Curriculum Learning for Natural Language Understanding
Benfeng Xu, Licheng Zhang, Zhendong Mao, Quan Wang, Hongtao Xie, Yongdong Zhang
Semantics: Textual Inference and Other Areas of Semantics Long Paper
Session 11A: Jul 8
(05:00-06:00 GMT)
Session 13A: Jul 8
(12:00-13:00 GMT)
Abstract:
With the great success of pre-trained language models, the pretrain-finetune paradigm now becomes the undoubtedly dominant solution for natural language understanding (NLU) tasks. At the fine-tune stage, target task data is usually introduced in a completely random order and treated equally. However, examples in NLU tasks can vary greatly in difficulty, and similar to human learning procedure, language models can benefit from an easy-to-difficult curriculum. Based on this idea, we propose our Curriculum Learning approach. By reviewing the trainset in a crossed way, we are able to distinguish easy examples from difficult ones, and arrange a curriculum for language models. Without any manual model architecture design or use of external data, our Curriculum Learning approach obtains significant and universal performance improvements on a wide range of NLU tasks.
You can open the
pre-recorded video
in a separate window.
NOTE: The SlidesLive video may display a random order of the authors.
The correct author list is shown at the top of this webpage.
Similar Papers
Towards Unsupervised Language Understanding and Generation by Joint Dual Learning
Shang-Yu Su, Chao-Wei Huang, Yun-Nung Chen,
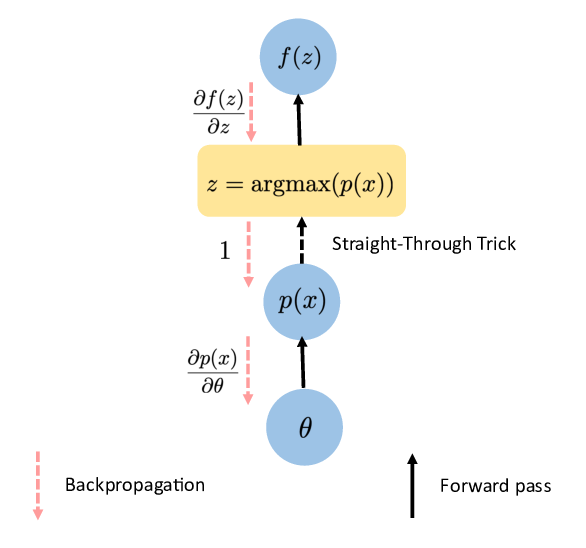
Learning to Customize Model Structures for Few-shot Dialogue Generation Tasks
Yiping Song, Zequn Liu, Wei Bi, Rui Yan, Ming Zhang,
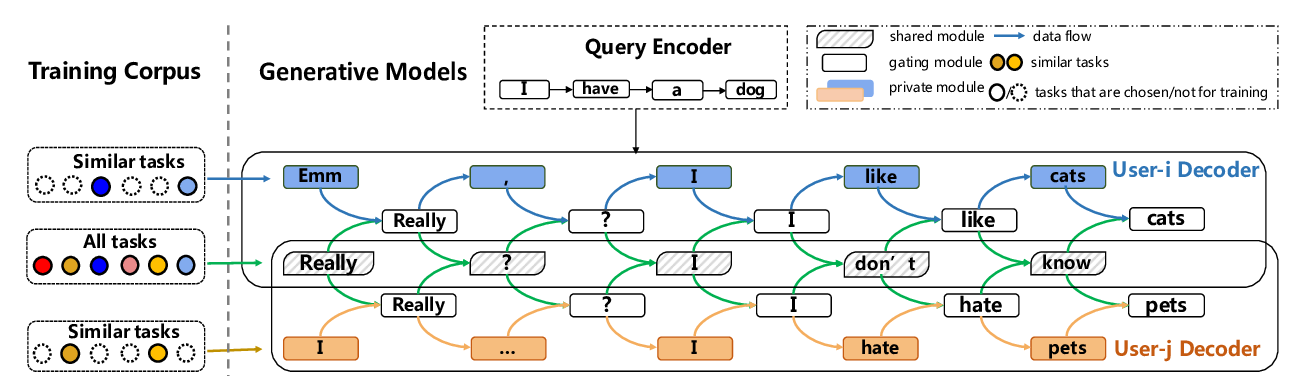
KLEJ: Comprehensive Benchmark for Polish Language Understanding
Piotr Rybak, Robert Mroczkowski, Janusz Tracz, Ireneusz Gawlik,
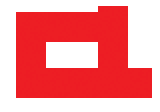
SMART: Robust and Efficient Fine-Tuning for Pre-trained Natural Language Models through Principled Regularized Optimization
Haoming Jiang, Pengcheng He, Weizhu Chen, Xiaodong Liu, Jianfeng Gao, Tuo Zhao,
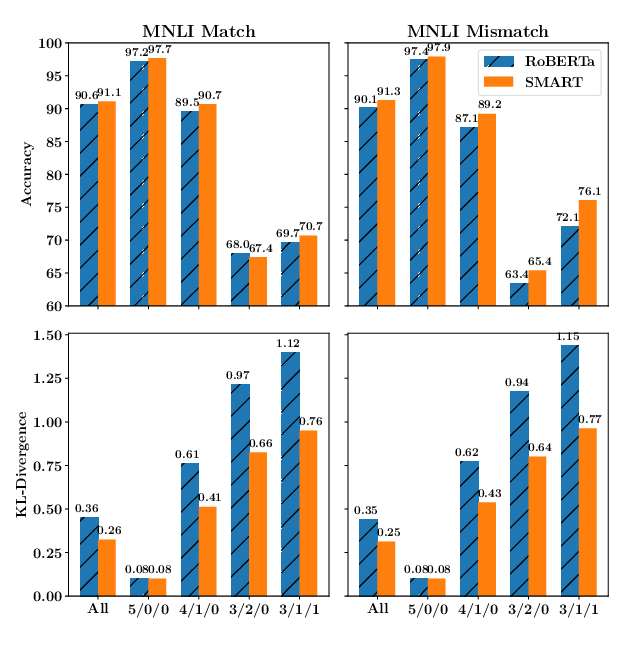