Learning to Deceive with Attention-Based Explanations
Danish Pruthi, Mansi Gupta, Bhuwan Dhingra, Graham Neubig, Zachary C. Lipton
Interpretability and Analysis of Models for NLP Long Paper
Session 9A: Jul 7
(17:00-18:00 GMT)
Session 10B: Jul 7
(21:00-22:00 GMT)
Abstract:
Attention mechanisms are ubiquitous components in neural architectures applied to natural language processing. In addition to yielding gains in predictive accuracy, attention weights are often claimed to confer interpretability, purportedly useful both for providing insights to practitioners and for explaining why a model makes its decisions to stakeholders. We call the latter use of attention mechanisms into question by demonstrating a simple method for training models to produce deceptive attention masks. Our method diminishes the total weight assigned to designated impermissible tokens, even when the models can be shown to nevertheless rely on these features to drive predictions. Across multiple models and tasks, our approach manipulates attention weights while paying surprisingly little cost in accuracy. Through a human study, we show that our manipulated attention-based explanations deceive people into thinking that predictions from a model biased against gender minorities do not rely on the gender. Consequently, our results cast doubt on attention’s reliability as a tool for auditing algorithms in the context of fairness and accountability.
You can open the
pre-recorded video
in a separate window.
NOTE: The SlidesLive video may display a random order of the authors.
The correct author list is shown at the top of this webpage.
Similar Papers
Human Attention Maps for Text Classification: Do Humans and Neural Networks Focus on the Same Words?
Cansu Sen, Thomas Hartvigsen, Biao Yin, Xiangnan Kong, Elke Rundensteiner,
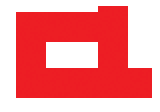
Towards Transparent and Explainable Attention Models
Akash Kumar Mohankumar, Preksha Nema, Sharan Narasimhan, Mitesh M. Khapra, Balaji Vasan Srinivasan, Balaraman Ravindran,
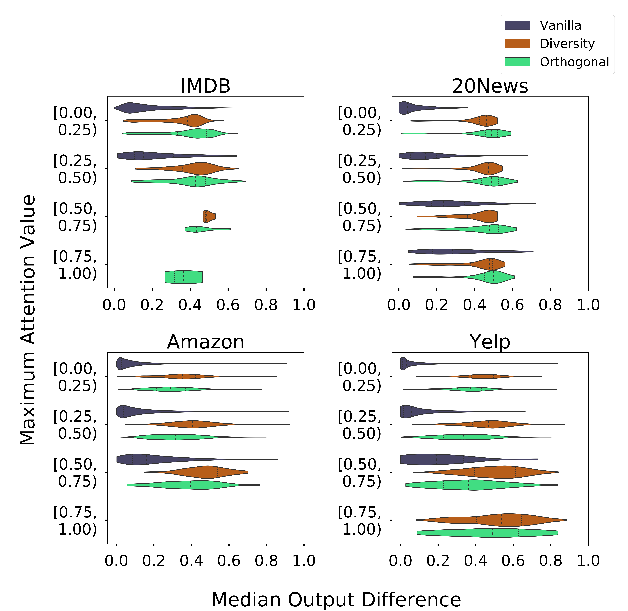