Character-Level Translation with Self-attention
Yingqiang Gao, Nikola I. Nikolov, Yuhuang Hu, Richard H.R. Hahnloser
Machine Translation Short Paper
Session 2B: Jul 6
(09:00-10:00 GMT)
Session 3B: Jul 6
(13:00-14:00 GMT)
Abstract:
We explore the suitability of self-attention models for character-level neural machine translation. We test the standard transformer model, as well as a novel variant in which the encoder block combines information from nearby characters using convolutions. We perform extensive experiments on WMT and UN datasets, testing both bilingual and multilingual translation to English using up to three input languages (French, Spanish, and Chinese). Our transformer variant consistently outperforms the standard transformer at the character-level and converges faster while learning more robust character-level alignments.
You can open the
pre-recorded video
in a separate window.
NOTE: The SlidesLive video may display a random order of the authors.
The correct author list is shown at the top of this webpage.
Similar Papers
Combining Subword Representations into Word-level Representations in the Transformer Architecture
Noe Casas, Marta R. Costa-jussà, José A. R. Fonollosa,
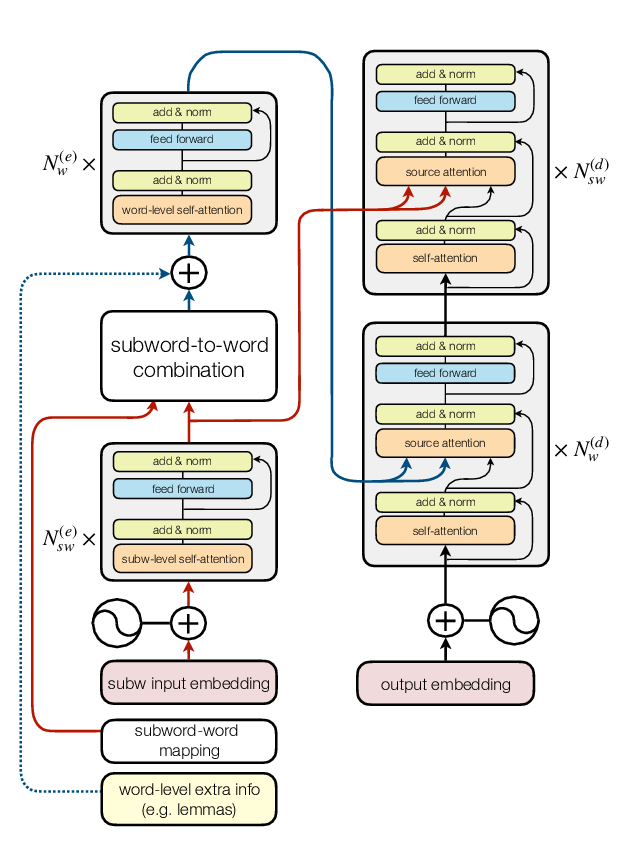
Lipschitz Constrained Parameter Initialization for Deep Transformers
Hongfei Xu, Qiuhui Liu, Josef van Genabith, Deyi Xiong, Jingyi Zhang,
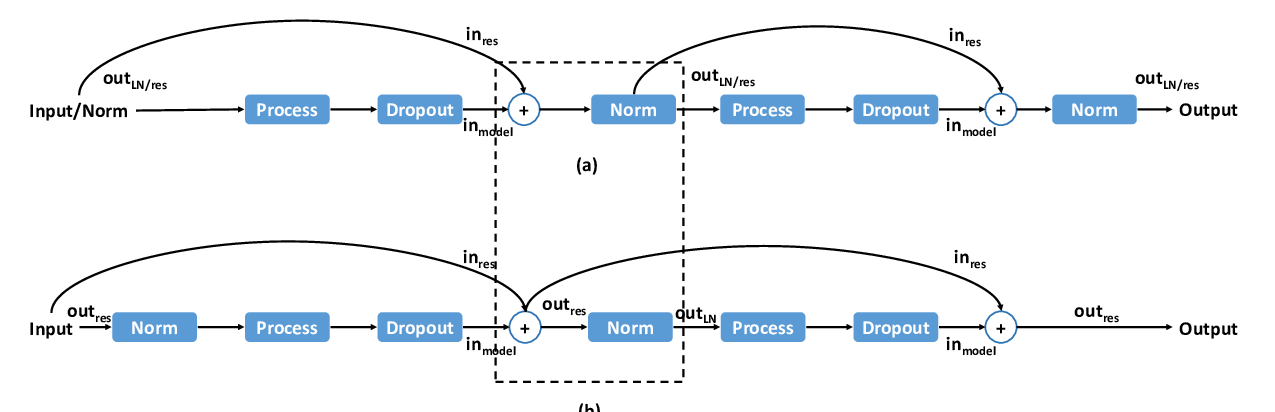