Self-Attention Guided Copy Mechanism for Abstractive Summarization
Song Xu, Haoran Li, Peng Yuan, Youzheng Wu, Xiaodong He, Bowen Zhou
Summarization Short Paper
Session 2A: Jul 6
(08:00-09:00 GMT)
Session 3B: Jul 6
(13:00-14:00 GMT)
Abstract:
Copy module has been widely equipped in the recent abstractive summarization models, which facilitates the decoder to extract words from the source into the summary. Generally, the encoder-decoder attention is served as the copy distribution, while how to guarantee that important words in the source are copied remains a challenge. In this work, we propose a Transformer-based model to enhance the copy mechanism. Specifically, we identify the importance of each source word based on the degree centrality with a directed graph built by the self-attention layer in the Transformer. We use the centrality of each source word to guide the copy process explicitly. Experimental results show that the self-attention graph provides useful guidance for the copy distribution. Our proposed models significantly outperform the baseline methods on the CNN/Daily Mail dataset and the Gigaword dataset.
You can open the
pre-recorded video
in a separate window.
NOTE: The SlidesLive video may display a random order of the authors.
The correct author list is shown at the top of this webpage.
Similar Papers
Attend, Translate and Summarize: An Efficient Method for Neural Cross-Lingual Summarization
Junnan Zhu, Yu Zhou, Jiajun Zhang, Chengqing Zong,
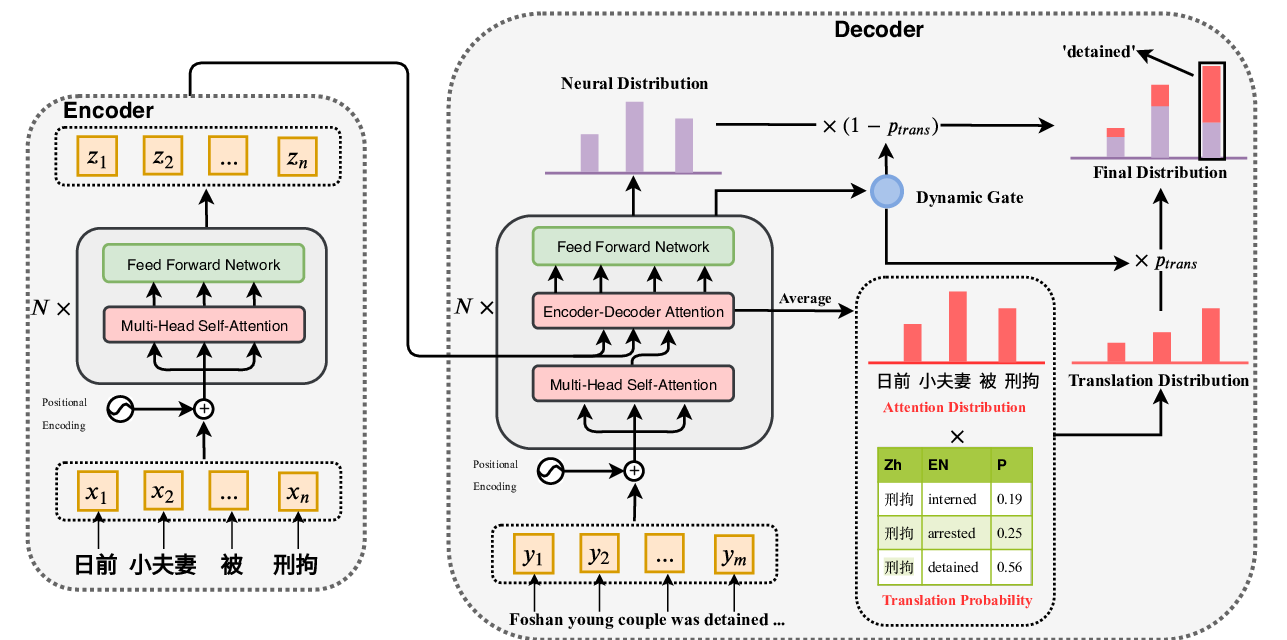
DeFormer: Decomposing Pre-trained Transformers for Faster Question Answering
Qingqing Cao, Harsh Trivedi, Aruna Balasubramanian, Niranjan Balasubramanian,
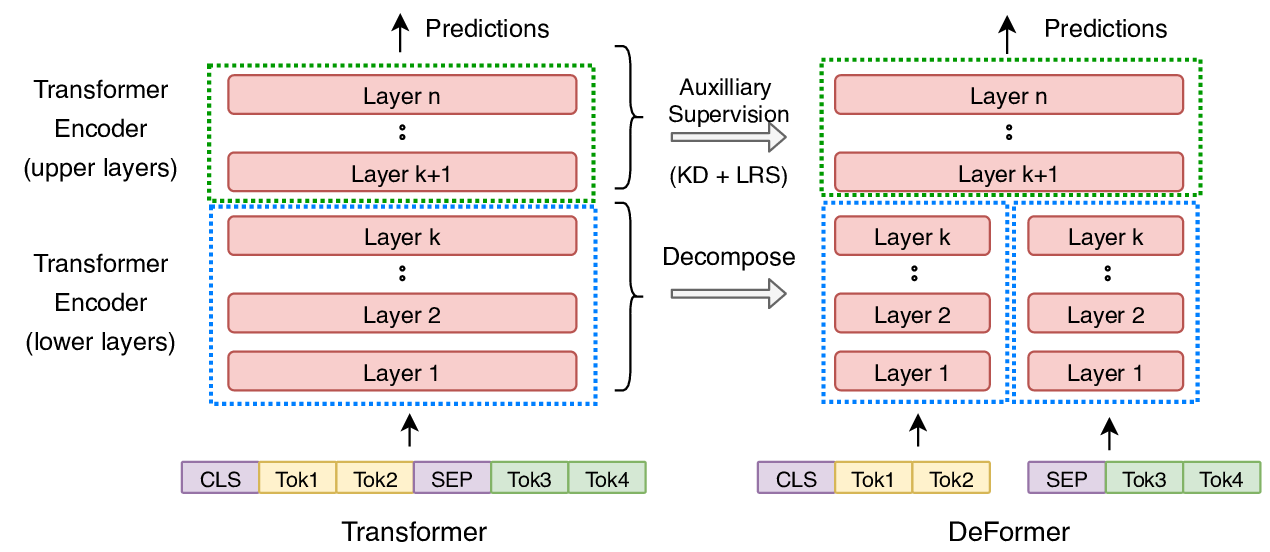
IMoJIE: Iterative Memory-Based Joint Open Information Extraction
Keshav Kolluru, Samarth Aggarwal, Vipul Rathore, Mausam -, Soumen Chakrabarti,
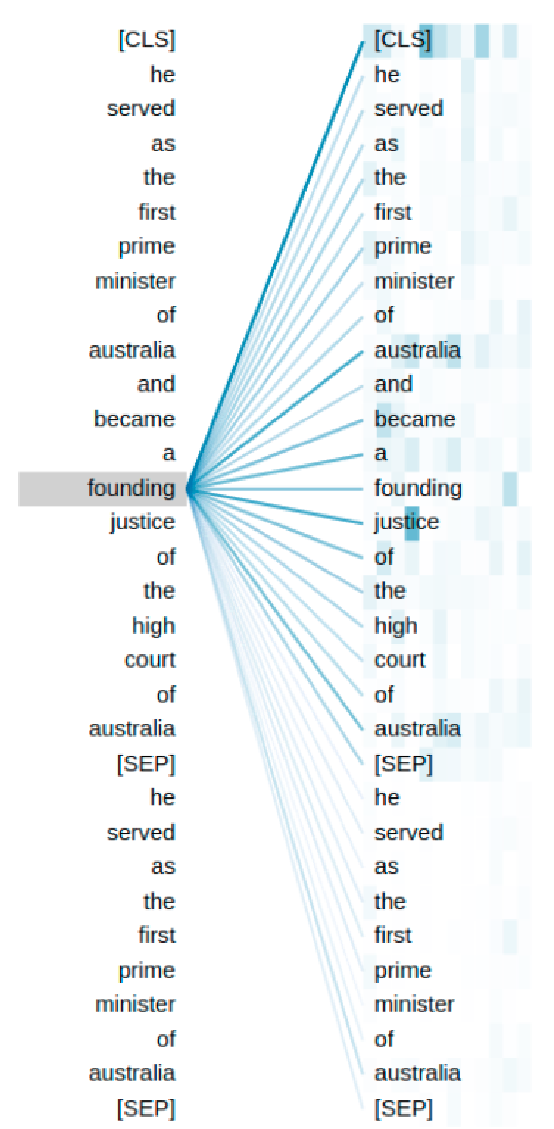