Response-Anticipated Memory for On-Demand Knowledge Integration in Response Generation
Zhiliang Tian, Wei Bi, Dongkyu Lee, Lanqing Xue, Yiping Song, Xiaojiang Liu, Nevin L. Zhang
Dialogue and Interactive Systems Long Paper
Session 1B: Jul 6
(06:00-07:00 GMT)
Session 2A: Jul 6
(08:00-09:00 GMT)
Abstract:
Neural conversation models are known to generate appropriate but non-informative responses in general. A scenario where informativeness can be significantly enhanced is Conversing by Reading (CbR), where conversations take place with respect to a given external document. In previous work, the external document is utilized by (1) creating a context-aware document memory that integrates information from the document and the conversational context, and then (2) generating responses referring to the memory. In this paper, we propose to create the document memory with some anticipated responses in mind. This is achieved using a teacher-student framework. The teacher is given the external document, the context, and the ground-truth response, and learns how to build a response-aware document memory from three sources of information. The student learns to construct a response-anticipated document memory from the first two sources, and teacher’s insight on memory creation. Empirical results show that our model outperforms the previous state-of-the-art for the CbR task.
You can open the
pre-recorded video
in a separate window.
NOTE: The SlidesLive video may display a random order of the authors.
The correct author list is shown at the top of this webpage.
Similar Papers
PeTra: A Sparsely Supervised Memory Model for People Tracking
Shubham Toshniwal, Allyson Ettinger, Kevin Gimpel, Karen Livescu,
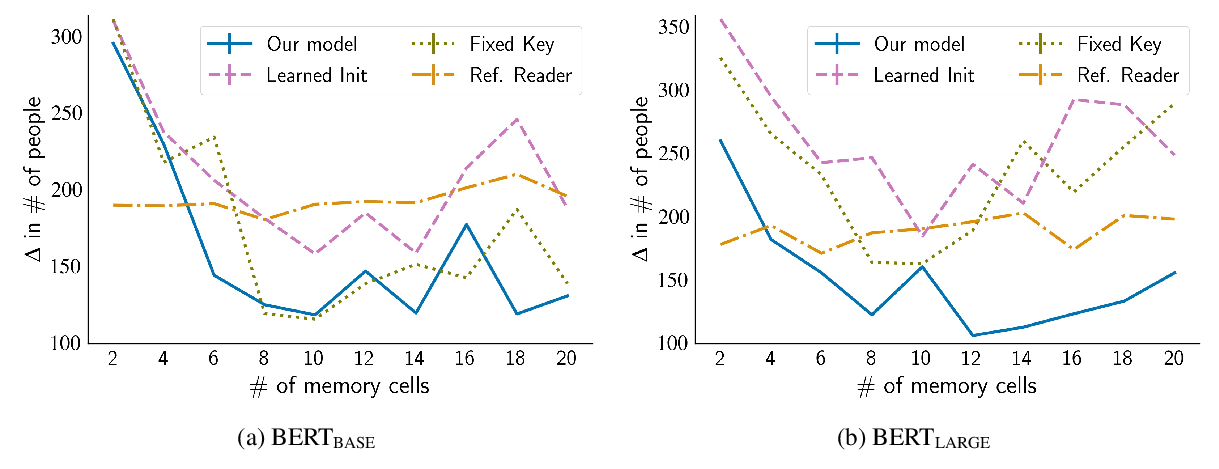
Reasoning with Latent Structure Refinement for Document-Level Relation Extraction
Guoshun Nan, Zhijiang Guo, Ivan Sekulic, Wei Lu,
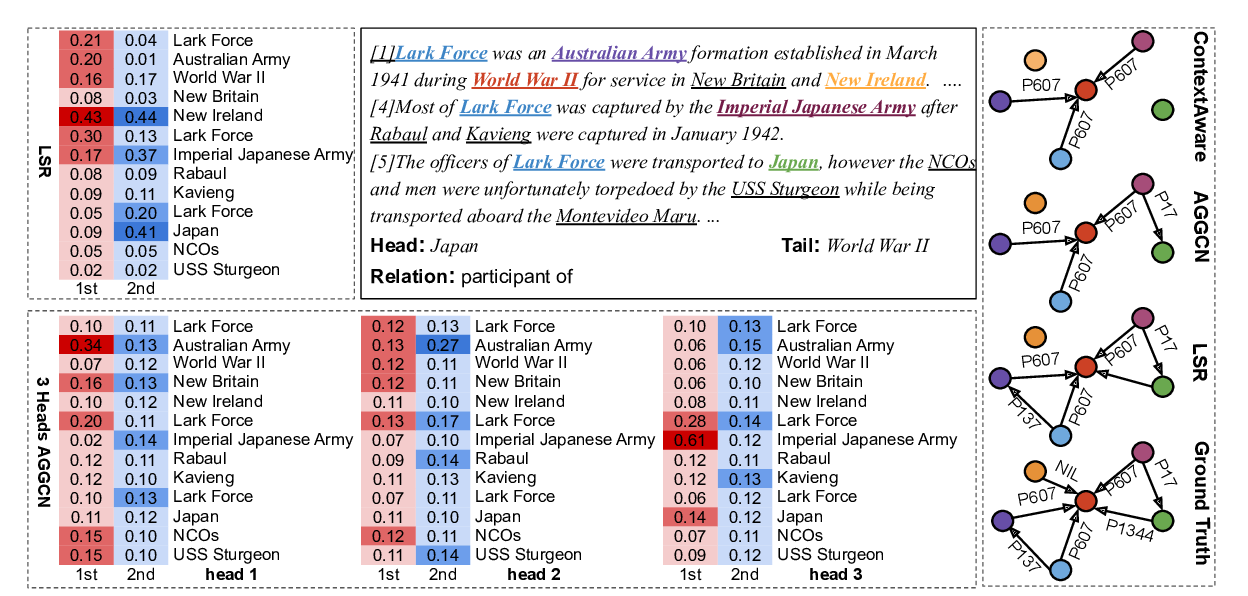
SciREX: A Challenge Dataset for Document-Level Information Extraction
Sarthak Jain, Madeleine van Zuylen, Hannaneh Hajishirzi, Iz Beltagy,
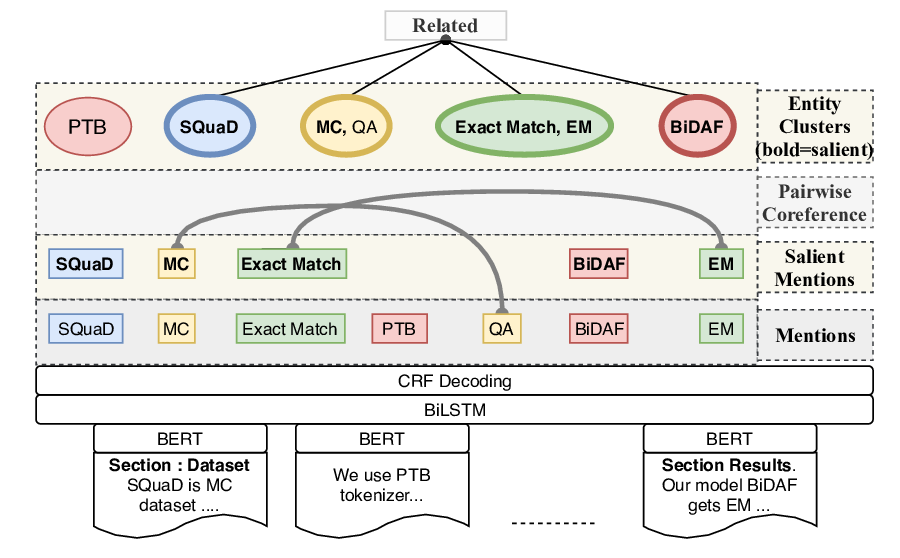