ZPR2: Joint Zero Pronoun Recovery and Resolution using Multi-Task Learning and BERT
Linfeng Song, Kun Xu, Yue Zhang, Jianshu Chen, Dong Yu
Discourse and Pragmatics Short Paper
Session 9B: Jul 7
(18:00-19:00 GMT)
Session 10B: Jul 7
(21:00-22:00 GMT)
Abstract:
Zero pronoun recovery and resolution aim at recovering the dropped pronoun and pointing out its anaphoric mentions, respectively. We propose to better explore their interaction by solving both tasks together, while the previous work treats them separately. For zero pronoun resolution, we study this task in a more realistic setting, where no parsing trees or only automatic trees are available, while most previous work assumes gold trees. Experiments on two benchmarks show that joint modeling significantly outperforms our baseline that already beats the previous state of the arts.
You can open the
pre-recorded video
in a separate window.
NOTE: The SlidesLive video may display a random order of the authors.
The correct author list is shown at the top of this webpage.
Similar Papers
An Empirical Comparison of Unsupervised Constituency Parsing Methods
Jun Li, Yifan Cao, Jiong Cai, Yong Jiang, Kewei Tu,
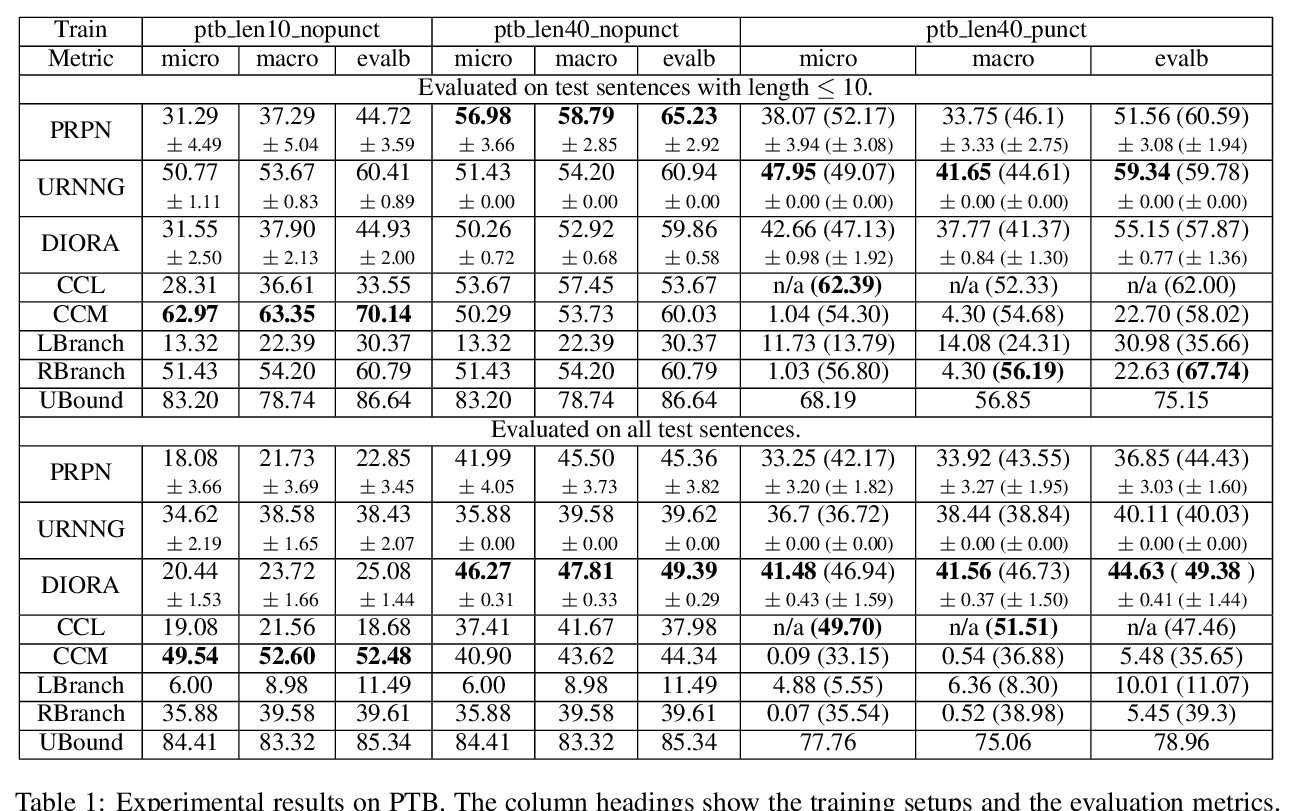
Learning to Segment Actions from Observation and Narration
Daniel Fried, Jean-Baptiste Alayrac, Phil Blunsom, Chris Dyer, Stephen Clark, Aida Nematzadeh,

Zero-shot Text Classification via Reinforced Self-training
Zhiquan Ye, Yuxia Geng, Jiaoyan Chen, Jingmin Chen, Xiaoxiao Xu, Suhang Zheng, Feng Wang, Jun Zhang, Huajun Chen,
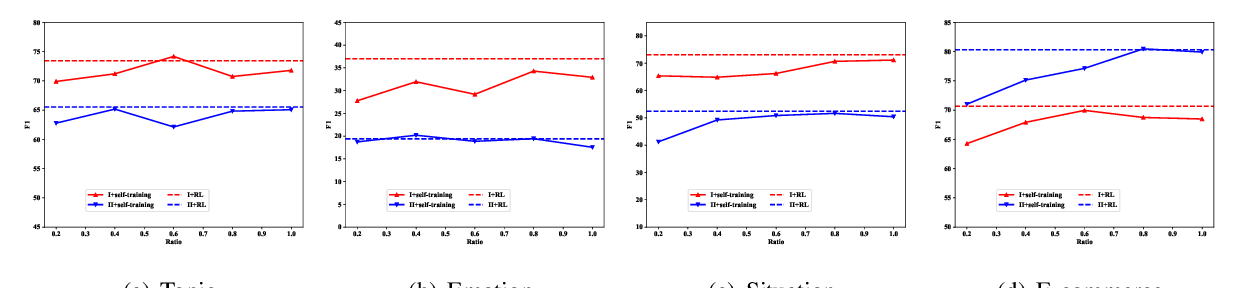