Unsupervised Opinion Summarization as Copycat-Review Generation
Arthur Bražinskas, Mirella Lapata, Ivan Titov
Summarization Long Paper
Session 9A: Jul 7
(17:00-18:00 GMT)
Session 10B: Jul 7
(21:00-22:00 GMT)
Abstract:
Opinion summarization is the task of automatically creating summaries that reflect subjective information expressed in multiple documents, such as product reviews. While the majority of previous work has focused on the extractive setting, i.e., selecting fragments from input reviews to produce a summary, we let the model generate novel sentences and hence produce abstractive summaries. Recent progress in summarization has seen the development of supervised models which rely on large quantities of document-summary pairs. Since such training data is expensive to acquire, we instead consider the unsupervised setting, in other words, we do not use any summaries in training. We define a generative model for a review collection which capitalizes on the intuition that when generating a new review given a set of other reviews of a product, we should be able to control the “amount of novelty” going into the new review or, equivalently, vary the extent to which it deviates from the input. At test time, when generating summaries, we force the novelty to be minimal, and produce a text reflecting consensus opinions. We capture this intuition by defining a hierarchical variational autoencoder model. Both individual reviews and the products they correspond to are associated with stochastic latent codes, and the review generator (“decoder”) has direct access to the text of input reviews through the pointer-generator mechanism. Experiments on Amazon and Yelp datasets, show that setting at test time the review’s latent code to its mean, allows the model to produce fluent and coherent summaries reflecting common opinions.
You can open the
pre-recorded video
in a separate window.
NOTE: The SlidesLive video may display a random order of the authors.
The correct author list is shown at the top of this webpage.
Similar Papers
OpinionDigest: A Simple Framework for Opinion Summarization
Yoshihiko Suhara, Xiaolan Wang, Stefanos Angelidis, Wang-Chiew Tan,
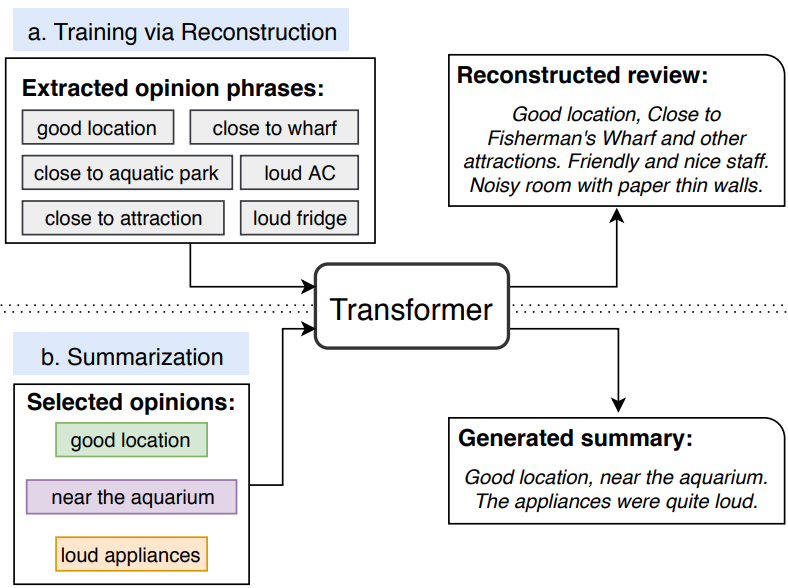
Leveraging Graph to Improve Abstractive Multi-Document Summarization
Wei Li, Xinyan Xiao, Jiachen Liu, Hua Wu, Haifeng Wang, Junping Du,
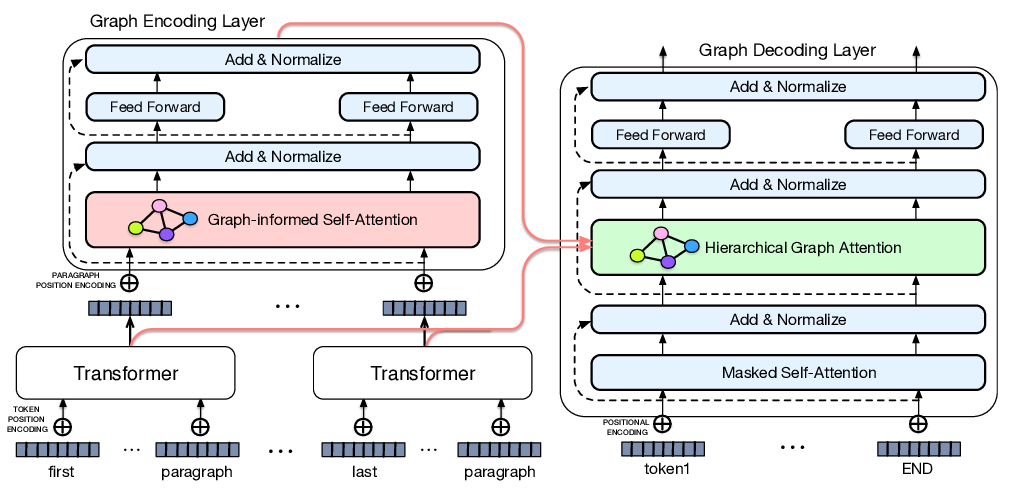
On Faithfulness and Factuality in Abstractive Summarization
Joshua Maynez, Shashi Narayan, Bernd Bohnet, Ryan McDonald,
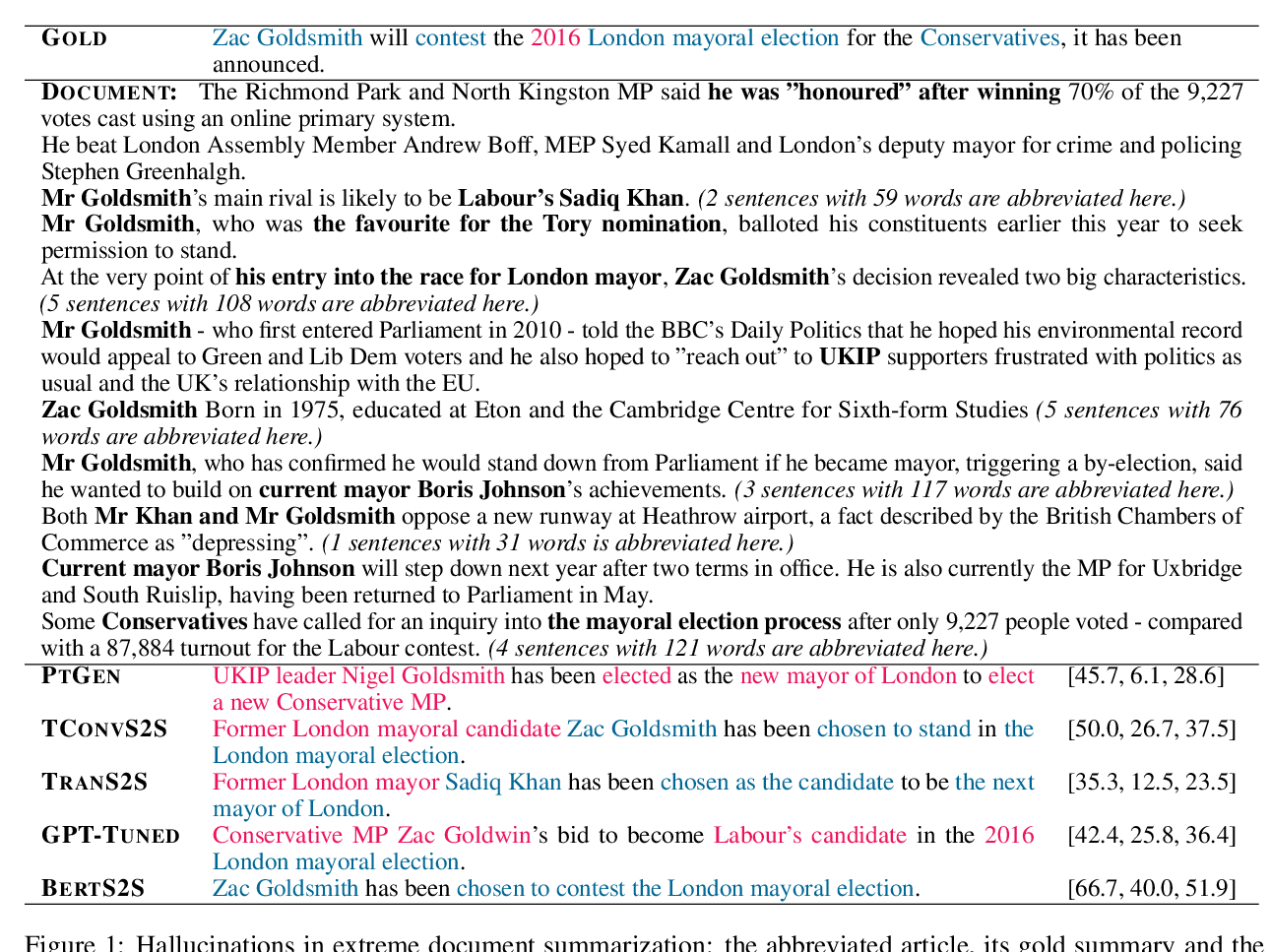