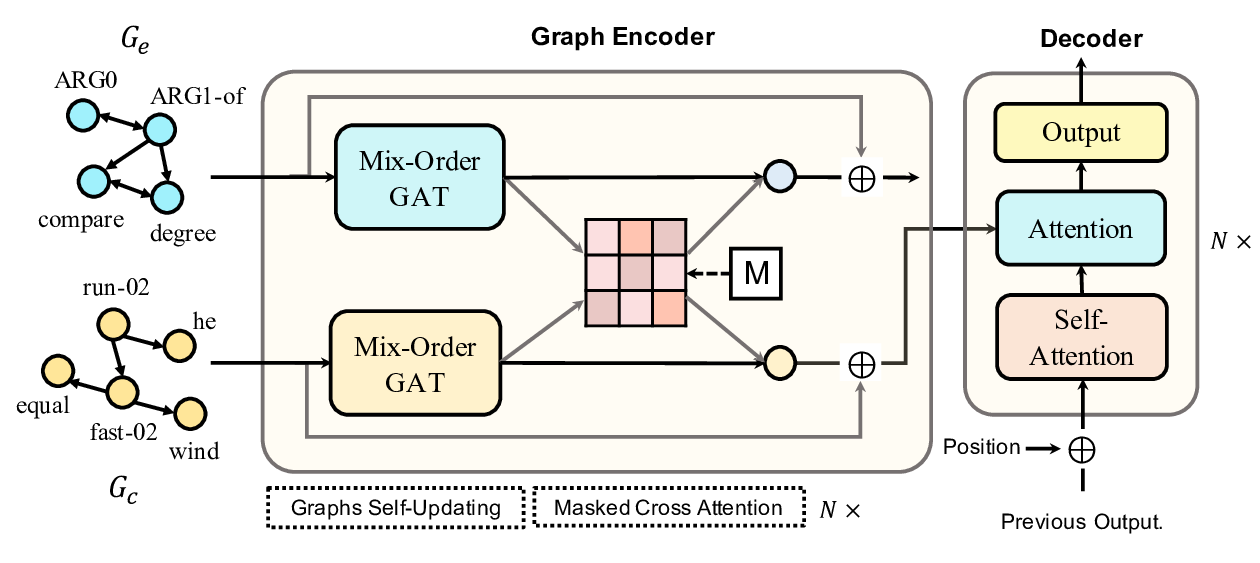
GPT-too: A Language-Model-First Approach for AMR-to-Text Generation
Manuel Mager, Ramón Fernandez Astudillo, Tahira Naseem, Md Arafat Sultan, Young-Suk Lee, Radu Florian, Salim Roukos,
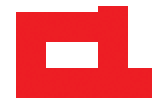
Heterogeneous Graph Transformer for Graph-to-Sequence Learning
Shaowei Yao, Tianming Wang, Xiaojun Wan,
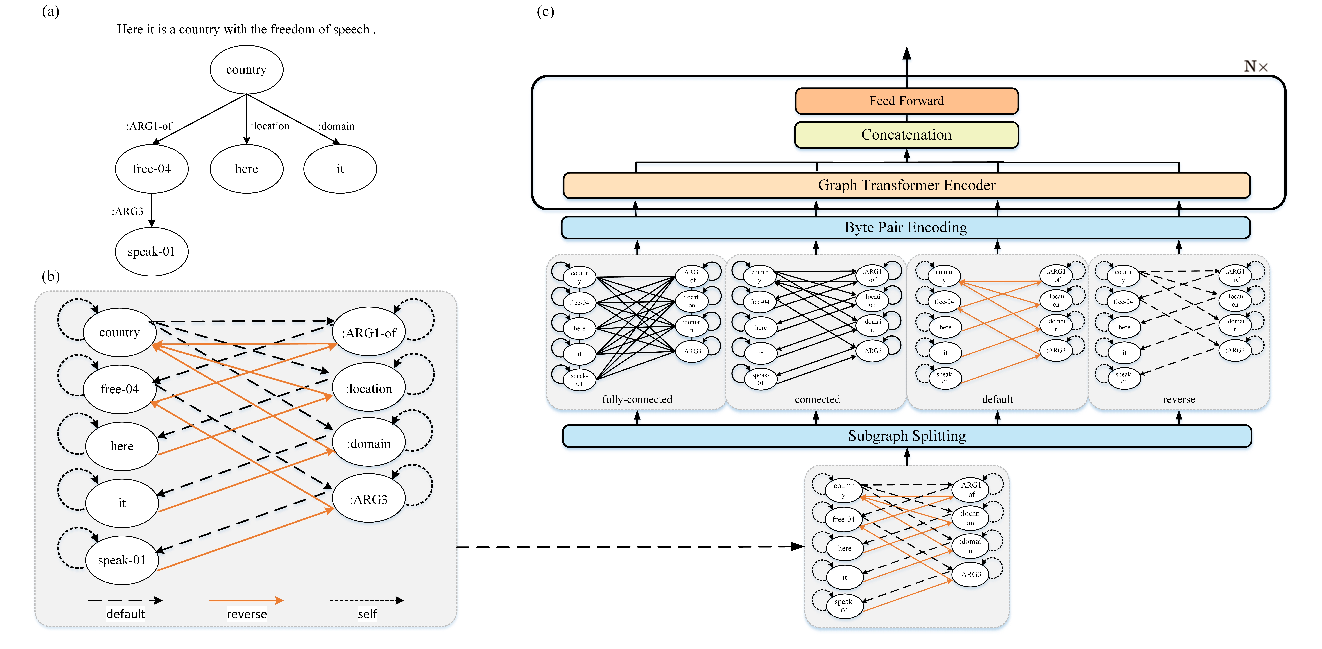