LINSPECTOR: Multilingual Probing Tasks for Word Representations
Gözde Gül Sahin, Clara Vania, Ilia Kuznetsov, Iryna Gurevych
Resources and Evaluation CL Paper
Session 9A: Jul 7
(17:00-18:00 GMT)
Session 10A: Jul 7
(20:00-21:00 GMT)
Abstract:
Despite an ever growing number of word representation models introduced for a large number of languages, there is a lack of a standardized technique to provide insights into what is captured by these models. Such insights would help the community to get an estimate of the downstream task performance, as well as to design more informed neural architectures, while avoiding extensive experimentation which requires substantial computational resources not all researchers have access to. A recent development in NLP is to use simple classification tasks, also called probing tasks, that test for a single linguistic feature such as part-of-speech. Existing studies mostly focus on exploring the linguistic information encoded by the continuous representations of English text. However, from a typological perspective the morphologically poor English is rather an outlier: the information encoded by the word order and function words in English is often stored on a subword, morphological level in other languages. To address this, we introduce 15 type-level probing tasks such as case marking, possession, word length, morphological tag count and pseudoword identification for 24 languages. We present a reusable methodology for creation and evaluation of such tests in a multilingual setting, which is challenging due to lack of resources, lower quality of tools and differences among languages. We then present experiments on several diverse multilingual word embedding models, in which we relate the probing task performance for a diverse set of languages to a range of five classic NLP tasks: POS-tagging, dependency parsing, semantic role labeling, named entity recognition and natural language inference. We find that a number of probing tests have significantly high positive correlation to the downstream tasks, especially for morphologically rich languages. We show that our tests can be used to explore word embeddings or black-box neural models for linguistic cues in a multilingual setting. We release the probing datasets and the evaluation suite LINSPECTOR with https://github.com/UKPLab/linspector.
You can open the
pre-recorded video
in a separate window.
NOTE: The SlidesLive video may display a random order of the authors.
The correct author list is shown at the top of this webpage.
Similar Papers
Information-Theoretic Probing for Linguistic Structure
Tiago Pimentel, Josef Valvoda, Rowan Hall Maudslay, Ran Zmigrod, Adina Williams, Ryan Cotterell,
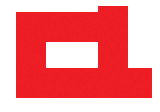
Stanza: A Python Natural Language Processing Toolkit for Many Human Languages
Peng Qi, Yuhao Zhang, Yuhui Zhang, Jason Bolton, Christopher D. Manning,
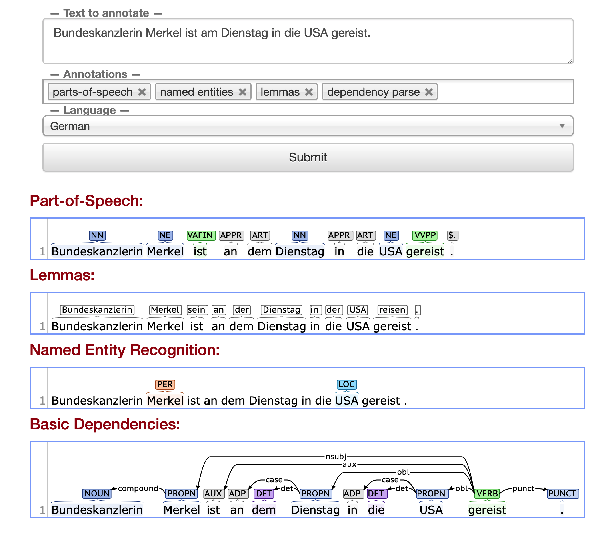
Probing Linguistic Features of Sentence-Level Representations in Relation Extraction
Christoph Alt, Aleksandra Gabryszak, Leonhard Hennig,
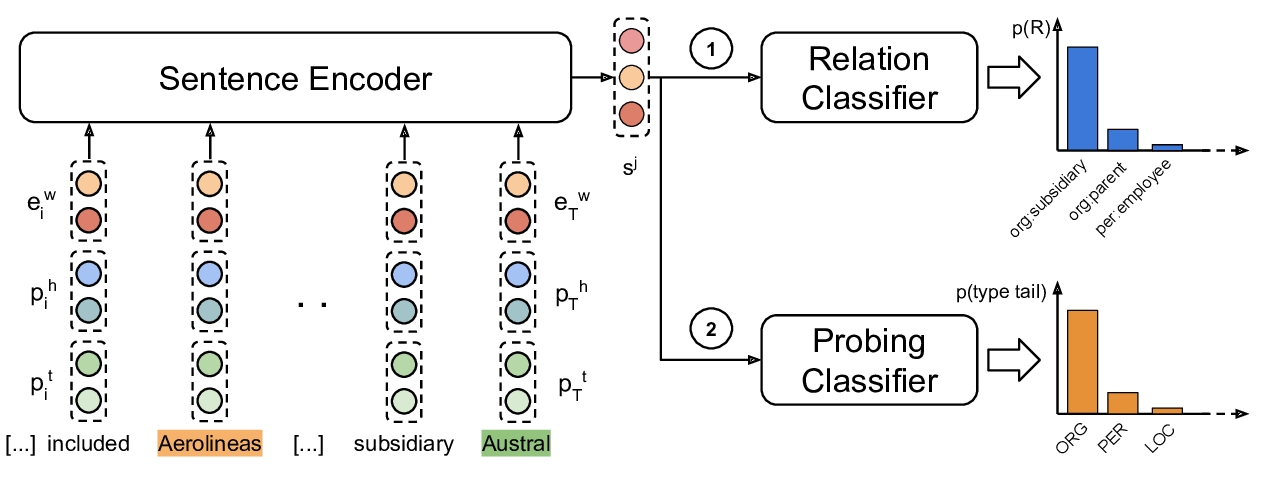
Perturbed Masking: Parameter-free Probing for Analyzing and Interpreting BERT
Zhiyong Wu, Yun Chen, Ben Kao, Qun Liu,
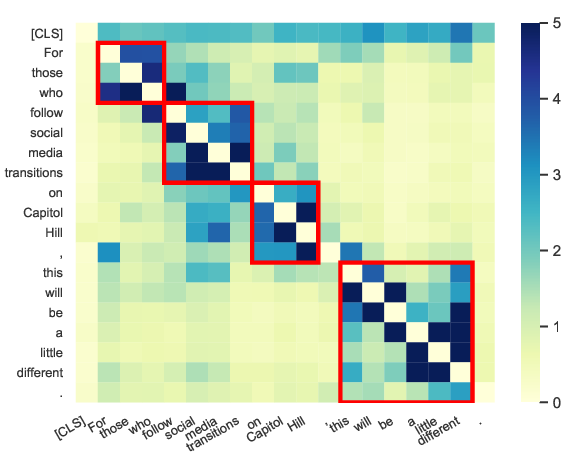