Enhancing Machine Translation with Dependency-Aware Self-Attention
Emanuele Bugliarello, Naoaki Okazaki
Machine Translation Short Paper
Session 2B: Jul 6
(09:00-10:00 GMT)
Session 3A: Jul 6
(12:00-13:00 GMT)
Abstract:
Most neural machine translation models only rely on pairs of parallel sentences, assuming syntactic information is automatically learned by an attention mechanism. In this work, we investigate different approaches to incorporate syntactic knowledge in the Transformer model and also propose a novel, parameter-free, dependency-aware self-attention mechanism that improves its translation quality, especially for long sentences and in low-resource scenarios. We show the efficacy of each approach on WMT English-German and English-Turkish, and WAT English-Japanese translation tasks.
You can open the
pre-recorded video
in a separate window.
NOTE: The SlidesLive video may display a random order of the authors.
The correct author list is shown at the top of this webpage.
Similar Papers
Leveraging Monolingual Data with Self-Supervision for Multilingual Neural Machine Translation
Aditya Siddhant, Ankur Bapna, Yuan Cao, Orhan Firat, Mia Chen, Sneha Kudugunta, Naveen Arivazhagan, Yonghui Wu,
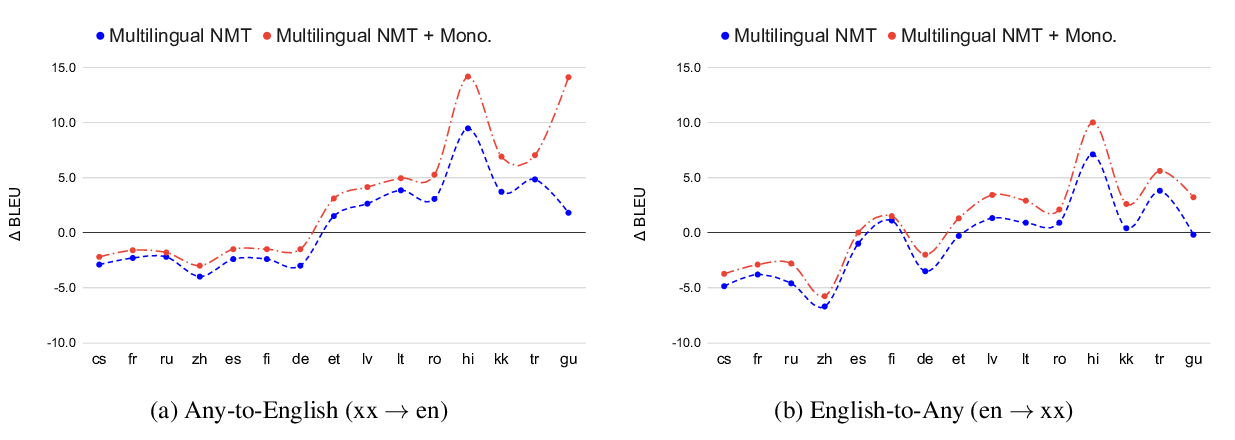
Learning Source Phrase Representations for Neural Machine Translation
Hongfei Xu, Josef van Genabith, Deyi Xiong, Qiuhui Liu, Jingyi Zhang,
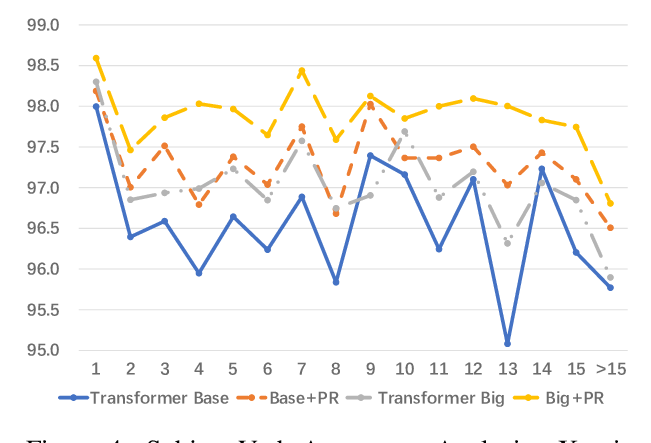
Hypernymy Detection for Low-Resource Languages via Meta Learning
Changlong Yu, Jialong Han, Haisong Zhang, Wilfred Ng,
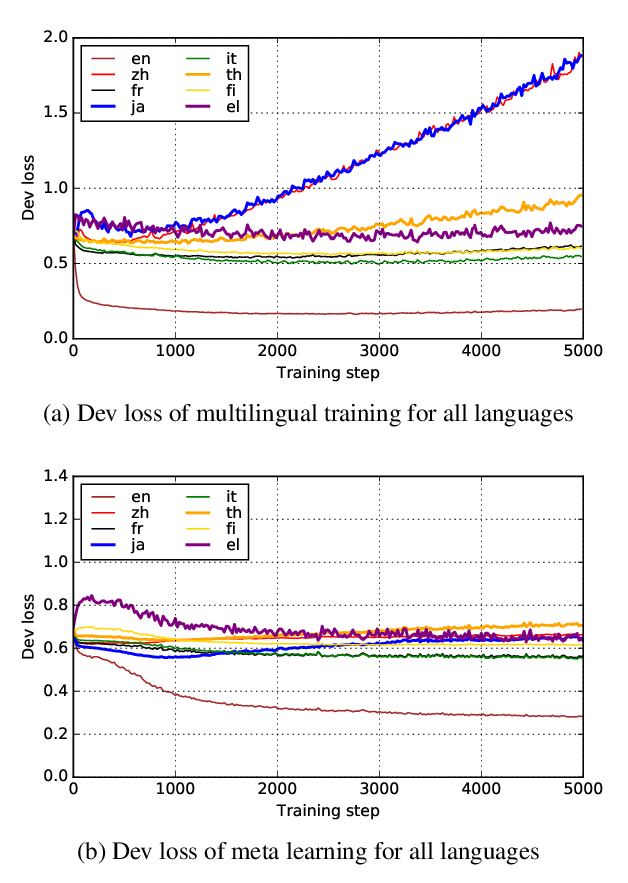