Enriched In-Order Linearization for Faster Sequence-to-Sequence Constituent Parsing
Daniel Fernández-González, Carlos Gómez-Rodríguez
Syntax: Tagging, Chunking and Parsing Short Paper
Session 7A: Jul 7
(08:00-09:00 GMT)
Session 8A: Jul 7
(12:00-13:00 GMT)
Abstract:
Sequence-to-sequence constituent parsing requires a linearization to represent trees as sequences. Top-down tree linearizations, which can be based on brackets or shift-reduce actions, have achieved the best accuracy to date. In this paper, we show that these results can be improved by using an in-order linearization instead. Based on this observation, we implement an enriched in-order shift-reduce linearization inspired by Vinyals et al. (2015)'s approach, achieving the best accuracy to date on the English PTB dataset among fully-supervised single-model sequence-to-sequence constituent parsers. Finally, we apply deterministic attention mechanisms to match the speed of state-of-the-art transition-based parsers, thus showing that sequence-to-sequence models can match them, not only in accuracy, but also in speed.
You can open the
pre-recorded video
in a separate window.
NOTE: The SlidesLive video may display a random order of the authors.
The correct author list is shown at the top of this webpage.
Similar Papers
Transition-based Semantic Dependency Parsing with Pointer Networks
Daniel Fernández-González, Carlos Gómez-Rodríguez,
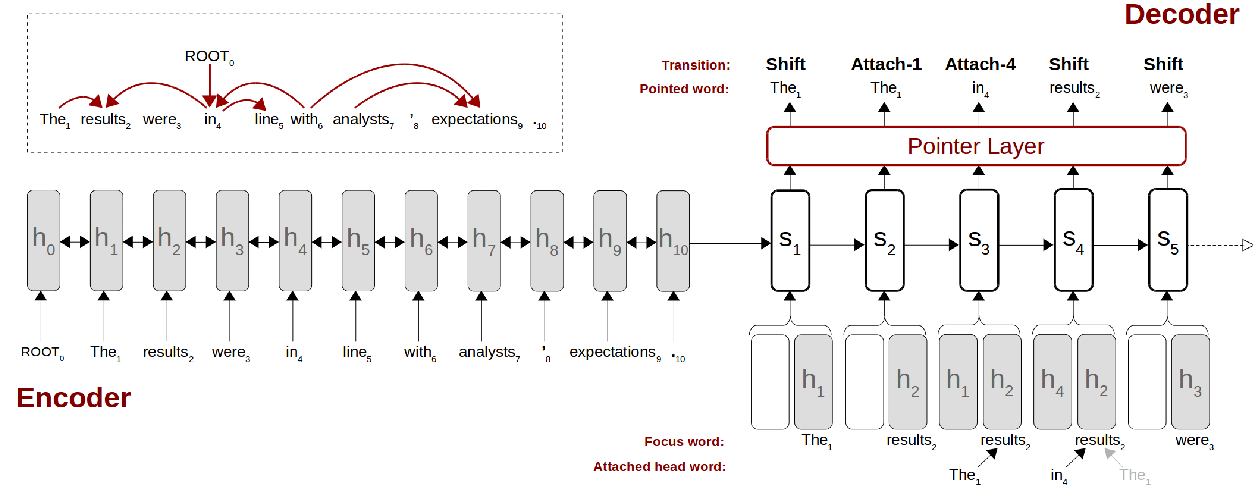
A Complete Shift-Reduce Chinese Discourse Parser with Robust Dynamic Oracle
Shyh-Shiun Hung, Hen-Hsen Huang, Hsin-Hsi Chen,
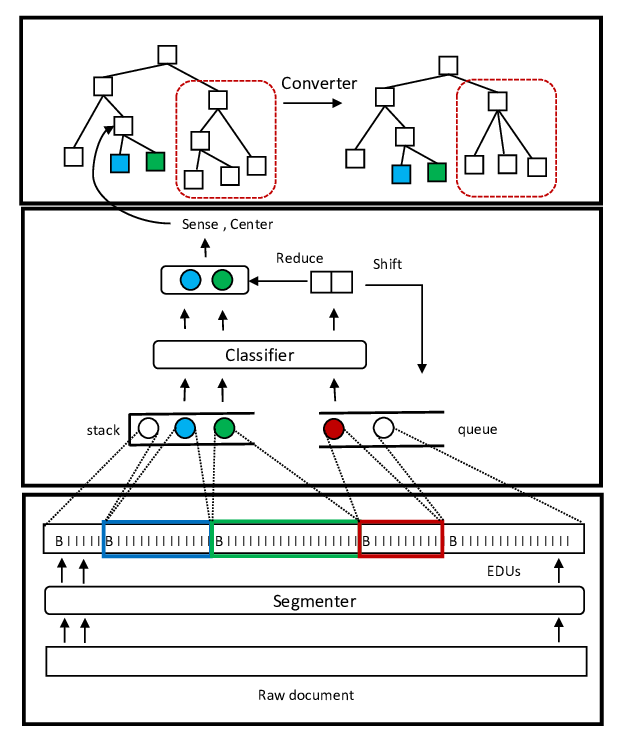
SeqVAT: Virtual Adversarial Training for Semi-Supervised Sequence Labeling
Luoxin Chen, Weitong Ruan, Xinyue Liu, Jianhua Lu,
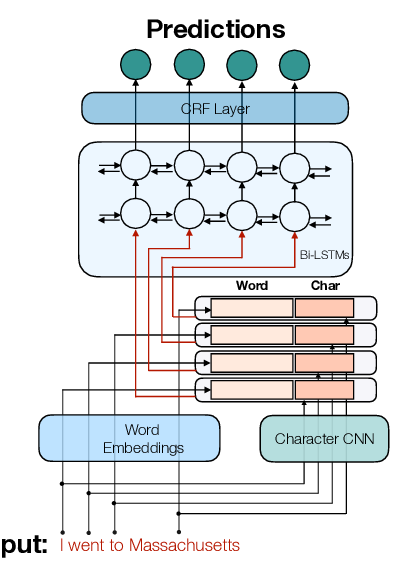