SpanBERT: Improving Pre-training by Representing and Predicting Spans
Mandar Joshi, Danqi Chen, Yinhan Liu, Daniel S. Weld, Luke Zettlemoyer, Omer Levy
Machine Learning for NLP TACL Paper
Session 9A: Jul 7
(17:00-18:00 GMT)
Session 10B: Jul 7
(21:00-22:00 GMT)
Abstract:
We present SpanBERT, a pre-training method that is designed to better represent and predict spans of text. Our approach extends BERT by (1) masking contiguous random spans, rather than random tokens, and (2) training the span boundary representations to predict the entire content of the masked span, without relying on the individual token representations within it. SpanBERT consistently outperforms BERT and our better-tuned baselines, with substantial gains on span selection tasks such as question answering and coreference resolution. In particular, with the same training data and model size as BERT-Large, our single model obtains 94.6% and 88.7% F1 on SQuAD 1.1 and 2.0 respectively. We also achieve a new state of the art on the OntoNotes coreference resolution task (79.6% F1), strong performance on the TACRED relation extraction benchmark, and even gains on GLUE.
You can open the
pre-recorded video
in a separate window.
NOTE: The SlidesLive video may display a random order of the authors.
The correct author list is shown at the top of this webpage.
Similar Papers
Span Selection Pre-training for Question Answering
Michael Glass, Alfio Gliozzo, Rishav Chakravarti, Anthony Ferritto, Lin Pan, G P Shrivatsa Bhargav, Dinesh Garg, Avi Sil,
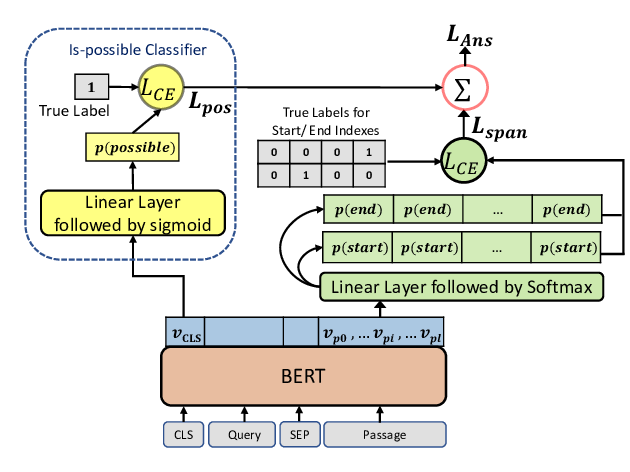
Span-ConveRT: Few-shot Span Extraction for Dialog with Pretrained Conversational Representations
Samuel Coope, Tyler Farghly, Daniela Gerz, Ivan Vulić, Matthew Henderson,
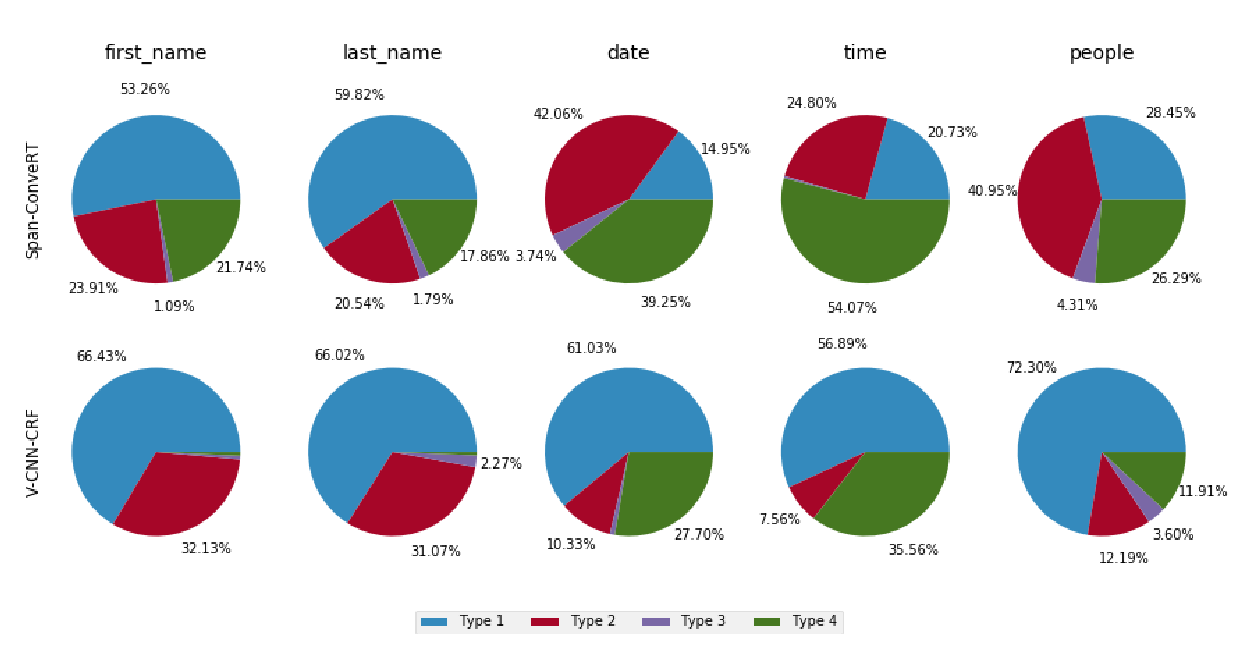
CorefQA: Coreference Resolution as Query-based Span Prediction
Wei Wu, Fei Wang, Arianna Yuan, Fei Wu, Jiwei Li,
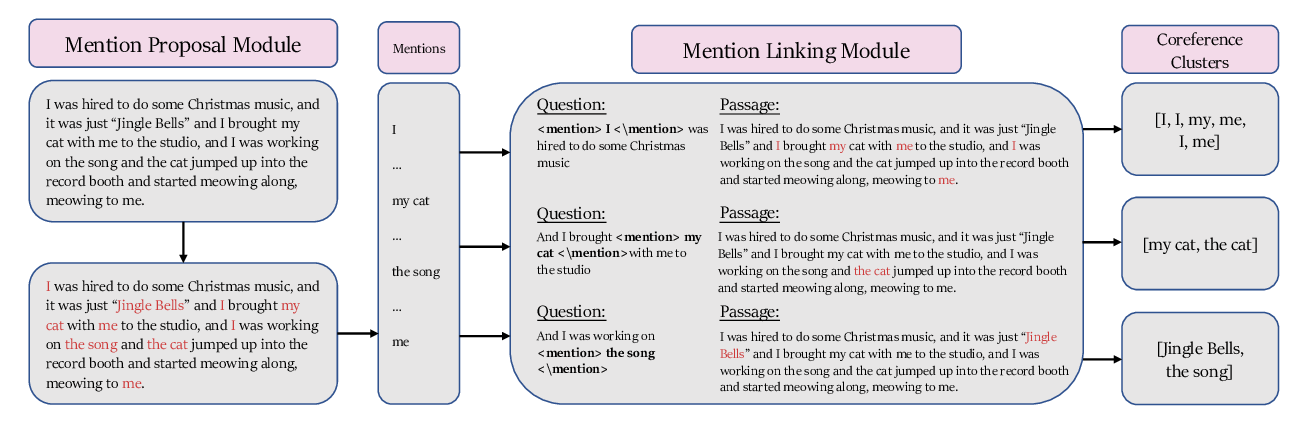