Examining the State-of-the-Art in News Timeline Summarization
Demian Gholipour Ghalandari, Georgiana Ifrim
Summarization Long Paper
Session 2A: Jul 6
(08:00-09:00 GMT)
Session 3B: Jul 6
(13:00-14:00 GMT)
Abstract:
Previous work on automatic news timeline summarization (TLS) leaves an unclear picture about how this task can generally be approached and how well it is currently solved. This is mostly due to the focus on individual subtasks, such as date selection and date summarization, and to the previous lack of appropriate evaluation metrics for the full TLS task. In this paper, we compare different TLS strategies using appropriate evaluation frameworks, and propose a simple and effective combination of methods that improves over the stateof-the-art on all tested benchmarks. For a more robust evaluation, we also present a new TLS dataset, which is larger and spans longer time periods than previous datasets.
You can open the
pre-recorded video
in a separate window.
NOTE: The SlidesLive video may display a random order of the authors.
The correct author list is shown at the top of this webpage.
Similar Papers
Estimating the influence of auxiliary tasks for multi-task learning of sequence tagging tasks
Fynn Schröder, Chris Biemann,
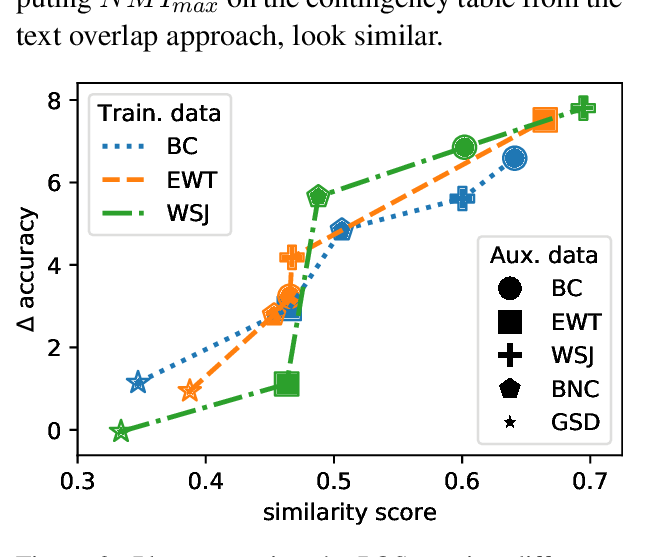
SpanBERT: Improving Pre-training by Representing and Predicting Spans
Mandar Joshi, Danqi Chen, Yinhan Liu, Daniel S. Weld, Luke Zettlemoyer, Omer Levy,
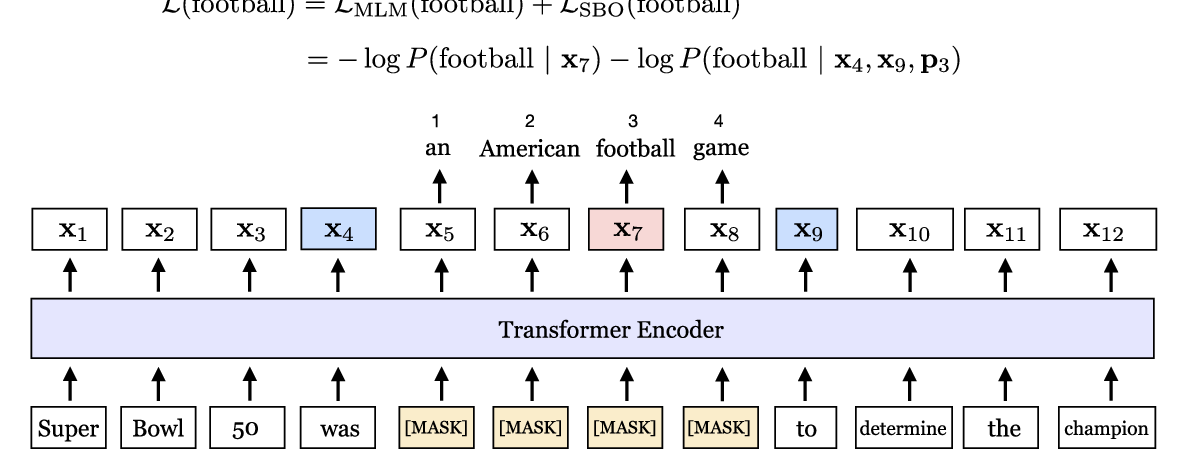
Implicit Discourse Relation Classification: We Need to Talk about Evaluation
Najoung Kim, Song Feng, Chulaka Gunasekara, Luis Lastras,
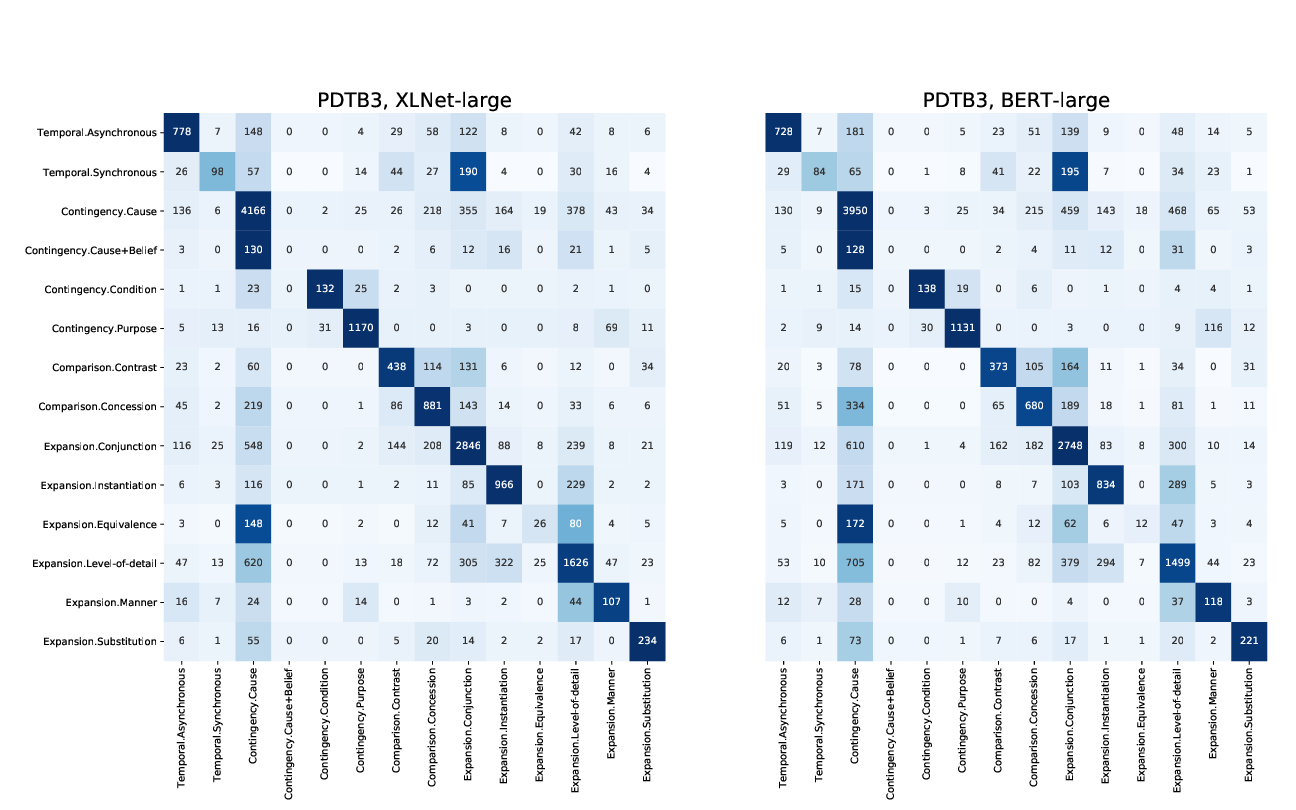
One Size Does Not Fit All: Generating and Evaluating Variable Number of Keyphrases
Xingdi Yuan, Tong Wang, Rui Meng, Khushboo Thaker, Peter Brusilovsky, Daqing He, Adam Trischler,
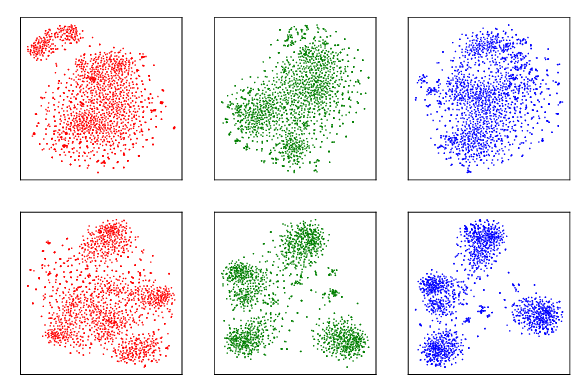