Improving Image Captioning Evaluation by Considering Inter References Variance
Yanzhi Yi, Hangyu Deng, Jinglu Hu
Resources and Evaluation Long Paper
Session 1B: Jul 6
(06:00-07:00 GMT)
Session 2B: Jul 6
(09:00-10:00 GMT)
Abstract:
Evaluating image captions is very challenging partially due to the fact that there are multiple correct captions for every single image. Most of the existing one-to-one metrics operate by penalizing mismatches between reference and generative caption without considering the intrinsic variance between ground truth captions. It usually leads to over-penalization and thus a bad correlation to human judgment. Recently, the latest one-to-one metric BERTScore can achieve high human correlation in system-level tasks while some issues can be fixed for better performance. In this paper, we propose a novel metric based on BERTScore that could handle such a challenge and extend BERTScore with a few new features appropriately for image captioning evaluation. The experimental results show that our metric achieves state-of-the-art human judgment correlation.
You can open the
pre-recorded video
in a separate window.
NOTE: The SlidesLive video may display a random order of the authors.
The correct author list is shown at the top of this webpage.
Similar Papers
Understanding Advertisements with BERT
Kanika Kalra, Bhargav Kurma, Silpa Vadakkeeveetil Sreelatha, Manasi Patwardhan, Shirish Karande,
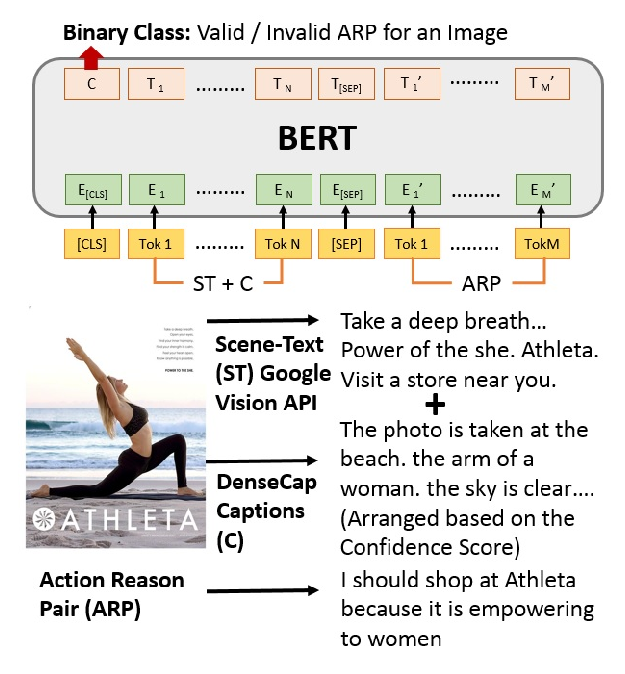
Designing Precise and Robust Dialogue Response Evaluators
Tianyu Zhao, Divesh Lala, Tatsuya Kawahara,
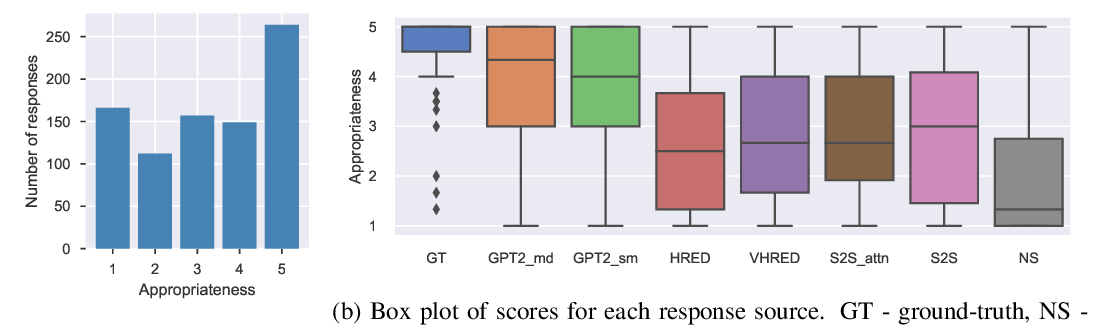