Improving Truthfulness of Headline Generation
Kazuki Matsumaru, Sho Takase, Naoaki Okazaki
Summarization Long Paper
Session 2A: Jul 6
(08:00-09:00 GMT)
Session 3B: Jul 6
(13:00-14:00 GMT)
Abstract:
Most studies on abstractive summarization report ROUGE scores between system and reference summaries. However, we have a concern about the truthfulness of generated summaries: whether all facts of a generated summary are mentioned in the source text. This paper explores improving the truthfulness in headline generation on two popular datasets. Analyzing headlines generated by the state-of-the-art encoder-decoder model, we show that the model sometimes generates untruthful headlines. We conjecture that one of the reasons lies in untruthful supervision data used for training the model. In order to quantify the truthfulness of article-headline pairs, we consider the textual entailment of whether an article entails its headline. After confirming quite a few untruthful instances in the datasets, this study hypothesizes that removing untruthful instances from the supervision data may remedy the problem of the untruthful behaviors of the model. Building a binary classifier that predicts an entailment relation between an article and its headline, we filter out untruthful instances from the supervision data. Experimental results demonstrate that the headline generation model trained on filtered supervision data shows no clear difference in ROUGE scores but remarkable improvements in automatic and manual evaluations of the generated headlines.
You can open the
pre-recorded video
in a separate window.
NOTE: The SlidesLive video may display a random order of the authors.
The correct author list is shown at the top of this webpage.
Similar Papers
Hooks in the Headline: Learning to Generate Headlines with Controlled Styles
Di Jin, Zhijing Jin, Joey Tianyi Zhou, Lisa Orii, Peter Szolovits,
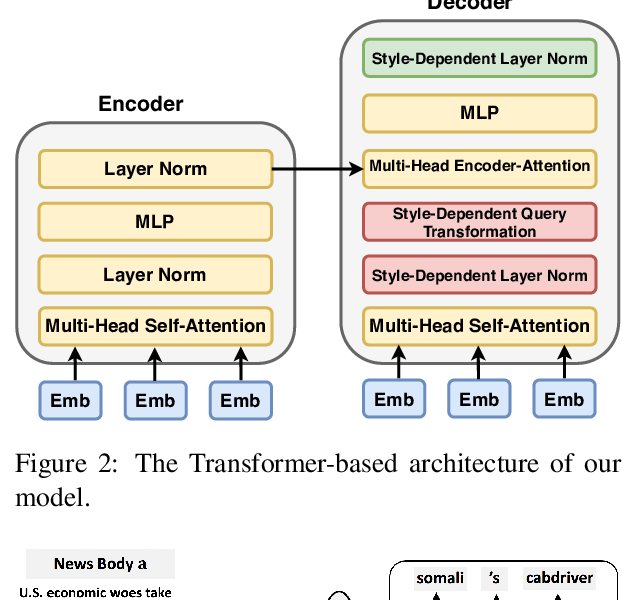
FEQA: A Question Answering Evaluation Framework for Faithfulness Assessment in Abstractive Summarization
Esin Durmus, He He, Mona Diab,
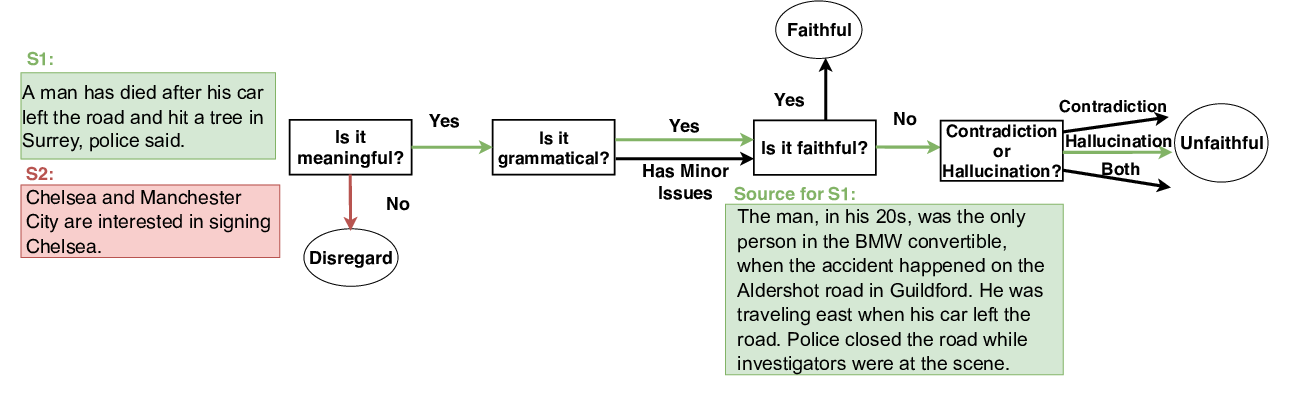
Optimizing the Factual Correctness of a Summary: A Study of Summarizing Radiology Reports
Yuhao Zhang, Derek Merck, Emily Tsai, Christopher D. Manning, Curtis Langlotz,
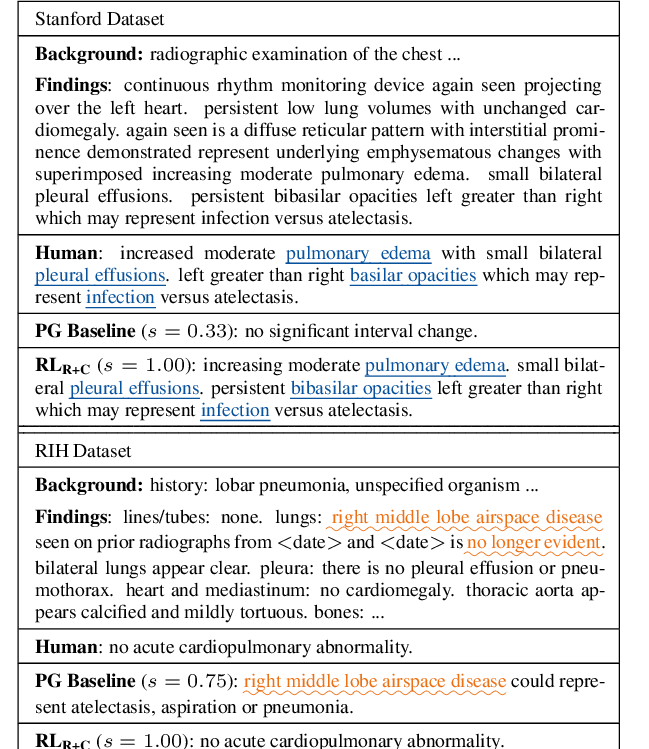
On Faithfulness and Factuality in Abstractive Summarization
Joshua Maynez, Shashi Narayan, Bernd Bohnet, Ryan McDonald,
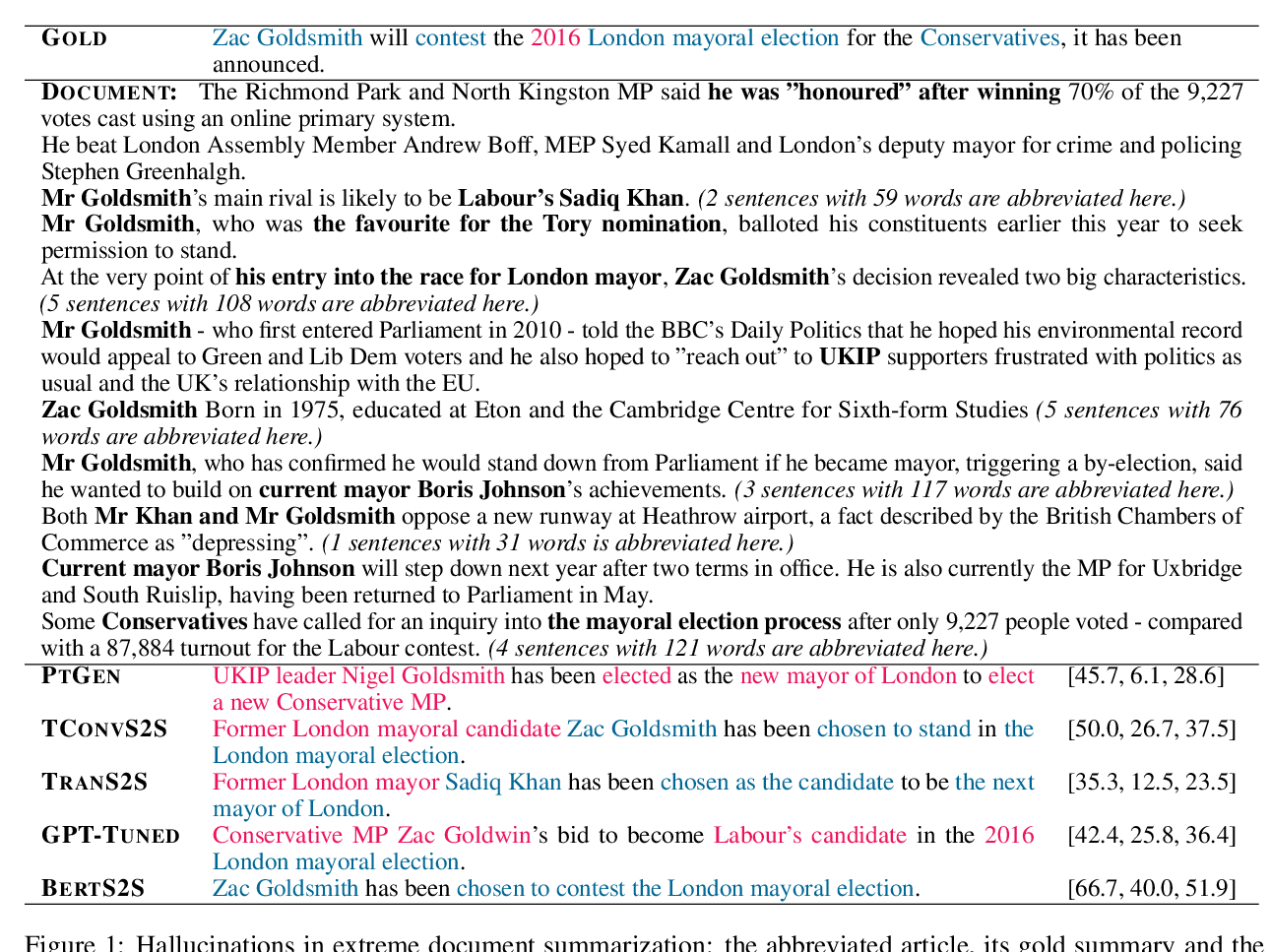