Representation Learning for Information Extraction from Form-like Documents
Bodhisattwa Prasad Majumder, Navneet Potti, Sandeep Tata, James Bradley Wendt, Qi Zhao, Marc Najork
Information Extraction Long Paper
Session 11B: Jul 8
(06:00-07:00 GMT)
Session 15B: Jul 8
(21:00-22:00 GMT)
Abstract:
We propose a novel approach using representation learning for tackling the problem of extracting structured information from form-like document images. We propose an extraction system that uses knowledge of the types of the target fields to generate extraction candidates and a neural network architecture that learns a dense representation of each candidate based on neighboring words in the document. These learned representations are not only useful in solving the extraction task for unseen document templates from two different domains but are also interpretable, as we show using loss cases.
You can open the
pre-recorded video
in a separate window.
NOTE: The SlidesLive video may display a random order of the authors.
The correct author list is shown at the top of this webpage.
Similar Papers
Effective Inter-Clause Modeling for End-to-End Emotion-Cause Pair Extraction
Penghui Wei, Jiahao Zhao, Wenji Mao,
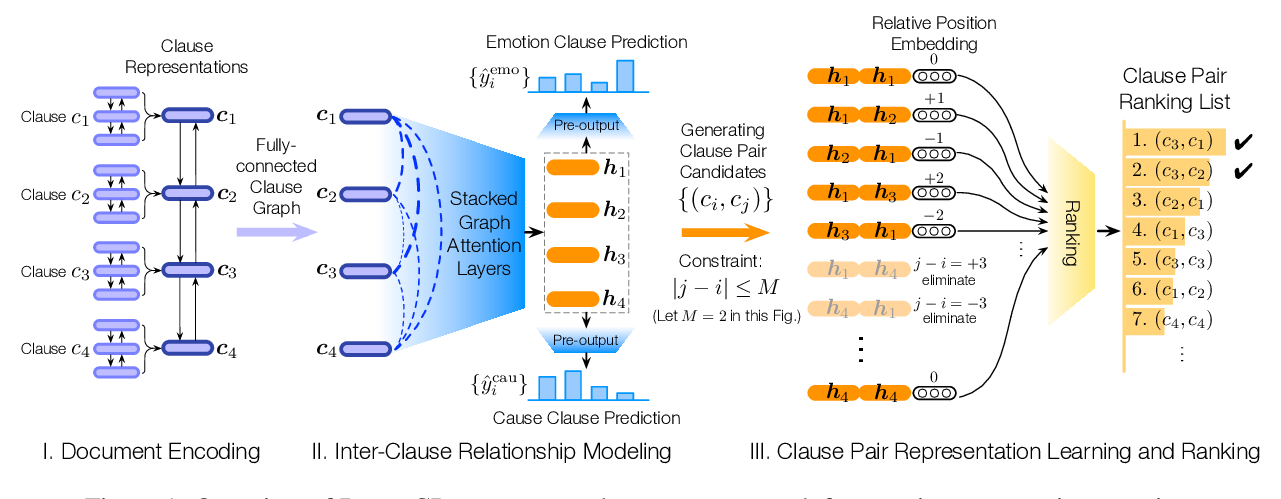
Better Document-level Machine Translation with Bayes' Rule
Lei Yu, Laurent Sartran, Wojciech Stokowiec, Wang Ling, Lingpeng Kong, Phil Blunsom, Chris Dyer,
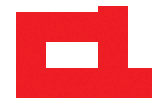
Every Document Owns Its Structure: Inductive Text Classification via Graph Neural Networks
Yufeng Zhang, Xueli Yu, Zeyu Cui, Shu Wu, Zhongzhen Wen, Liang Wang,
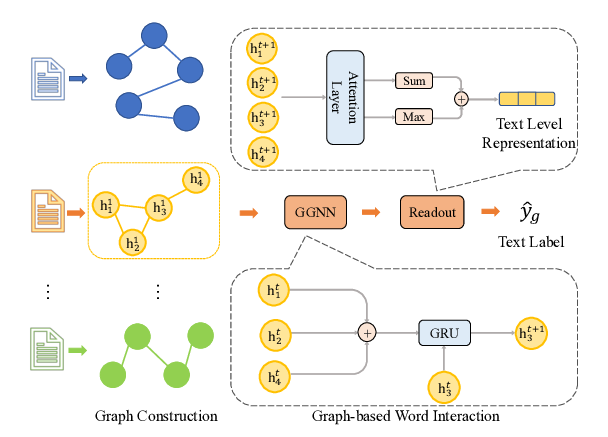
SciREX: A Challenge Dataset for Document-Level Information Extraction
Sarthak Jain, Madeleine van Zuylen, Hannaneh Hajishirzi, Iz Beltagy,
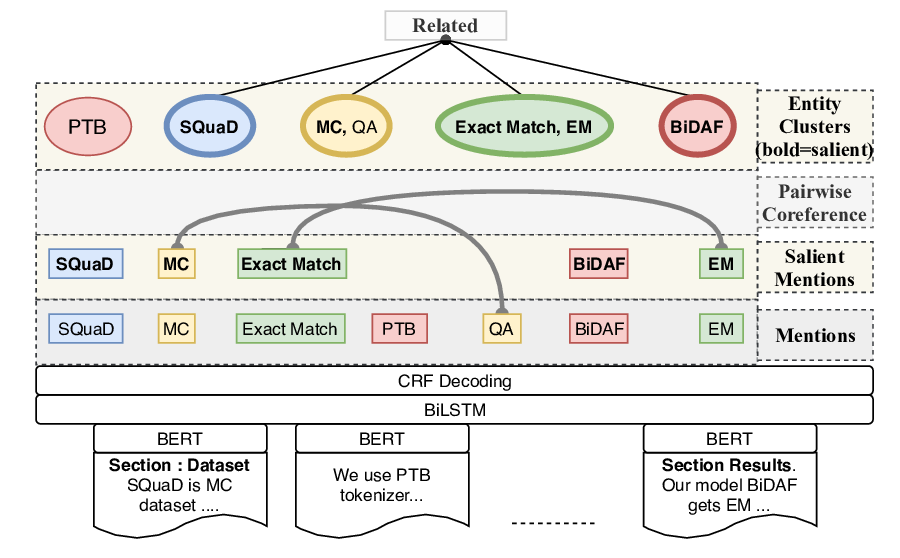