QuASE: Question-Answer Driven Sentence Encoding
Hangfeng He, Qiang Ning, Dan Roth
Semantics: Textual Inference and Other Areas of Semantics Long Paper
Session 14B: Jul 8
(18:00-19:00 GMT)
Session 15A: Jul 8
(20:00-21:00 GMT)
Abstract:
Question-answering (QA) data often encodes essential information in many facets. This paper studies a natural question: Can we get supervision from QA data for other tasks (typically, non-QA ones)? For example, can we use QAMR (Michael et al., 2017) to improve named entity recognition? We suggest that simply further pre-training BERT is often not the best option, and propose the question-answer driven sentence encoding (QuASE) framework. QuASE learns representations from QA data, using BERT or other state-of-the-art contextual language models. In particular, we observe the need to distinguish between two types of sentence encodings, depending on whether the target task is a single- or multi-sentence input; in both cases, the resulting encoding is shown to be an easy-to-use plugin for many downstream tasks. This work may point out an alternative way to supervise NLP tasks.
You can open the
pre-recorded video
in a separate window.
NOTE: The SlidesLive video may display a random order of the authors.
The correct author list is shown at the top of this webpage.
Similar Papers
Template-Based Question Generation from Retrieved Sentences for Improved Unsupervised Question Answering
Alexander Fabbri, Patrick Ng, Zhiguo Wang, Ramesh Nallapati, Bing Xiang,
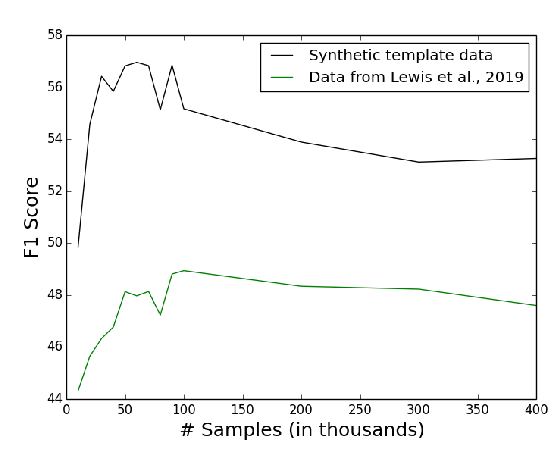
Generating Diverse and Consistent QA pairs from Contexts with Information-Maximizing Hierarchical Conditional VAEs
Dong Bok Lee, Seanie Lee, Woo Tae Jeong, Donghwan Kim, Sung Ju Hwang,
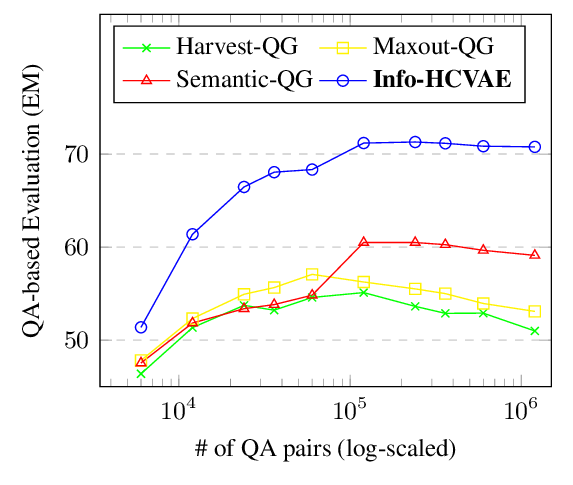
Fluent Response Generation for Conversational Question Answering
Ashutosh Baheti, Alan Ritter, Kevin Small,
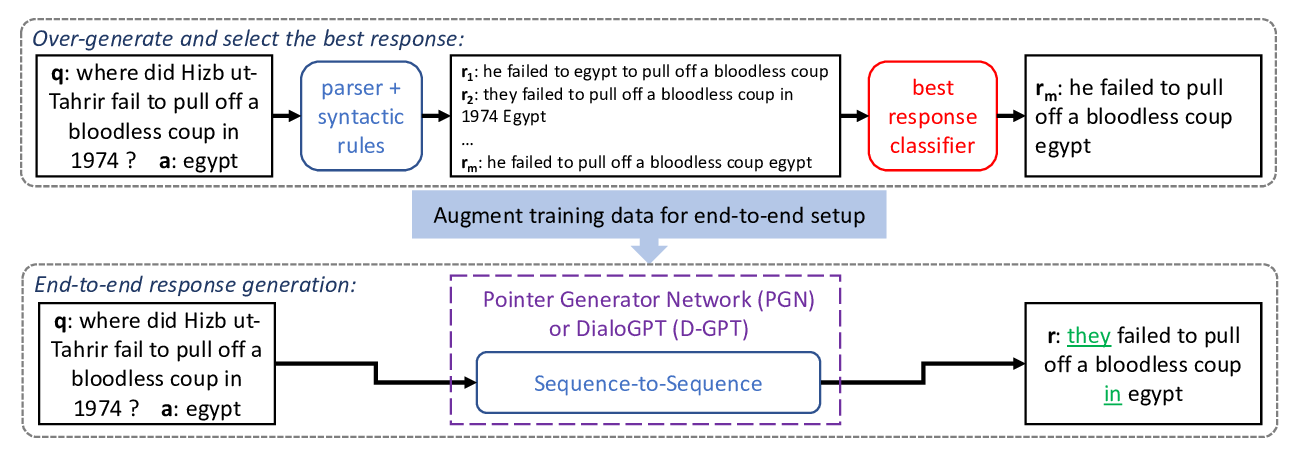
Harvesting and Refining Question-Answer Pairs for Unsupervised QA
Zhongli Li, Wenhui Wang, Li Dong, Furu Wei, Ke Xu,
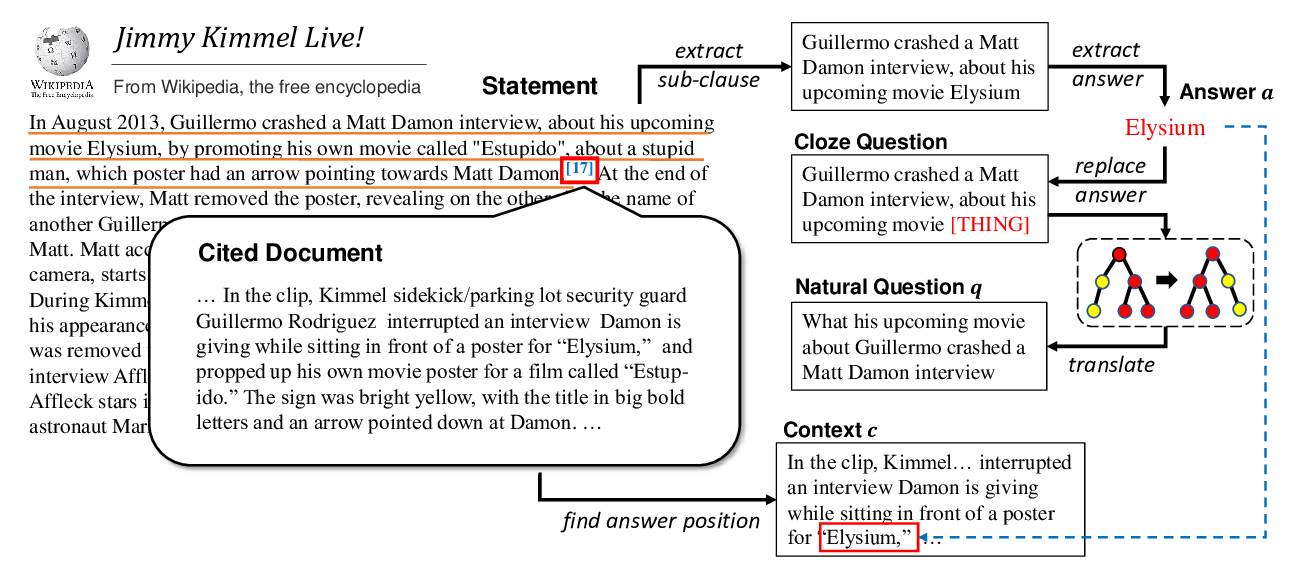