Low Resource Sequence Tagging using Sentence Reconstruction
Tal Perl, Sriram Chaudhury, Raja Giryes
Machine Learning for NLP Short Paper
Session 4B: Jul 6
(18:00-19:00 GMT)
Session 5B: Jul 6
(21:00-22:00 GMT)
Abstract:
This work revisits the task of training sequence tagging models with limited resources using transfer learning. We investigate several proposed approaches introduced in recent works and suggest a new loss that relies on sentence reconstruction from normalized embeddings. Specifically, our method demonstrates how by adding a decoding layer for sentence reconstruction, we can improve the performance of various baselines. We show improved results on the CoNLL02 NER and UD 1.2 POS datasets and demonstrate the power of the method for transfer learning with low-resources achieving 0.6 F1 score in Dutch using only one sample from it.
You can open the
pre-recorded video
in a separate window.
NOTE: The SlidesLive video may display a random order of the authors.
The correct author list is shown at the top of this webpage.
Similar Papers
In Neural Machine Translation, What Does Transfer Learning Transfer?
Alham Fikri Aji, Nikolay Bogoychev, Kenneth Heafield, Rico Sennrich,
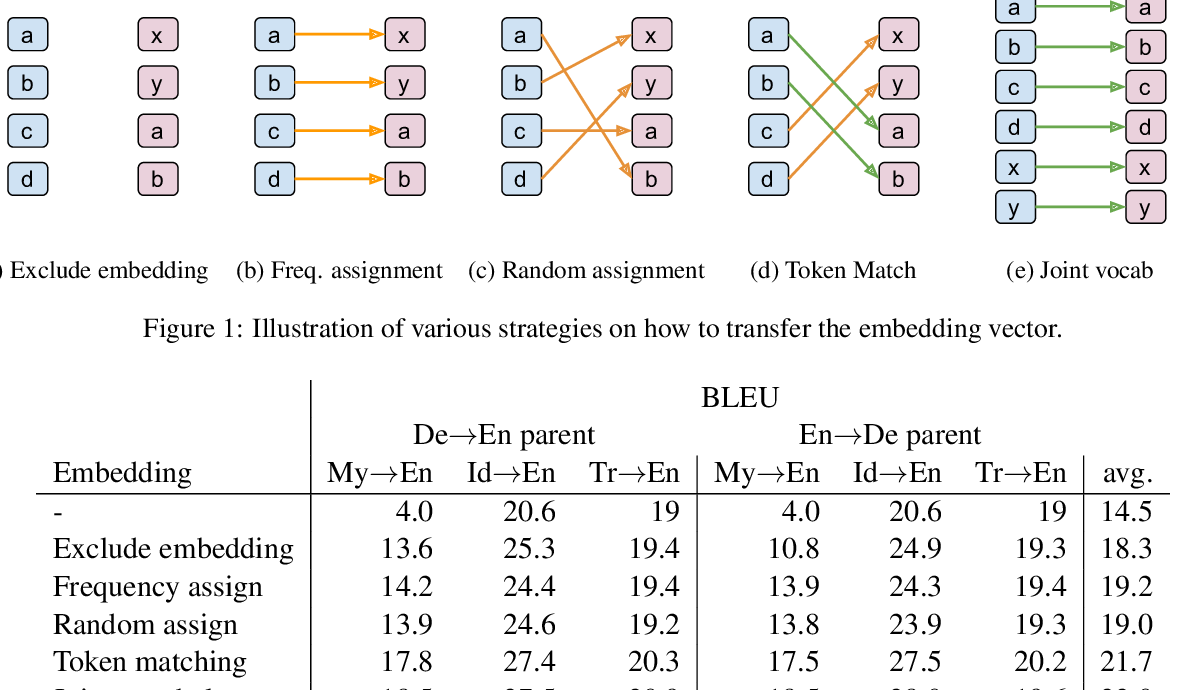
Simplify the Usage of Lexicon in Chinese NER
Ruotian Ma, Minlong Peng, Qi Zhang, Zhongyu Wei, Xuanjing Huang,
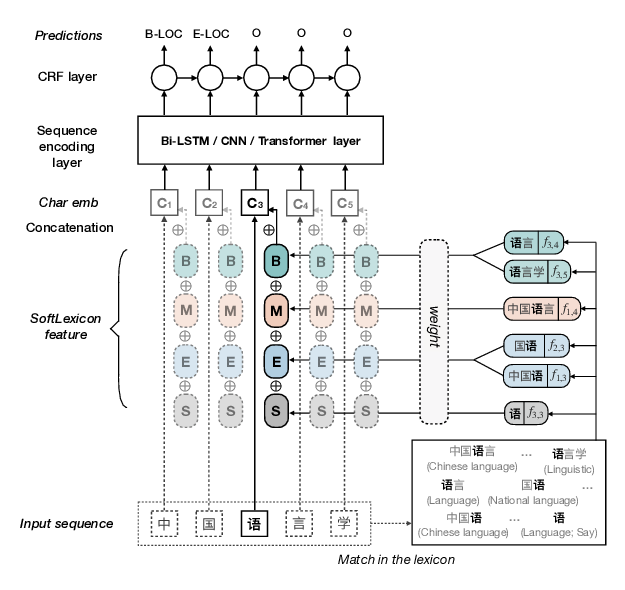
Hypernymy Detection for Low-Resource Languages via Meta Learning
Changlong Yu, Jialong Han, Haisong Zhang, Wilfred Ng,
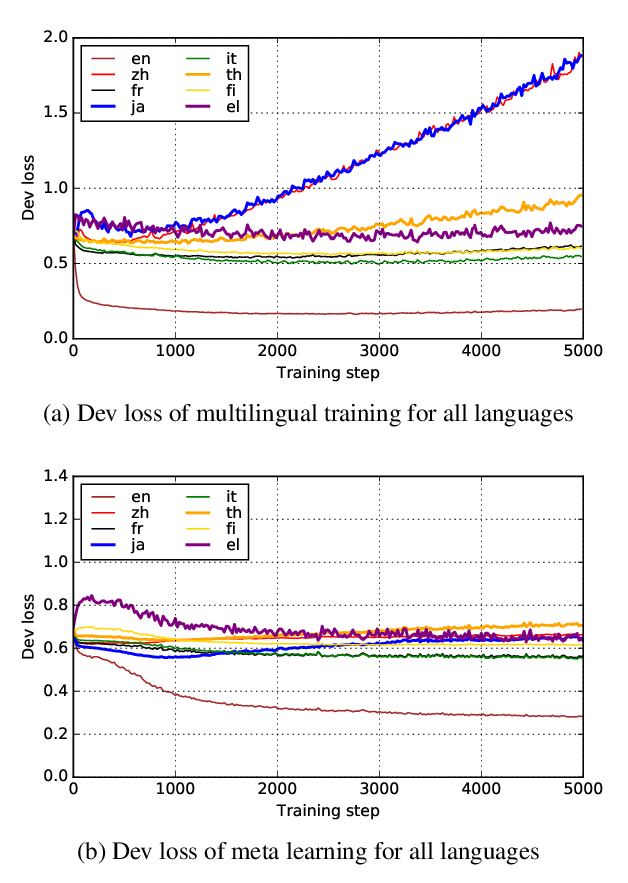
Multi-Domain Named Entity Recognition with Genre-Aware and Agnostic Inference
Jing Wang, Mayank Kulkarni, Daniel Preotiuc-Pietro,
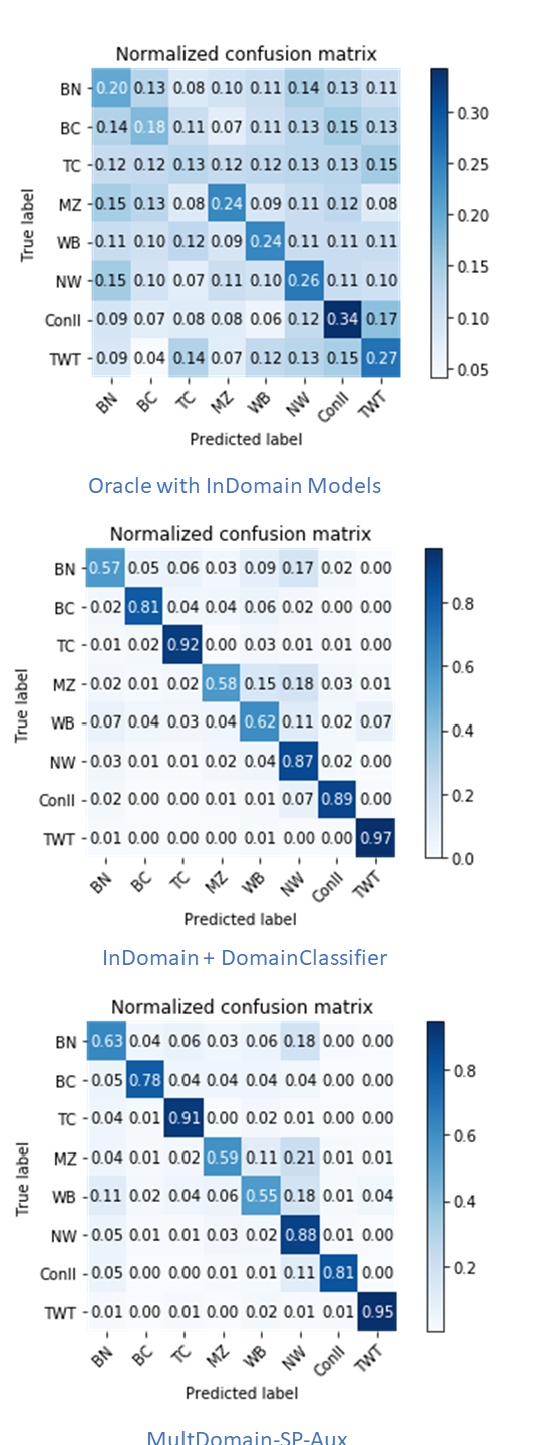