SMART: Robust and Efficient Fine-Tuning for Pre-trained Natural Language Models through Principled Regularized Optimization
Haoming Jiang, Pengcheng He, Weizhu Chen, Xiaodong Liu, Jianfeng Gao, Tuo Zhao
Machine Learning for NLP Long Paper
Session 4A: Jul 6
(17:00-18:00 GMT)
Session 5A: Jul 6
(20:00-21:00 GMT)
Abstract:
Transfer learning has fundamentally changed the landscape of natural language processing (NLP). Many state-of-the-art models are first pre-trained on a large text corpus and then fine-tuned on downstream tasks. However, due to limited data resources from downstream tasks and the extremely high complexity of pre-trained models, aggressive fine-tuning often causes the fine-tuned model to overfit the training data of downstream tasks and fail to generalize to unseen data. To address such an issue in a principled manner, we propose a new learning framework for robust and efficient fine-tuning for pre-trained models to attain better generalization performance. The proposed framework contains two important ingredients: 1. Smoothness-inducing regularization, which effectively manages the complexity of the model; 2. Bregman proximal point optimization, which is an instance of trust-region methods and can prevent aggressive updating. Our experiments show that the proposed framework achieves new state-of-the-art performance on a number of NLP tasks including GLUE, SNLI, SciTail and ANLI. Moreover, it also outperforms the state-of-the-art T5 model, which is the largest pre-trained model containing 11 billion parameters, on GLUE.
You can open the
pre-recorded video
in a separate window.
NOTE: The SlidesLive video may display a random order of the authors.
The correct author list is shown at the top of this webpage.
Similar Papers
Do you have the right scissors? Tailoring Pre-trained Language Models via Monte-Carlo Methods
Ning Miao, Yuxuan Song, Hao Zhou, Lei Li,
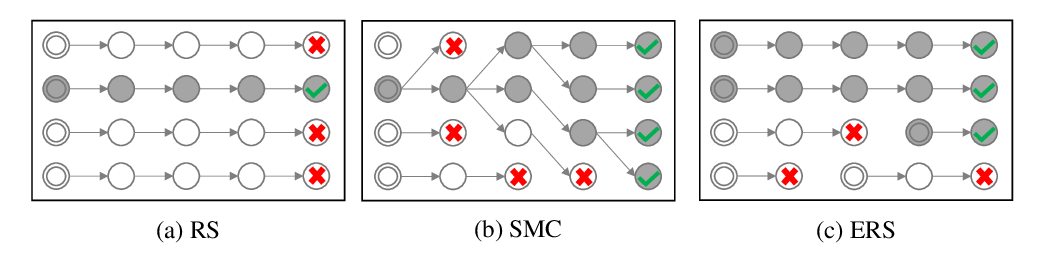
Pre-training Is (Almost) All You Need: An Application to Commonsense Reasoning
Alexandre Tamborrino, Nicola Pellicanò, Baptiste Pannier, Pascal Voitot, Louise Naudin,
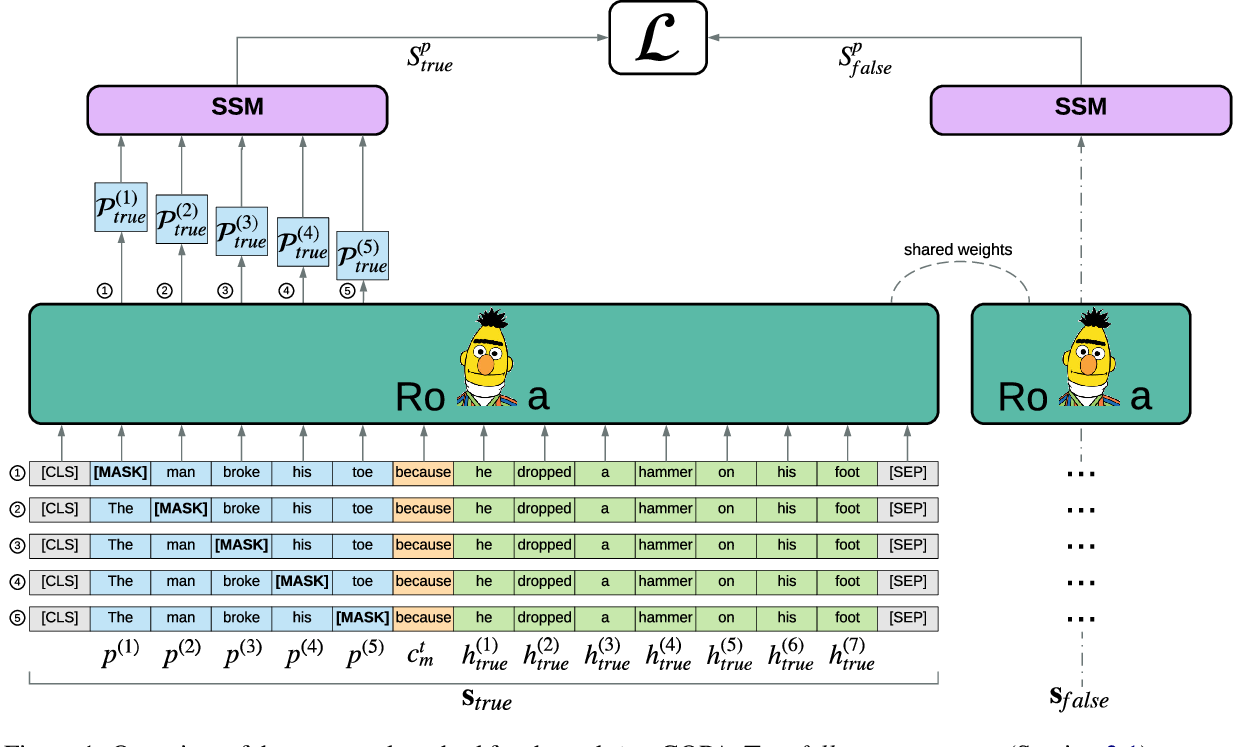
Integrating Multimodal Information in Large Pretrained Transformers
Wasifur Rahman, Md Kamrul Hasan, Sangwu Lee, AmirAli Bagher Zadeh, Chengfeng Mao, Louis-Philippe Morency, Ehsan Hoque,
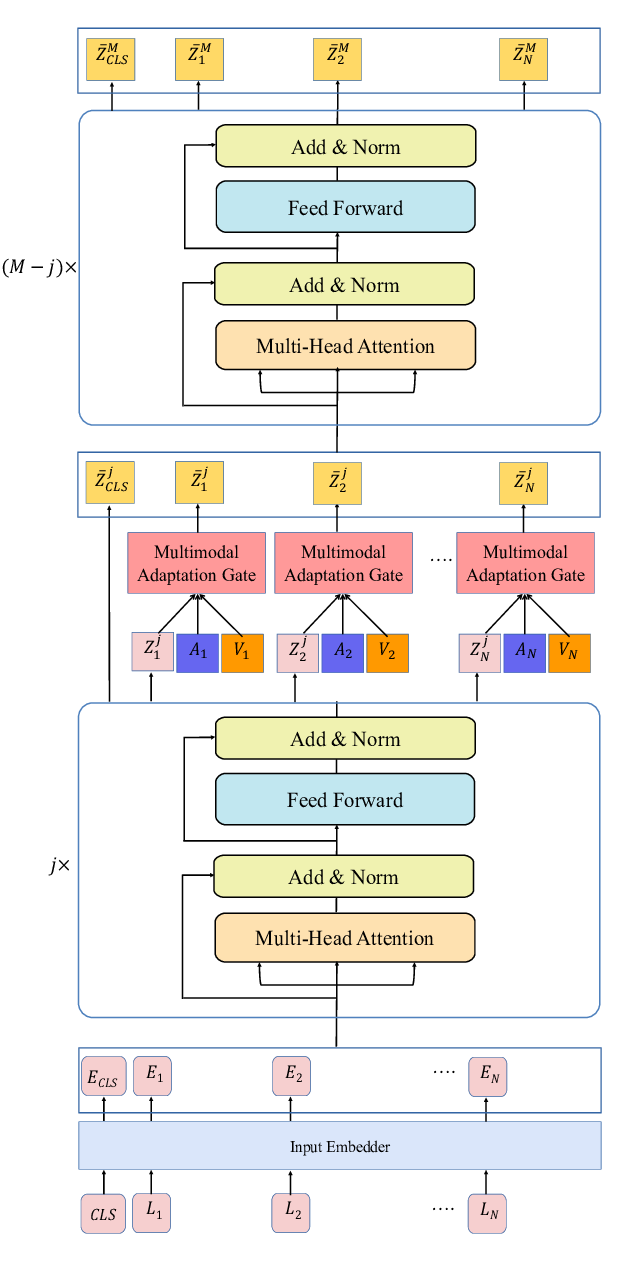