Probing Linguistic Features of Sentence-Level Representations in Relation Extraction
Christoph Alt, Aleksandra Gabryszak, Leonhard Hennig
Information Extraction Long Paper
Session 2B: Jul 6
(09:00-10:00 GMT)
Session 3B: Jul 6
(13:00-14:00 GMT)
Abstract:
Despite the recent progress, little is known about the features captured by state-of-the-art neural relation extraction (RE) models. Common methods encode the source sentence, conditioned on the entity mentions, before classifying the relation. However, the complexity of the task makes it difficult to understand how encoder architecture and supporting linguistic knowledge affect the features learned by the encoder. We introduce 14 probing tasks targeting linguistic properties relevant to RE, and we use them to study representations learned by more than 40 different encoder architecture and linguistic feature combinations trained on two datasets, TACRED and SemEval 2010 Task 8. We find that the bias induced by the architecture and the inclusion of linguistic features are clearly expressed in the probing task performance. For example, adding contextualized word representations greatly increases performance on probing tasks with a focus on named entity and part-of-speech information, and yields better results in RE. In contrast, entity masking improves RE, but considerably lowers performance on entity type related probing tasks.
You can open the
pre-recorded video
in a separate window.
NOTE: The SlidesLive video may display a random order of the authors.
The correct author list is shown at the top of this webpage.
Similar Papers
Perturbed Masking: Parameter-free Probing for Analyzing and Interpreting BERT
Zhiyong Wu, Yun Chen, Ben Kao, Qun Liu,
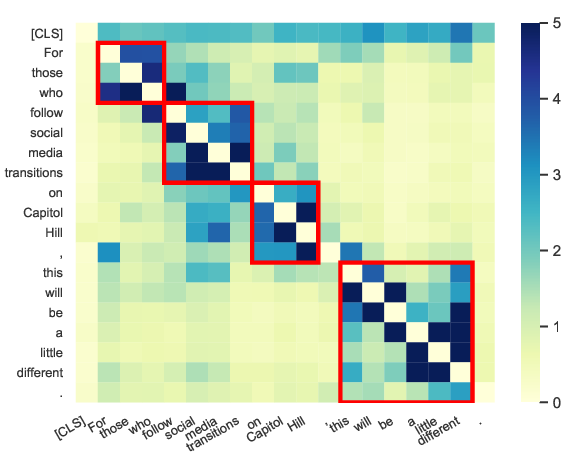
LINSPECTOR: Multilingual Probing Tasks for Word Representations
Gözde Gül Sahin, Clara Vania, Ilia Kuznetsov, Iryna Gurevych,
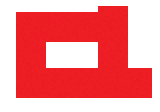
On the Linguistic Representational Power of Neural Machine Translation Models
Yonatan Belinkov, Nadir Durrani, Fahim Dalvi, Hassan Sajjad, James Glass,
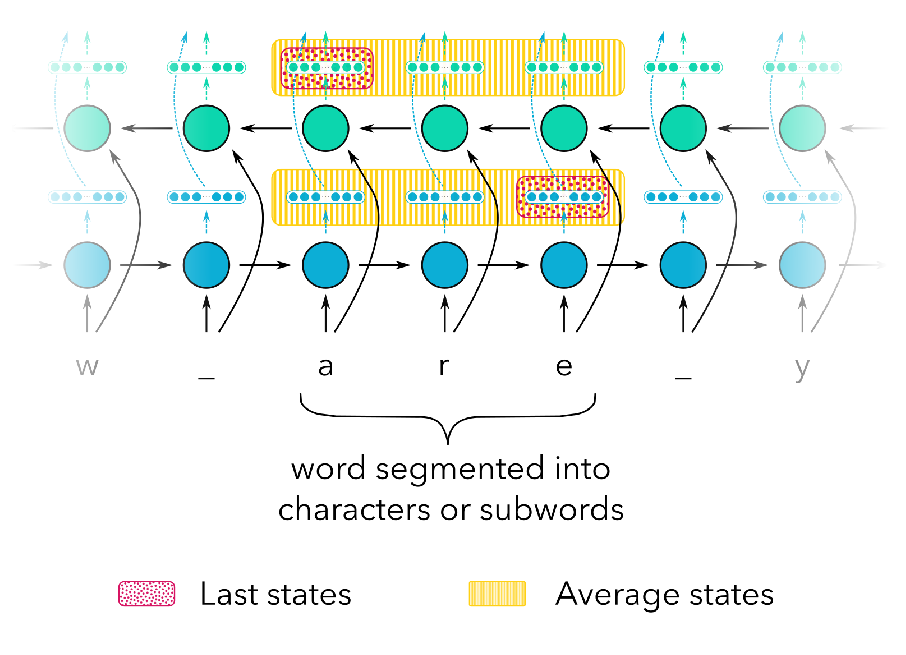
A Systematic Assessment of Syntactic Generalization in Neural Language Models
Jennifer Hu, Jon Gauthier, Peng Qian, Ethan Wilcox, Roger Levy,
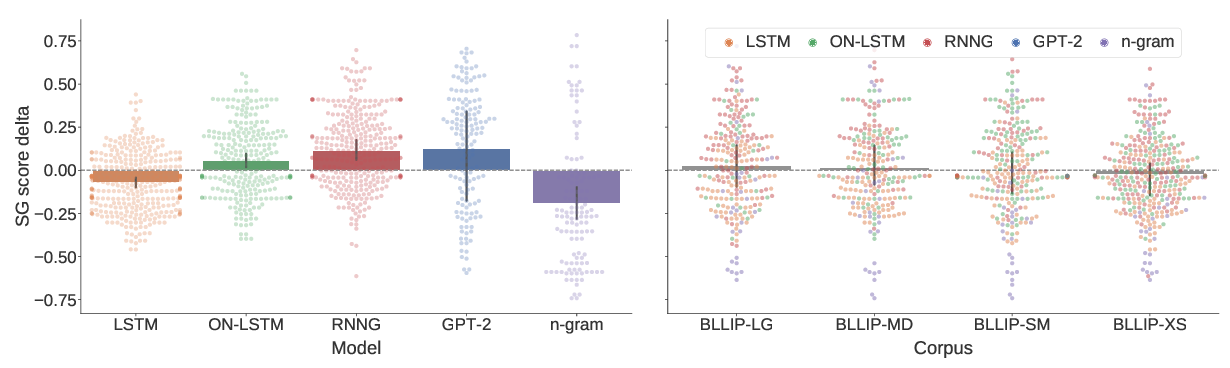