Asking and Answering Questions to Evaluate the Factual Consistency of Summaries
Alex Wang, Kyunghyun Cho, Mike Lewis
Summarization Long Paper
Session 9A: Jul 7
(17:00-18:00 GMT)
Session 10B: Jul 7
(21:00-22:00 GMT)
Abstract:
Practical applications of abstractive summarization models are limited by frequent factual inconsistencies with respect to their input. Existing automatic evaluation metrics for summarization are largely insensitive to such errors. We propose QAGS (pronounced ``kags''), an automatic evaluation protocol that is designed to identify factual inconsistencies in a generated summary. QAGS is based on the intuition that if we ask questions about a summary and its source, we will receive similar answers if the summary is factually consistent with the source. To evaluate QAGS, we collect human judgments of factual consistency on model-generated summaries for the CNN/DailyMail (Hermann et al., 2015) and XSUM (Narayan et al., 2018) summarization datasets. QAGS has substantially higher correlations with these judgments than other automatic evaluation metrics. Also, QAGS offers a natural form of interpretability: The answers and questions generated while computing QAGS indicate which tokens of a summary are inconsistent and why. We believe QAGS is a promising tool in automatically generating usable and factually consistent text. Code for QAGS will be available at https://github.com/W4ngatang/qags.
You can open the
pre-recorded video
in a separate window.
NOTE: The SlidesLive video may display a random order of the authors.
The correct author list is shown at the top of this webpage.
Similar Papers
FEQA: A Question Answering Evaluation Framework for Faithfulness Assessment in Abstractive Summarization
Esin Durmus, He He, Mona Diab,
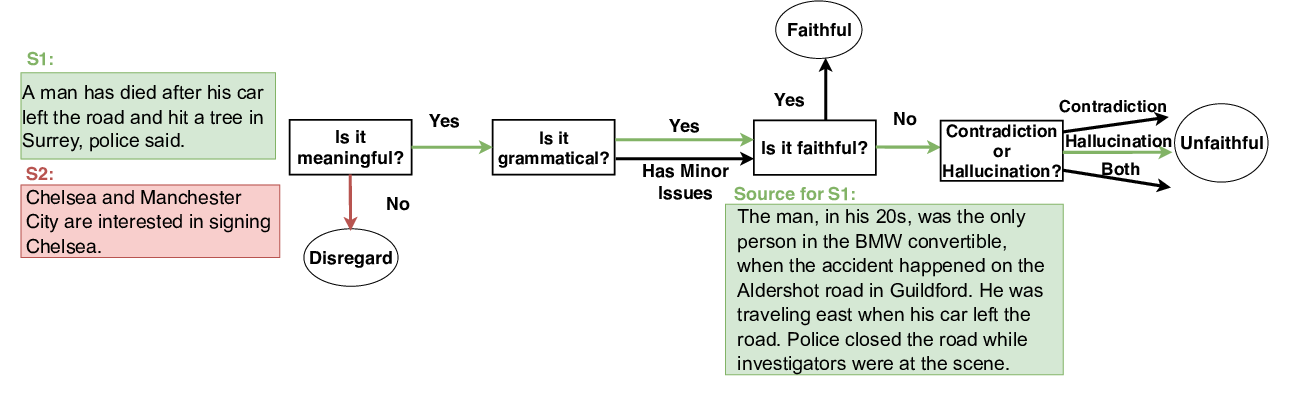
Template-Based Question Generation from Retrieved Sentences for Improved Unsupervised Question Answering
Alexander Fabbri, Patrick Ng, Zhiguo Wang, Ramesh Nallapati, Bing Xiang,
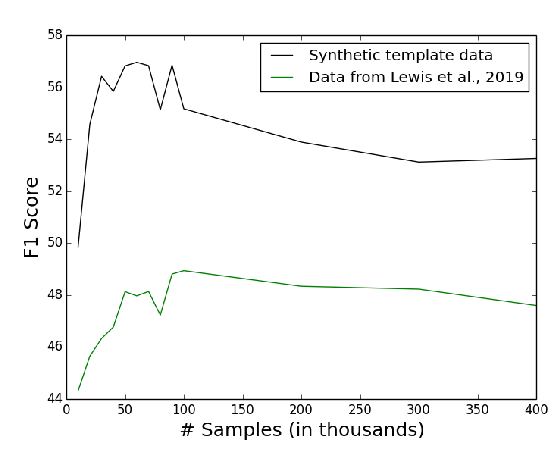
Fluent Response Generation for Conversational Question Answering
Ashutosh Baheti, Alan Ritter, Kevin Small,
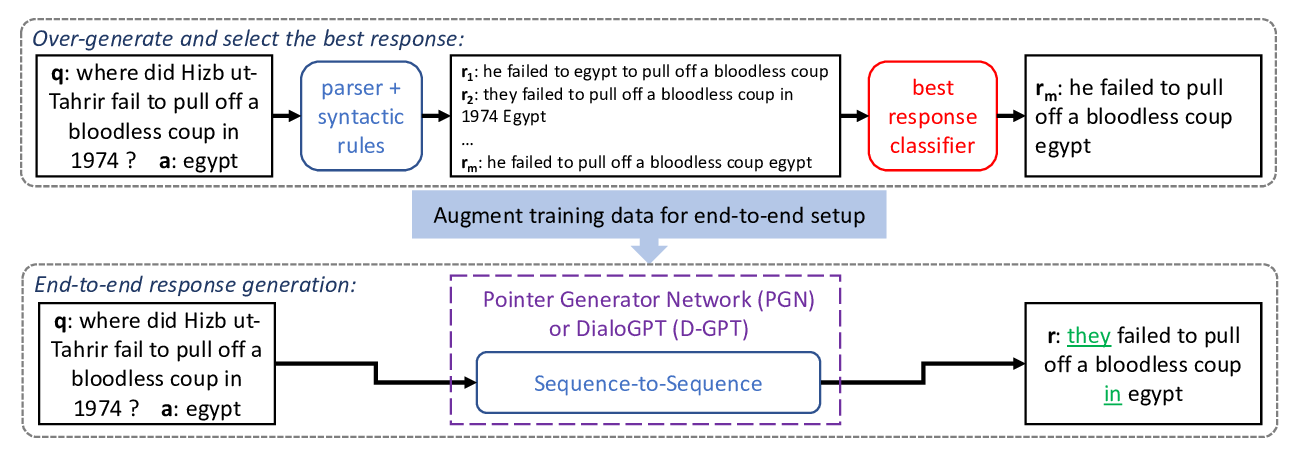
Generating Diverse and Consistent QA pairs from Contexts with Information-Maximizing Hierarchical Conditional VAEs
Dong Bok Lee, Seanie Lee, Woo Tae Jeong, Donghwan Kim, Sung Ju Hwang,
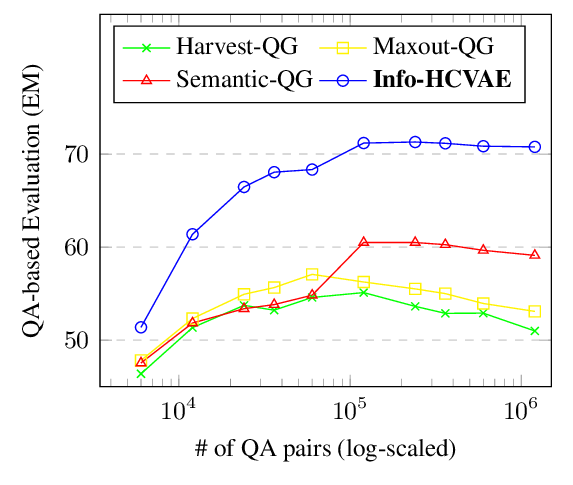