Fact-based Content Weighting for Evaluating Abstractive Summarisation
Xinnuo Xu, Ondřej Dušek, Jingyi Li, Verena Rieser, Ioannis Konstas
Summarization Short Paper
Session 9A: Jul 7
(17:00-18:00 GMT)
Session 10B: Jul 7
(21:00-22:00 GMT)
Abstract:
Abstractive summarisation is notoriously hard to evaluate since standard word-overlap-based metrics are insufficient. We introduce a new evaluation metric which is based on fact-level content weighting, i.e. relating the facts of the document to the facts of the summary. We fol- low the assumption that a good summary will reflect all relevant facts, i.e. the ones present in the ground truth (human-generated refer- ence summary). We confirm this hypothe- sis by showing that our weightings are highly correlated to human perception and compare favourably to the recent manual highlight- based metric of Hardy et al. (2019).
You can open the
pre-recorded video
in a separate window.
NOTE: The SlidesLive video may display a random order of the authors.
The correct author list is shown at the top of this webpage.
Similar Papers
FEQA: A Question Answering Evaluation Framework for Faithfulness Assessment in Abstractive Summarization
Esin Durmus, He He, Mona Diab,
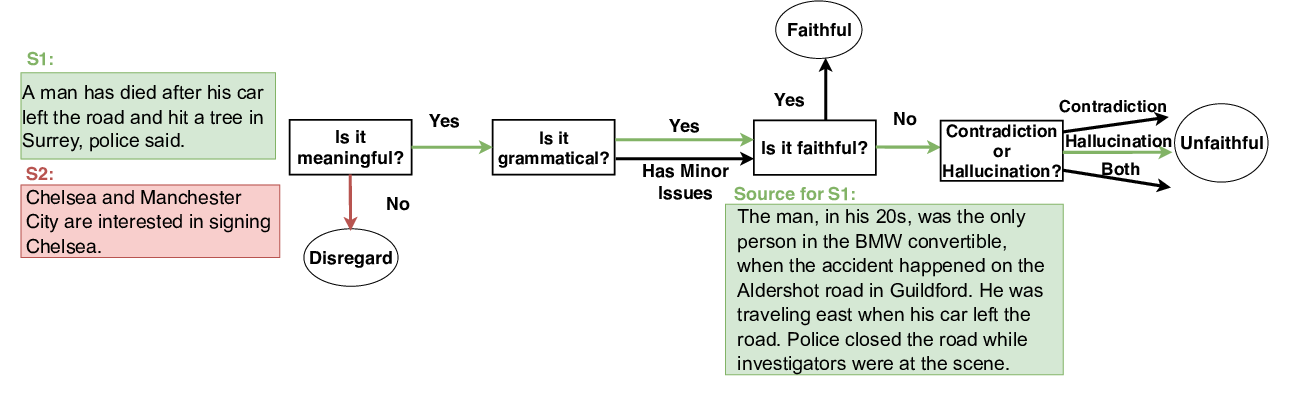
SUPERT: Towards New Frontiers in Unsupervised Evaluation Metrics for Multi-Document Summarization
Yang Gao, Wei Zhao, Steffen Eger,
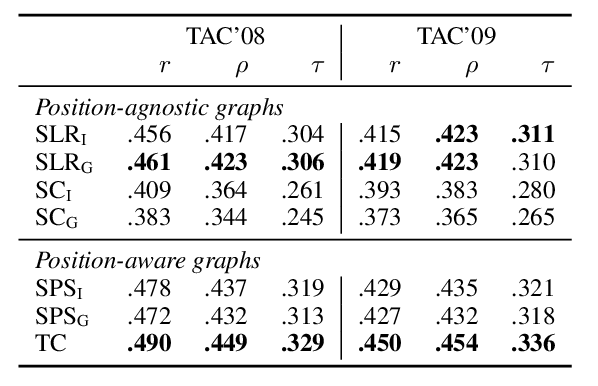
Asking and Answering Questions to Evaluate the Factual Consistency of Summaries
Alex Wang, Kyunghyun Cho, Mike Lewis,
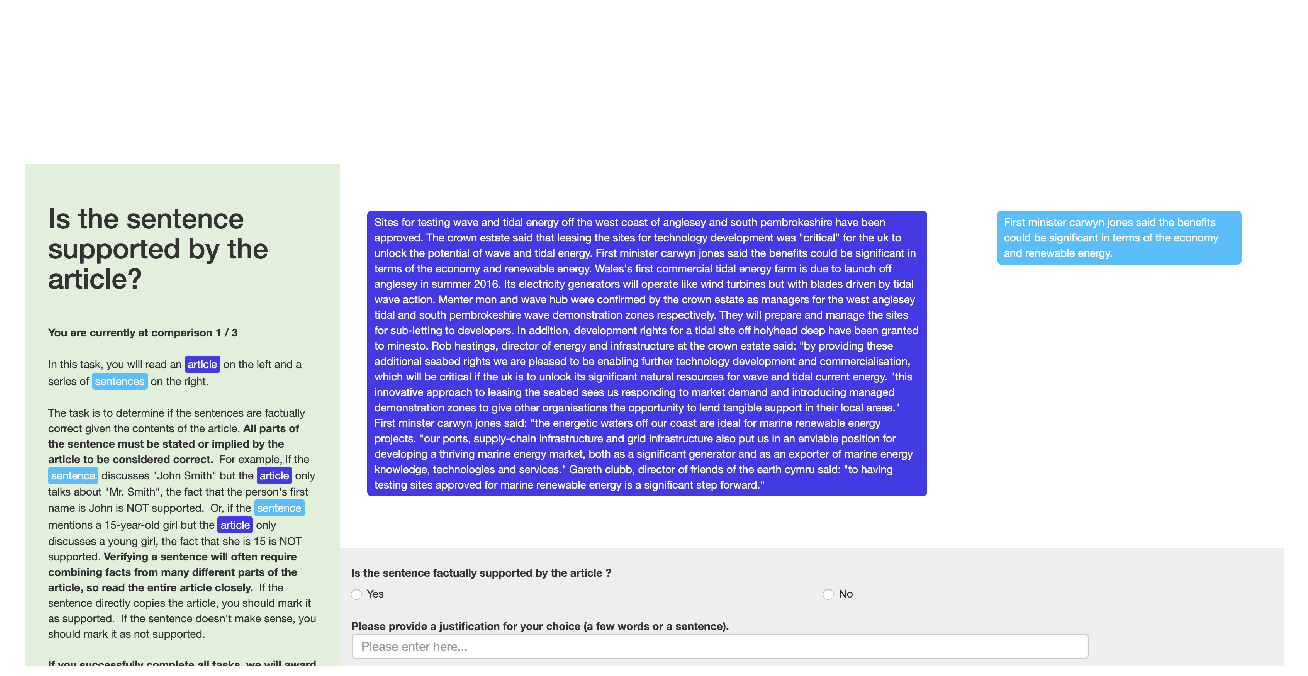
Towards Holistic and Automatic Evaluation of Open-Domain Dialogue Generation
Bo Pang, Erik Nijkamp, Wenjuan Han, Linqi Zhou, Yixian Liu, Kewei Tu,
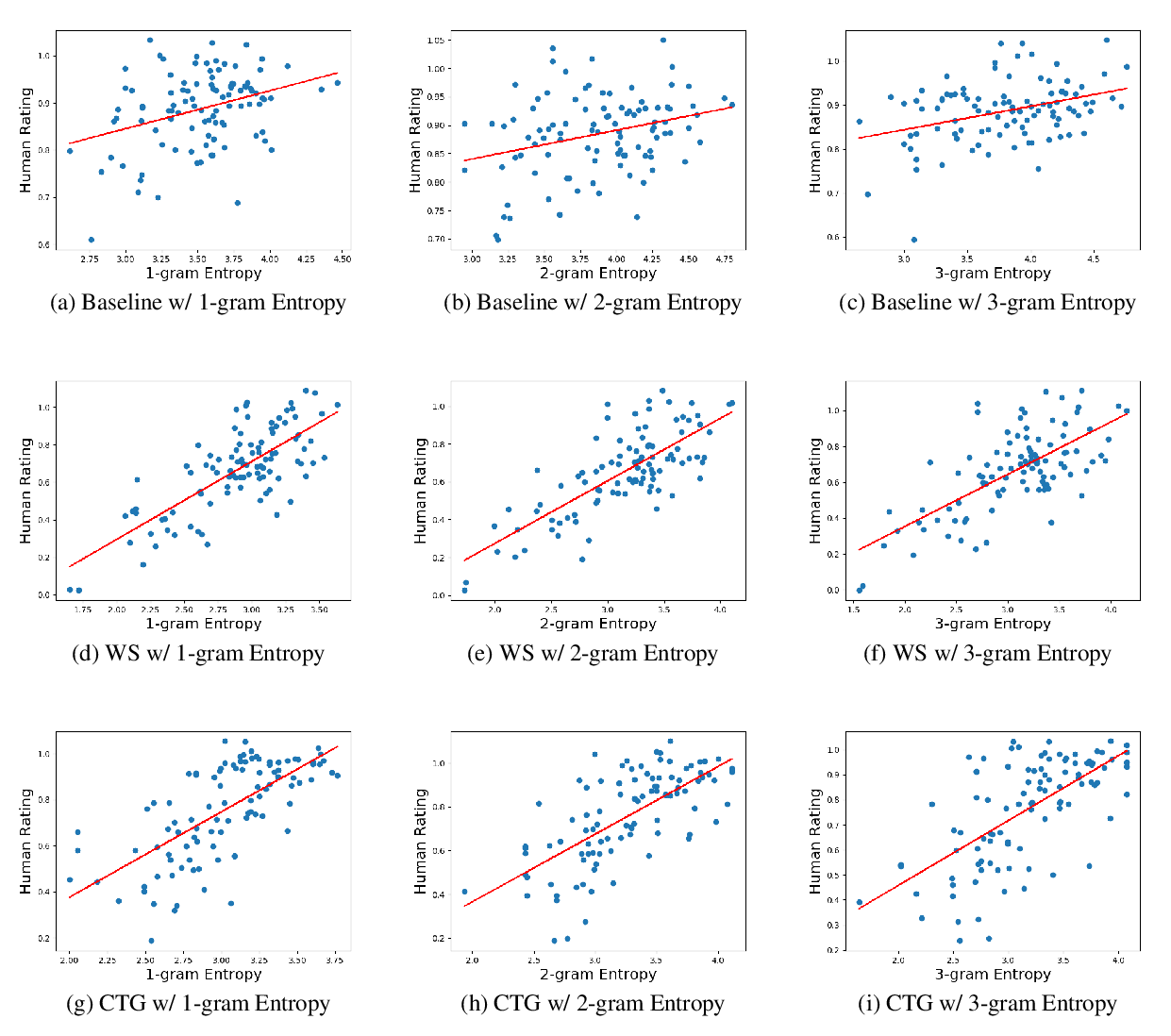