Why Overfitting Isn't Always Bad: Retrofitting Cross-Lingual Word Embeddings to Dictionaries
Mozhi Zhang, Yoshinari Fujinuma, Michael J. Paul, Jordan Boyd-Graber
Machine Learning for NLP Short Paper
Session 4A: Jul 6
(17:00-18:00 GMT)
Session 5A: Jul 6
(20:00-21:00 GMT)
Abstract:
Cross-lingual word embeddings (CLWE) are often evaluated on bilingual lexicon induction (BLI). Recent CLWE methods use linear projections, which underfit the training dictionary, to generalize on BLI. However, underfitting can hinder generalization to other downstream tasks that rely on words from the training dictionary. We address this limitation by retrofitting CLWE to the training dictionary, which pulls training translation pairs closer in the embedding space and overfits the training dictionary. This simple post-processing step often improves accuracy on two downstream tasks, despite lowering BLI test accuracy. We also retrofit to both the training dictionary and a synthetic dictionary induced from CLWE, which sometimes generalizes even better on downstream tasks. Our results confirm the importance of fully exploiting training dictionary in downstream tasks and explains why BLI is a flawed CLWE evaluation.
You can open the
pre-recorded video
in a separate window.
NOTE: The SlidesLive video may display a random order of the authors.
The correct author list is shown at the top of this webpage.
Similar Papers
Bilingual Dictionary Based Neural Machine Translation without Using Parallel Sentences
Xiangyu Duan, Baijun Ji, Hao Jia, Min Tan, Min Zhang, Boxing Chen, Weihua Luo, Yue Zhang,
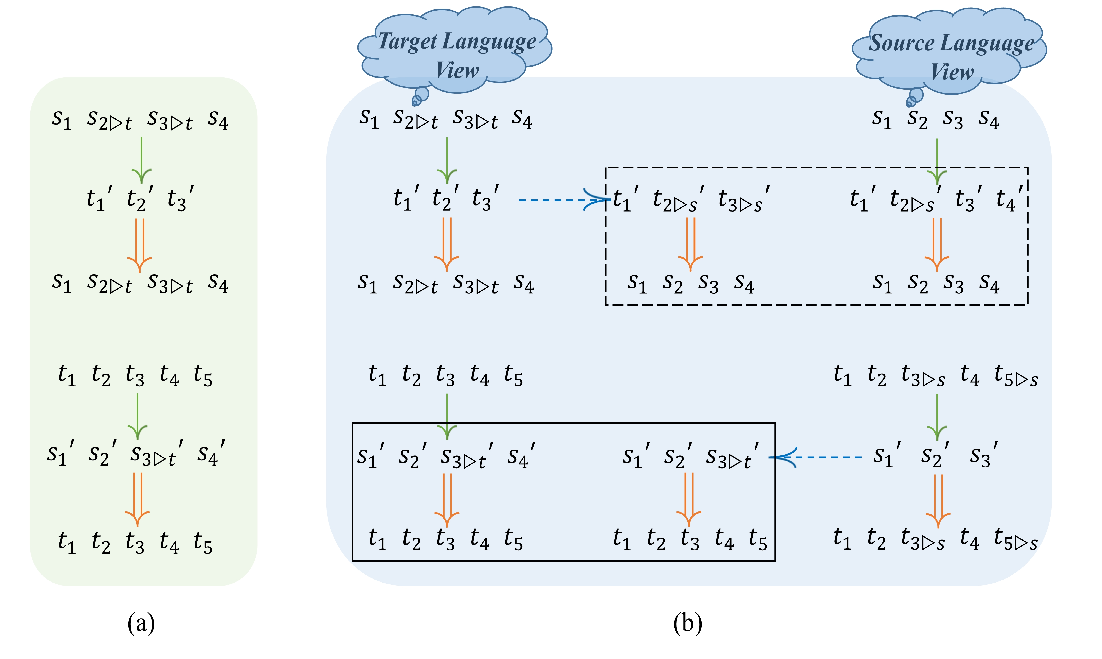
Classification-Based Self-Learning for Weakly Supervised Bilingual Lexicon Induction
Mladen Karan, Ivan Vulić, Anna Korhonen, Goran Glavaš,
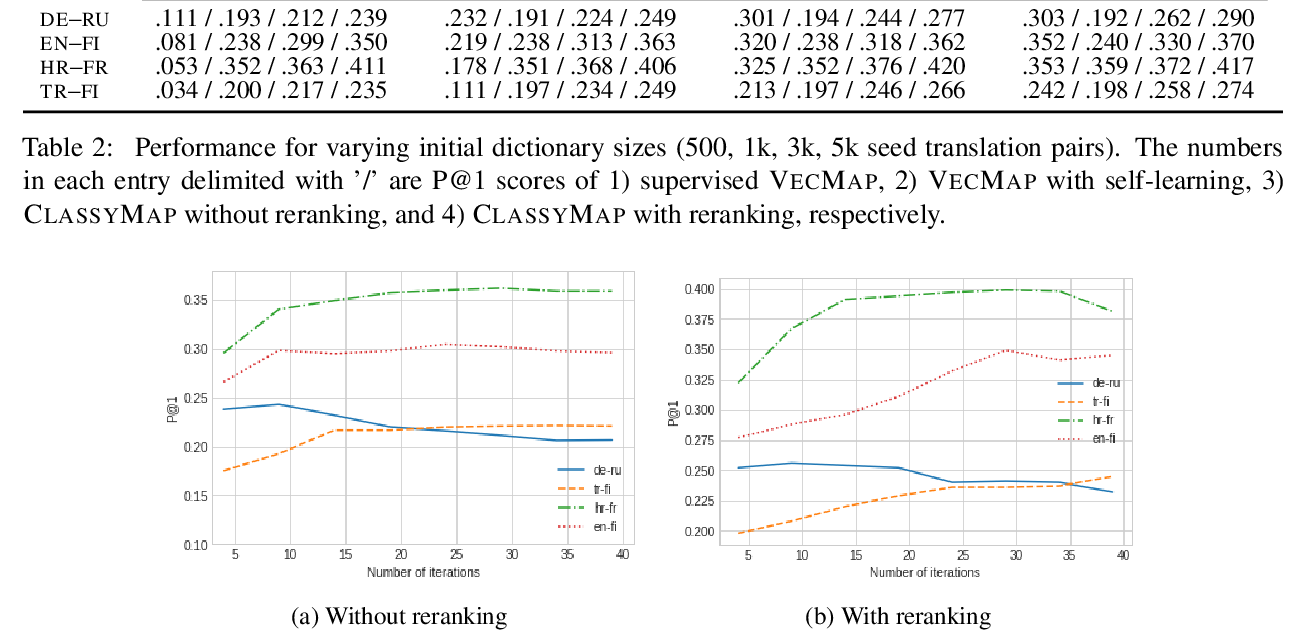
Efficient Contextual Representation Learning With Continuous Outputs
Liunian Harold Li, Patrick H. Chen, Cho-Jui Hsieh, Kai-Wei Chang,
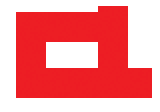