On the Importance of Diversity in Question Generation for QA
Md Arafat Sultan, Shubham Chandel, Ramón Fernandez Astudillo, Vittorio Castelli
Question Answering Short Paper
Session 9B: Jul 7
(18:00-19:00 GMT)
Session 10B: Jul 7
(21:00-22:00 GMT)
Abstract:
Automatic question generation (QG) has shown promise as a source of synthetic training data for question answering (QA). In this paper we ask: Is textual diversity in QG beneficial for downstream QA? Using top-p nucleus sampling to derive samples from a transformer-based question generator, we show that diversity-promoting QG indeed provides better QA training than likelihood maximization approaches such as beam search. We also show that standard QG evaluation metrics such as BLEU, ROUGE and METEOR are inversely correlated with diversity, and propose a diversity-aware intrinsic measure of overall QG quality that correlates well with extrinsic evaluation on QA.
You can open the
pre-recorded video
in a separate window.
NOTE: The SlidesLive video may display a random order of the authors.
The correct author list is shown at the top of this webpage.
Similar Papers
Generating Diverse and Consistent QA pairs from Contexts with Information-Maximizing Hierarchical Conditional VAEs
Dong Bok Lee, Seanie Lee, Woo Tae Jeong, Donghwan Kim, Sung Ju Hwang,
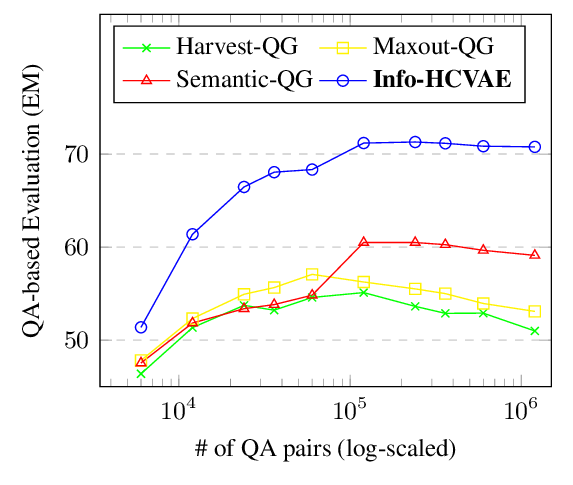
Template-Based Question Generation from Retrieved Sentences for Improved Unsupervised Question Answering
Alexander Fabbri, Patrick Ng, Zhiguo Wang, Ramesh Nallapati, Bing Xiang,
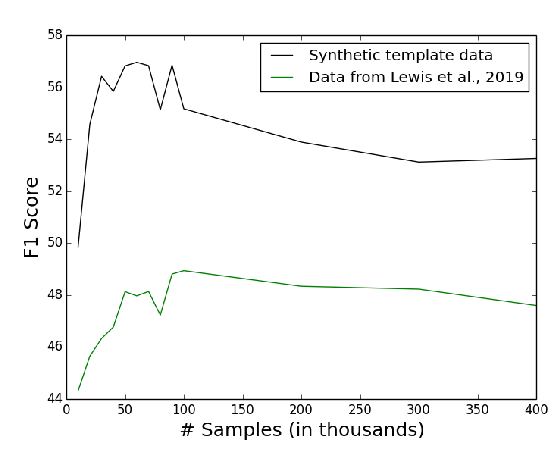
Asking and Answering Questions to Evaluate the Factual Consistency of Summaries
Alex Wang, Kyunghyun Cho, Mike Lewis,
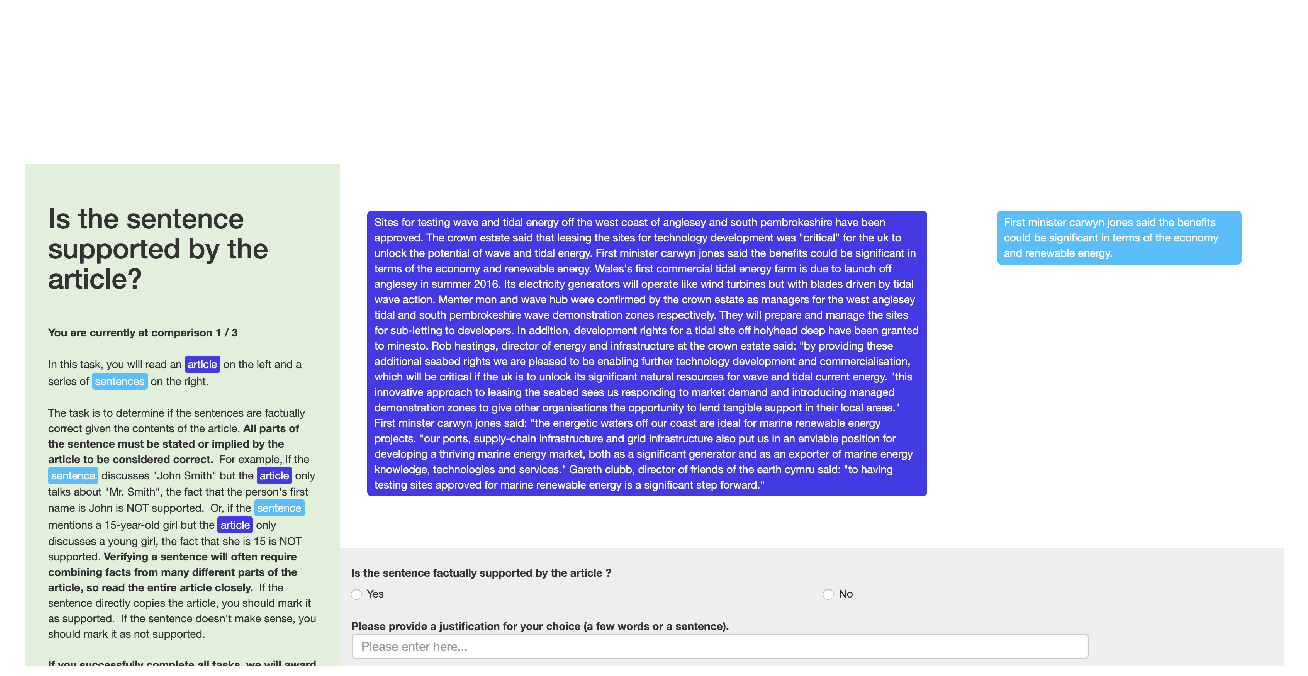