Make Up Your Mind! Adversarial Generation of Inconsistent Natural Language Explanations
Oana-Maria Camburu, Brendan Shillingford, Pasquale Minervini, Thomas Lukasiewicz, Phil Blunsom
Interpretability and Analysis of Models for NLP Short Paper
Session 7B: Jul 7
(09:00-10:00 GMT)
Session 8A: Jul 7
(12:00-13:00 GMT)
Abstract:
To increase trust in artificial intelligence systems, a promising research direction consists of designing neural models capable of generating natural language explanations for their predictions. In this work, we show that such models are nonetheless prone to generating mutually inconsistent explanations, such as ''Because there is a dog in the image.'' and ''Because there is no dog in the [same] image.'', exposing flaws in either the decision-making process of the model or in the generation of the explanations. We introduce a simple yet effective adversarial framework for sanity checking models against the generation of inconsistent natural language explanations. Moreover, as part of the framework, we address the problem of adversarial attacks with full target sequences, a scenario that was not previously addressed in sequence-to-sequence attacks. Finally, we apply our framework on a state-of-the-art neural natural language inference model that provides natural language explanations for its predictions. Our framework shows that this model is capable of generating a significant number of inconsistent explanations.
You can open the
pre-recorded video
in a separate window.
NOTE: The SlidesLive video may display a random order of the authors.
The correct author list is shown at the top of this webpage.
Similar Papers
NILE : Natural Language Inference with Faithful Natural Language Explanations
Sawan Kumar, Partha Talukdar,
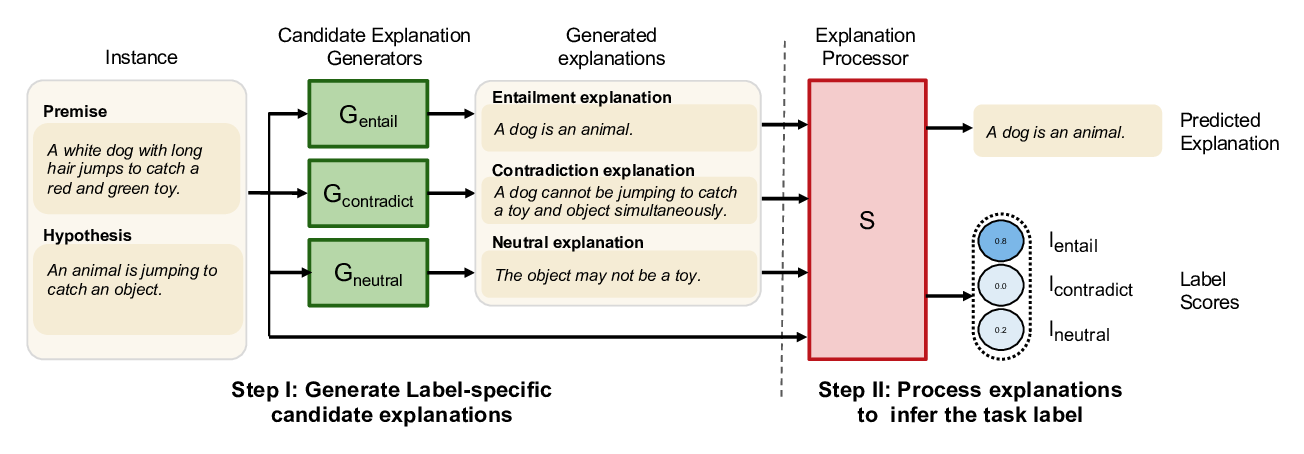
A Geometry-Inspired Attack for Generating Natural Language Adversarial Examples
Zhao Meng, Roger Wattenhofer,
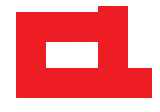
Climbing towards NLU: On Meaning, Form, and Understanding in the Age of Data
Emily M. Bender, Alexander Koller,
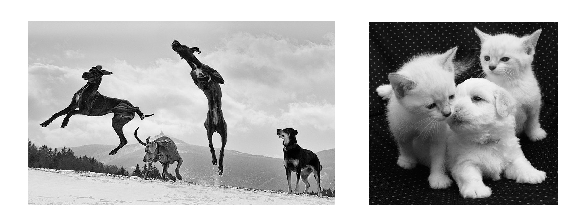
Generating Hierarchical Explanations on Text Classification via Feature Interaction Detection
Hanjie Chen, Guangtao Zheng, Yangfeng Ji,
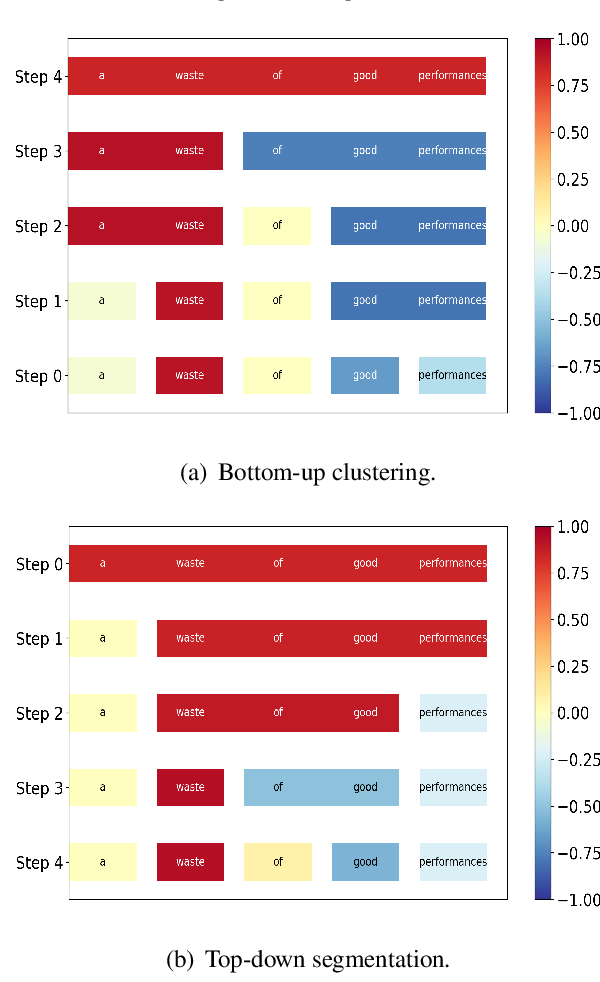