Generating Diverse and Consistent QA pairs from Contexts with Information-Maximizing Hierarchical Conditional VAEs
Dong Bok Lee, Seanie Lee, Woo Tae Jeong, Donghwan Kim, Sung Ju Hwang
Generation Long Paper
Session 1A: Jul 6
(05:00-06:00 GMT / 05:00-06:00 GMT)
Session 3A: Jul 6
(12:00-13:00 GMT / 12:00-13:00 GMT)
Abstract:
One of the most crucial challenges in question answering (QA) is the scarcity of labeled data, since it is costly to obtain question-answer (QA) pairs for a target text domain with human annotation. An alternative approach to tackle the problem is to use automatically generated QA pairs from either the problem context or from large amount of unstructured texts (e.g. Wikipedia). In this work, we propose a hierarchical conditional variational autoencoder (HCVAE) for generating QA pairs given unstructured texts as contexts, while maximizing the mutual information between generated QA pairs to ensure their consistency. We validate our Information Maximizing Hierarchical Conditional Variational AutoEncoder (Info-HCVAE) on several benchmark datasets by evaluating the performance of the QA model (BERT-base) using only the generated QA pairs (QA-based evaluation) or by using both the generated and human-labeled pairs (semi-supervised learning) for training, against state-of-the-art baseline models. The results show that our model obtains impressive performance gains over all baselines on both tasks, using only a fraction of data for training.
You can open the
pre-recorded video
in a separate window.
NOTE: The SlidesLive video may display a random order of the authors.
The correct author list is shown at the top of this webpage.
Similar Papers
Template-Based Question Generation from Retrieved Sentences for Improved Unsupervised Question Answering
Alexander Fabbri, Patrick Ng, Zhiguo Wang, Ramesh Nallapati, Bing Xiang,
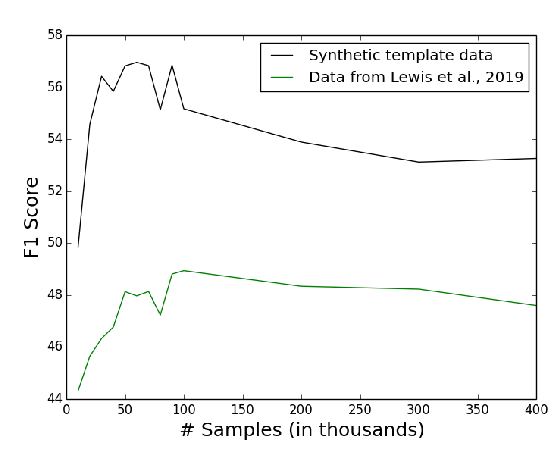
On the Importance of Diversity in Question Generation for QA
Md Arafat Sultan, Shubham Chandel, Ramón Fernandez Astudillo, Vittorio Castelli,
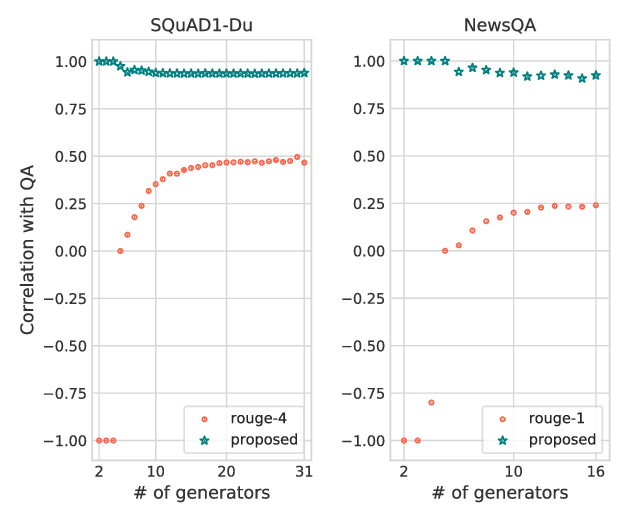
DoQA - Accessing Domain-Specific FAQs via Conversational QA
Jon Ander Campos, Arantxa Otegi, Aitor Soroa, Jan Deriu, Mark Cieliebak, Eneko Agirre,
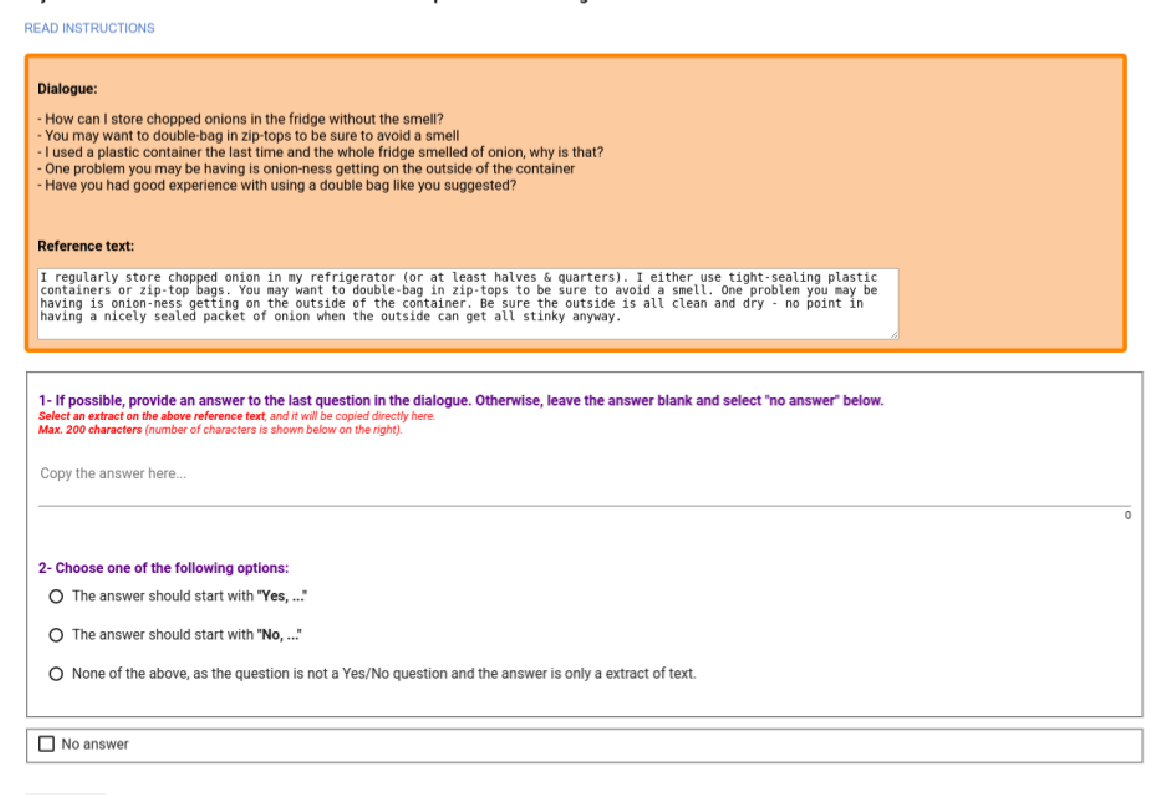