Abstract:
The main barrier to progress in the task of Formality Style Transfer is the inadequacy of training data. In this paper, we study how to augment parallel data and propose novel and simple data augmentation methods for this task to obtain useful sentence pairs with easily accessible models and systems. Experiments demonstrate that our augmented parallel data largely helps improve formality style transfer when it is used to pre-train the model, leading to the state-of-the-art results in the GYAFC benchmark dataset.
You can open the
pre-recorded video
in a separate window.
NOTE: The SlidesLive video may display a random order of the authors.
The correct author list is shown at the top of this webpage.
Similar Papers
Exploring Contextual Word-level Style Relevance for Unsupervised Style Transfer
Chulun Zhou, Liangyu Chen, Jiachen Liu, Xinyan Xiao, Jinsong Su, Sheng Guo, Hua Wu,
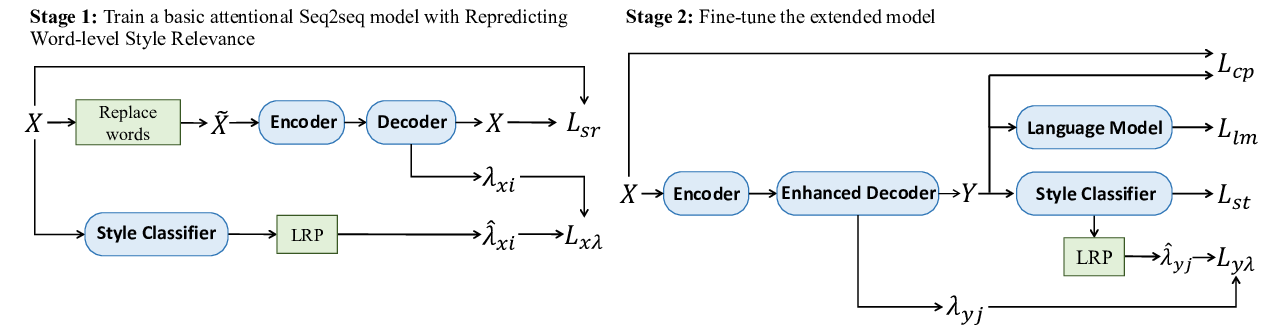
Politeness Transfer: A Tag and Generate Approach
Aman Madaan, Amrith Setlur, Tanmay Parekh, Barnabas Poczos, Graham Neubig, Yiming Yang, Ruslan Salakhutdinov, Alan W Black, Shrimai Prabhumoye,

Learning Implicit Text Generation via Feature Matching
Inkit Padhi, Pierre Dognin, Ke Bai, Cícero Nogueira dos Santos, Vijil Chenthamarakshan, Youssef Mroueh, Payel Das,
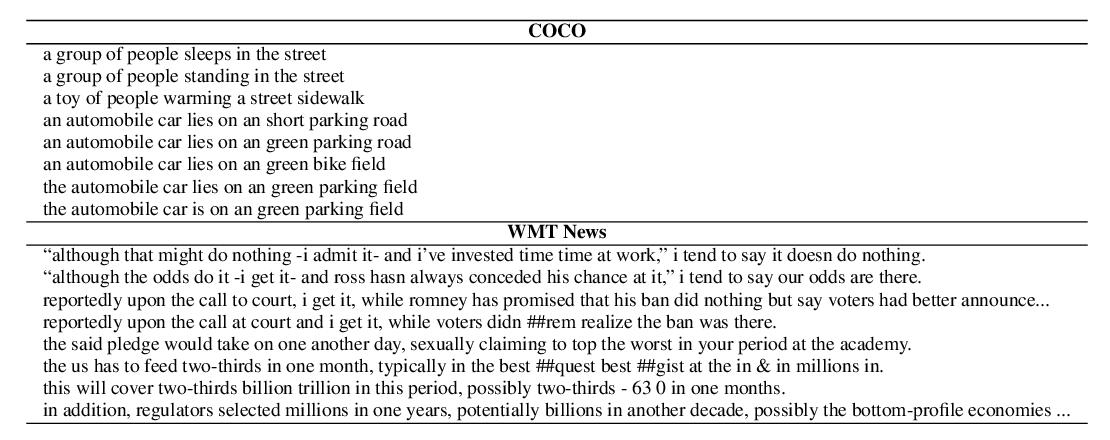